AI Assistants, Copilots, and Agents in Data & Analytics: What's the Difference?
Finding the Right Level of AI Automation for Your Organization.
%202.png)
Introduction
In the past year, vendors have been integrating AI assistants, copilots, and agents into their tools, particularly in the data and analytics sector. If you have scrolled through LinkedIn (or anywhere, really) long enough, you have likely encountered these terms, often used interchangeably.
If you've found yourself unsure about the exact meanings behind these terms, you're not alone. However, as you consider bringing these AI-powered systems into your organization, it's essential to have a clear understanding of their distinct capabilities and use cases. By taking the time to learn the differences between these three concepts, you'll be better positioned to select the right technology.
I recently came across an interesting mental model by Bain Capital Ventures. They studied the different levels of autonomy in AI-powered tools to understand what distinguishes assistants, copilots, and agents. They found that the key difference lies in a single variable—the level of autonomy. AI assistants have low agency and autonomy, AI agents are proactive and autonomous, and copilots fall somewhere in between. The image below illustrates these differences.
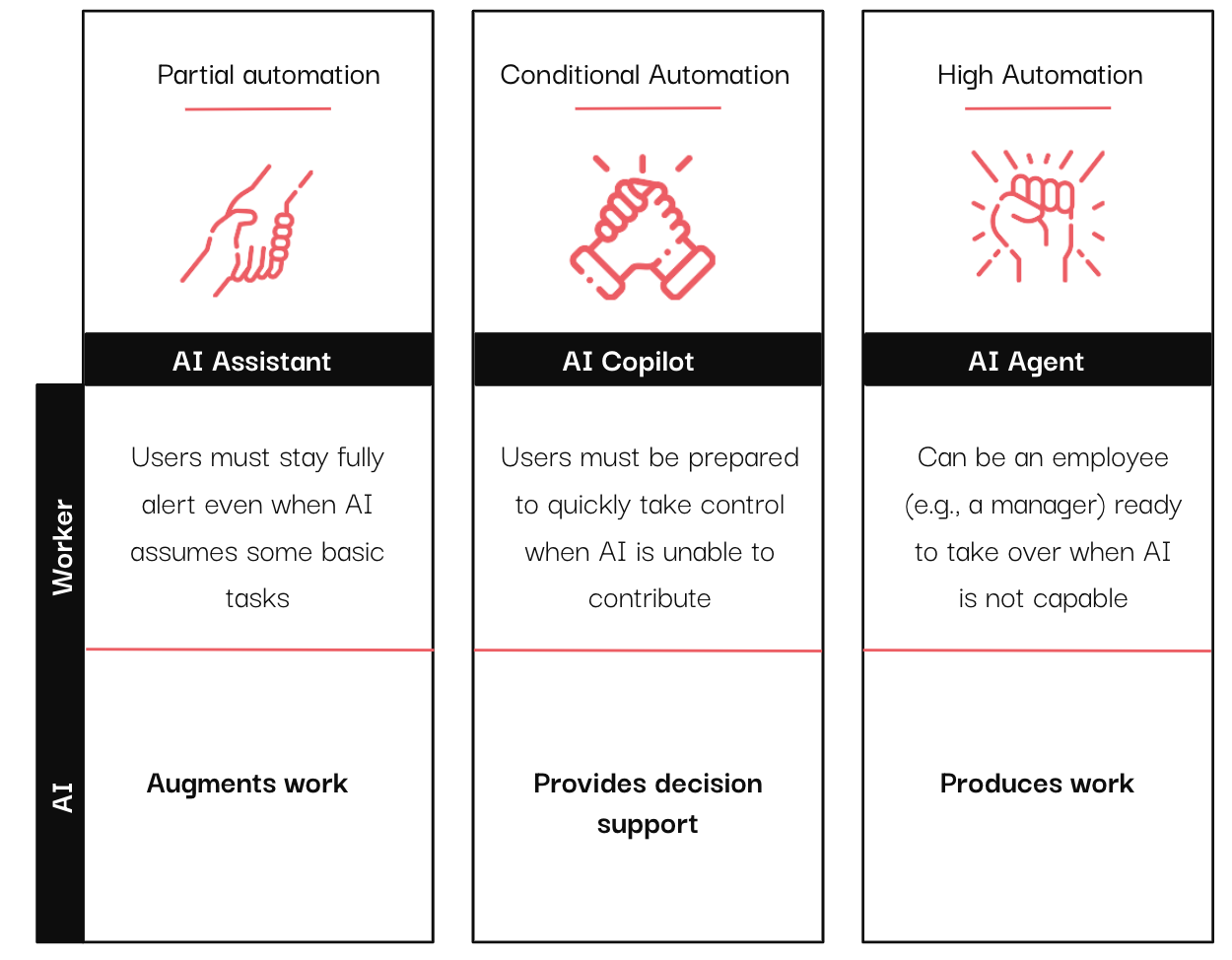
The different levels of autonomy ultimately impact business value, which is the primary concern for most companies. AI systems with low agency are an excellent way to augment work and help stakeholders answer data questions when they already know which questions to ask. However, in many organizations, the majority stakeholders are unsure of which data questions to ask or how to use the answers effectively. This is where companies aim to implement systems with greater agency and autonomy, which can act as prescriptive companions to stakeholders.
In this piece, I will examine the various autonomy levels of assistants, copilots, and agents and how they ultimately affect the business value each can bring to the enterprise. My goal is to help you differentiate between these technologies when implementing them.
I - AI Assistants: Providing Insights & Streamlining Workflows
When you come across the term "AI assistant," think: support function, insights, basic tasks, and low autonomy.
AI assistants are designed to enhance human capabilities across various tasks. They operate based on predefined rules and can quickly provide accurate and relevant information whenever needed.
In the realm of data and analytics, AI assistants operate under a key assumption: the user is well-versed in their domain. To give you a clearer picture, here are some examples of what an AI assistant can do in the data and analytics space:
- Automatically generate documentation for tables and columns within your ecosystem.
- Offer intelligent search capabilities, guiding users towards the most relevant data assets for their needs.
- Translate SQL queries into plain English, enabling less technical users to understand complex queries easily.
- Generate and debug code by formatting SQL queries, improving readability, and optimizing performance, ultimately boosting efficiency and productivity.
An AI assistant can make people who are already involved in analytics work more productive. They streamline the process of finding, understanding, and trusting data. However, it's important to note that an assistant assumes users are already familiar with analytics workflows and can only take on basic tasks.
How to spot an AI assistant from a mile away: they need human oversight, they are far from impacting decisions.
AI Copilots: Elevating Decision Support
Another way to differentiate AI Assistants, Copilots, and Agents is by examining their proximity to decision-making processes. As we've seen, Assistants boost productivity, but they don't directly influence decisions. Copilots, on the other hand, not only enhance productivity but also provide valuable support in the decision-making process.
The term "Copilot" implies the presence of a pilot in charge, someone who determines the direction by asking the right questions. It suggests a collaborative relationship between stakeholders and the machine, which is precisely what it achieves.
So, what can you anticipate from an AI copilot in the analytics domain?
- Natural language queries: AI copilots enable users to ask questions about their data using everyday language. The AI then offers recommendations and suggestions based on the user's query, empowering non-technical users to interact with and extract insights from their data effortlessly.
- Data analysis: Copilots assist users in analyzing data by proposing formulas and providing insights and recommendations based on data patterns.
- Data visualization: AI copilots recommend the most suitable types of charts, graphs, and visualizations based on the data and the user's objectives.
- Next-best actions: Drawing from the insights gleaned from the data, AI copilots suggest the most effective next steps for users to take. For instance, in a customer churn analysis, the AI may propose specific retention strategies for customers identified as high-risk.
Copilots represent a more advanced stage of evolution, as they actively participate in making recommendations, delivering greater business value to users. They empower users to work more efficiently and make better-informed decisions, but they still rely on stakeholders to pose the right questions and determine the desired analyses.
How to recognize an AI copilot from a mile away: They enhance decision-making processes through recommendations and work collaboratively with humans.
AI Agents: Autonomous Decision-Makers
"AI agents are autonomous or semiautonomous software entities that use AI techniques to perceive, make decisions, take actions and achieve goals in their digital or physical environments." Gartner
AI agents represent the most autonomous form of AI. Unlike AI assistants and copilots, AI agents can operate independently, make decisions based on the data they process, and learn from their experiences.
What sets AI agents apart is their level of autonomy. They can perceive their environment through sensors and act upon it through actuators, enabling them to work towards achieving specific goals without human supervision.

The concept of an agent in the context of language models involves enabling the model to break down a complex task into smaller, more manageable steps and then execute those steps sequentially. A large breakthrough in this area was the introduction of "Chain of Thought Prompting". This approach allows language models to decompose multi-step problems into intermediate stages, mimicking a "thinking process" to solve the problem.
So, what can you expect from an AI agent in the Data & Analytics space? Here are some key capabilities.
- Data analysis and decision-making: AI agents can analyze large amounts of data, identify patterns, and make informed decisions aligned with specific goals. They can apply machine learning techniques to extract insights from data, supporting and enhancing decision-making processes.
- Information processing: AI agents excel at processing information in various ways to enhance decision-making and understanding.
- Optimization: AI agents can employ optimization techniques to maximize benefits while managing trade-offs and constraints. They can generate executable action plans to optimize analytical processes and improve efficiency.
- Collaboration: Multiple AI agents can work together on complex analytical tasks that surpass the capabilities of individual agents. The combined application of multiple agents can provide more adaptable, scalable, and robust analytical solutions, particularly in environments that require decentralized decision-making based on data from various sources.
AI agents are the systems that impact business value the most. In fact, the adoption of analytics in enterprises has remained stagnant for 20 years, hovering between 20% and 30%. A staggering 70% of people in organizations do not know what questions to ask or what to do with the answers. AI agents are the systems closest to influencing decision making. They are autonomous enough to define goals, analyze their environment, and engage in prescriptive analytics - which is what organizations need the most.
Decision-making has the most significant impact on a business's bottom line. While AI assistants help organizations with data-driven decision making, AI agents take it a step further by enabling "data-centric" decision-making. They influence the decision-making process of the 70% of people in companies who were previously not involved in analytics.
How to recognize an AI agent from a mile away: They can act without the supervision of a human being, they can take decisions and act on them - affecting their environment.
Conclusion
The terms AI assistant, AI copilot, and AI agent are often used interchangeably, which can lead to confusion. However, the difference between them is quite straightforward. They all fall along the autonomy spectrum, each with a distinct level of independence and impact on decision-making.
At one end of the spectrum, AI assistants augment work, providing valuable support without any autonomy. They don't directly influence decision-making but rather serve as helpful tools to enhance productivity.
Moving along the spectrum, we find AI copilots, which have a higher level of autonomy. They can collaborate with stakeholders and offer recommendations to guide decision-making, acting as trusted advisors in the process.

At the far end of the spectrum, AI agents are fully autonomous entities capable of prescribing the best course of action based on their analysis of the environment and goals.
As a buyer, it's important to assess the optimal degree of automation for various roles within your organization. When reviewing potential vendors, request them to explicitly indicate where their technology stands on the autonomy spectrum. This will enable you to grasp the capabilities and constraints of each solution, helping you find the best solution.
Pursuing AI systems with increased autonomy can lead to higher returns on investment and simpler implementation. Nonetheless, this also necessitates a greater level of trust in the AI's capability to accurately understand your use cases.
Sources
- Gartner. "Innovation Guide: AI Agents." [Link]
- Simform. "Types of AI Agents." Simform Blog.
- Towards Data Science. "LLM Agents Intuitively and Exhaustively Explained." Medium.
- Geeks for Geeks. "Agents in Artificial Intelligence." Geeks for Geeks.
- AWS. "What is AI Agents?" AWS.
You might also like
Get in Touch to Learn More
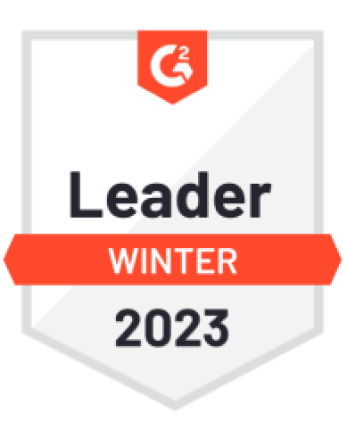
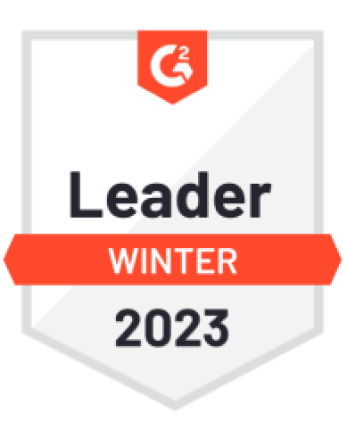

“[I like] The easy to use interface and the speed of finding the relevant assets that you're looking for in your database. I also really enjoy the score given to each table, [which] lets you prioritize the results of your queries by how often certain data is used.” - Michal P., Head of Data