The Data Experience is Broken
Why we need to change the way people interact with data.

Introduction
For the past 15 years, we’ve made tremendous innovations in terms of data production. We’ve optimized the way to store and process data with cloud data warehouses. We can model and transform data easily with dbt. We can create dashboards by clicking fingers thanks to great BI tools.
In terms of collecting, storing, and transforming data, we can now achieve things that were impossible or too expensive 20 years ago. And that’s something to be proud of. But as you can guess, this article is not about praising innovations in data infrastructure. When looking at the data infra landscape, it’s hard not to note the innovation discrepancy between the data production and the “data interaction” realms. It’s hard not to realize how little effort has been put to improve the way people interact with data, or what we call “the data experience”, compared to how much we’ve done to optimize data processes in general.
This article is about the data experience, how we’ve underinvested in it, and what can be done to improve it. The idea is close to the “data as a product” idea popularised by the data mesh trend, but we thought the data experience to be a more tangible idea, with a clear path to ameliorate it. Of course, the two ideas are tightly linked, and ameliorating the data experience does go through treating data as a product. We just want to look at things in the right order. Data experience is the why, while data as a product is the how.
The modern data stack is fragmented
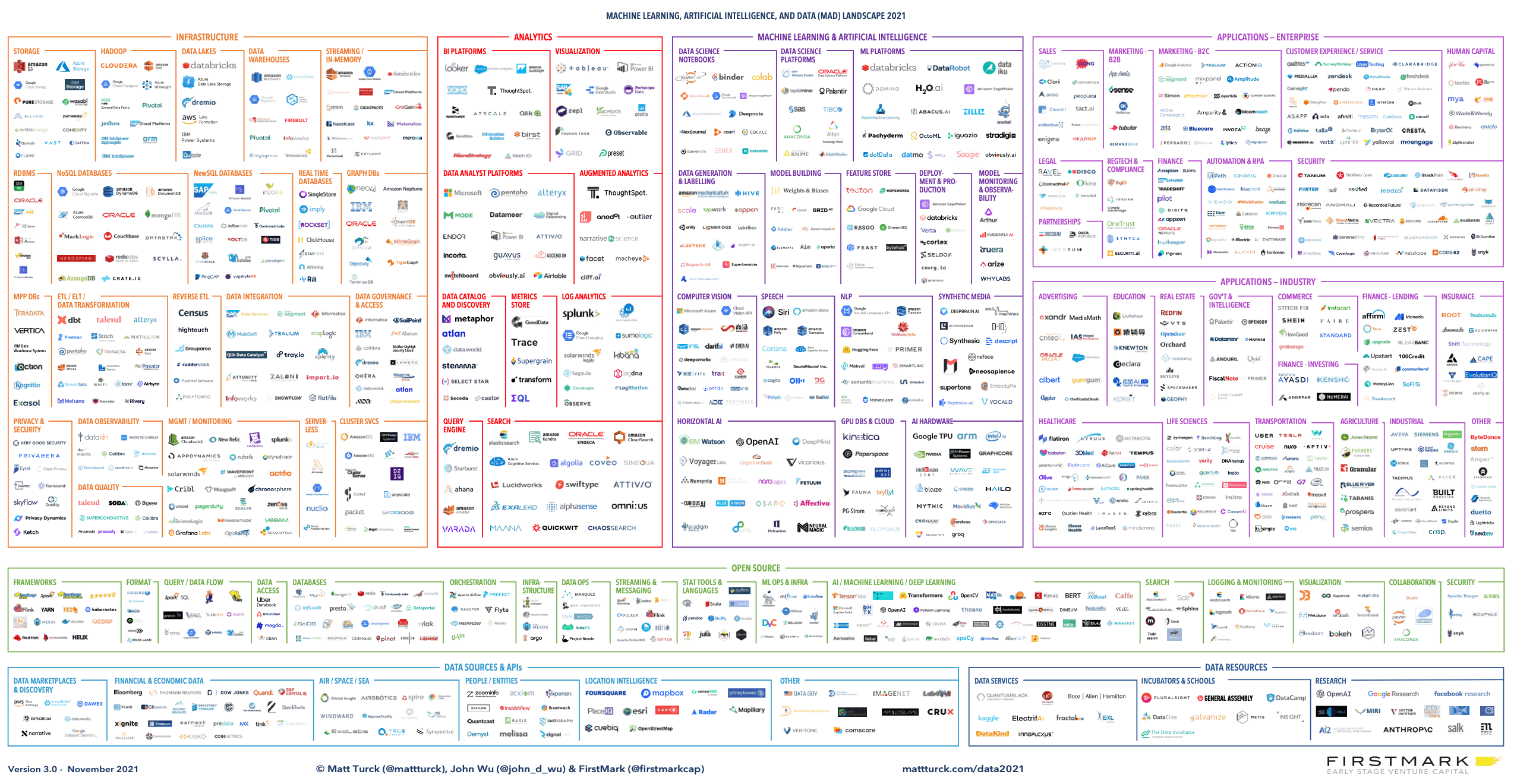
The modern data stack has emerged as our best solution to process volumes of data unseen before. Tools of the modern data stack are individually trying to solve for speed and scalability. Fivetran and Airbyte allow for data ingestion automation, Dbt enables analysts to perform quick and easy transformations. So yeah, individually, tools at each layer of the data stack automate a lot of data processes, making data teams supposedly more efficient. Paradoxically, these tools collectively create new problems which hinder data teams’ productivity. Sluggishness doesn't come from complex processes anymore but stems from the use of this highly fragmented stack. This lack of unification isn’t surprising. Each tool is trying to solve a specific pain point, without thinking about how they fit in the bigger picture. This creates a lot of issues, the worst probably being a huge amount of duplicated work in data teams.
It only takes a quick look at how metrics and KPIs are dealt with in a modern business to realize the absurdity this fragmented ecosystem brought about. Metrics are the core of every single business. They allow businesses to track and forecast performance. Every endeavor involving data ultimately aims at measuring a KPI. How many daily users do we have? What is our sales forecast for 2022? These are the questions prompting all the fuss around data. In short, we use data to track metric progression. Yet, metrics have to be defined in a different manner and in different languages in each tool of the modern data stack. Today, you define metrics in Dbt, in your BI tools, etc.. of course, each tool is dedicated to a specific persona. So it’s not even the same person defining metrics in each tool. Engineers define metrics in their apps, while data analysts do it in others. This unsurprisingly results in chaos and inconsistencies, ultimately killing a business.
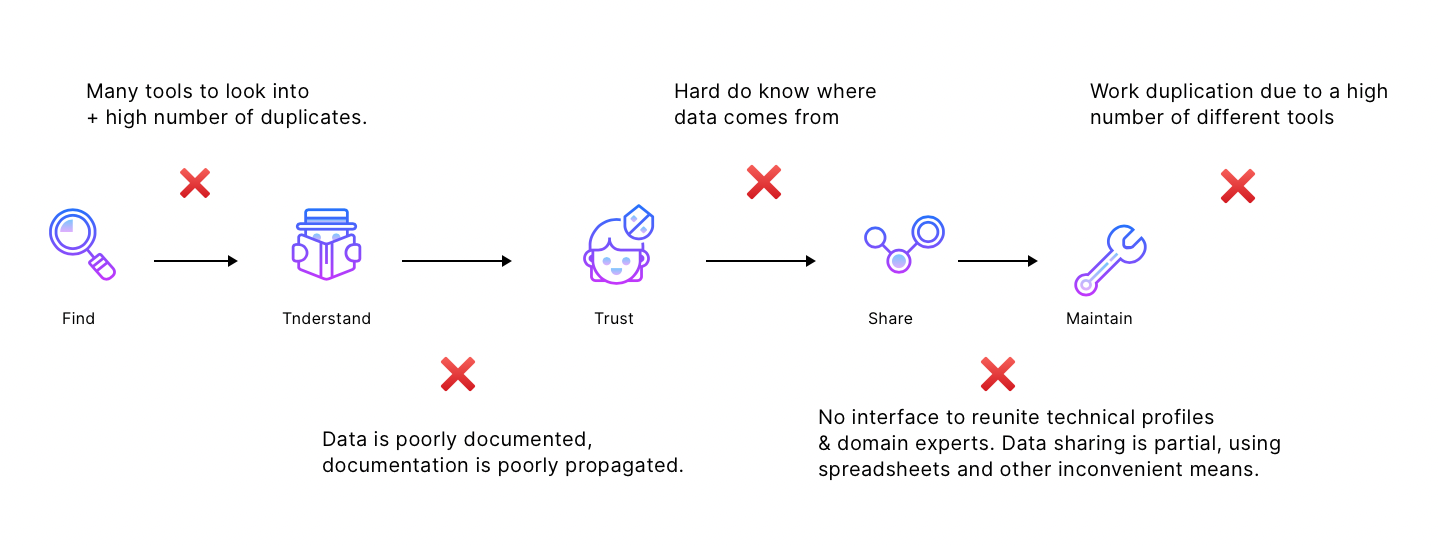
And it goes the same way for metadata. Organizations spend precious hours collecting metadata for their data assets. But the metadata collected in one tool doesn't propagate to others. This means data people have to switch tools to access the needed information, or re-do the work themselves. In short, data people are not having a good time. We’ve fragmented the big data problem into a lot of small different problems, but solving these subproblems doesn’t help with the bigger one.
We’re still failing at leveraging collaboration in data teams
It is commonly said that two heads are better than one, yet this view is far from being reflected in data teams. And it’s a shame because it might well be the thing allowing us to unlock new productivity levels in data teams. The data ecosystem has evolved extremely fast in the past few years. The large volumes of data and the tight regulations around it make it impossible to keep going without a greater level of collaboration. The lack of collaboration in data teams is creating important frictions in data people’s workflows on two levels notably:
- Getting access to data.
This is a new problem. Tight regulations such as GDPR or HIPAA require data access to be firmly controlled. Back in the day, the data steward would ensure this control, granting access to datasets to the right protagonists only. With the data volumes we’re now dealing with, controlling access to datasets while ensuring the availability of data would take a team of 10 people. People don’t hire a full team or data stewards though (or have I missed something?). This results in an overwhelmed data steward, and data people having to wait hours until they can finally be given access to a dataset. This completely contradicts the “self-serve” analytics nirvana everyone is trying to reach.
The process of granting and removing access to data should be made a collaborative one, ensuring anyone needing a data asset can use it right away while respecting compliance with regulatory frameworks.
- Understanding and using data.
The explosion of data volumes has also made our way to deal with metadata management outdated, further complicating data people’s workflows. before the cloud era, the data steward was in charge of metadata management, ensuring all data assets were properly documented. This ensured that those who consumed these data assets would understand them. The issue is, this too can’t be a one-man job anymore. There is simply too much data for all documentation to be handled by one person. Plus, data is alive, and documentation should be too. Automating data documentation has solved part of the problem. The other part will only be solved by leveraging collaboration. Collaboration in data teams, on the model of Github or notion, allows data customers to benefit from each others’ insights regarding a data asset. For example, employees can flag or upvote definitions to notify the dataset owner that they need rework. People can define KPIs, debate definitions, and get quick answers to their “why?” questions in the chat section attached. Our data tools should propose a feature allowing data people to build upon work that has already been done and re-use work to create business value right away.
Conclusion
The data experience is broken, and we shouldn’t find it too surprising. There’s been so much change in the manner in which we collect, store and process data that we have to throw away our previous best practices. Not changing these practices will be highly detrimental to the efficiency of data teams. Secondly, the modern data stack, which is the set of tooling we’re using to process this data is highly fragmented, causing data consumers’ workflows to be even more complex.
There’s no straightforward solution to a broken data experience, and these problems can’t be fixed with a magic wand. We need to learn to think about data users as customers whose experiences matters and should continuously be improved. We should be seeking to consolidate this customer experience rather than trying to fit new tools into these customers’ hands, hoping to make them more productive.
Subscribe to the Castor Blog
About us
We write about all the processes involved when leveraging data assets: from the modern data stack to data teams composition, to data governance. Our blog covers the technical and the less technical aspects of creating tangible value from data.
At Castor, we are building a data documentation tool for the Notion, Figma, Slack generation. We designed our catalog software to be easy to use, delightful and friendly.
Want to check it out? Reach out to us and we will show you a demo.
You might also like
Get in Touch to Learn More
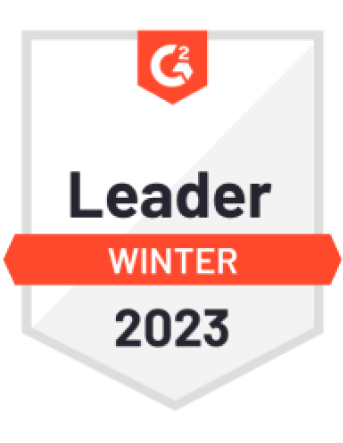
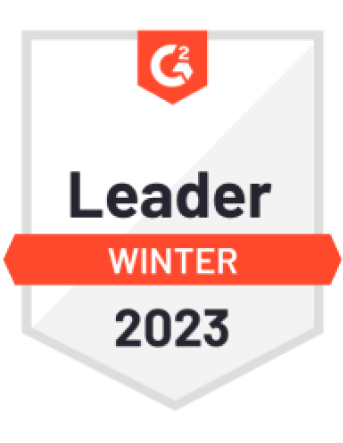
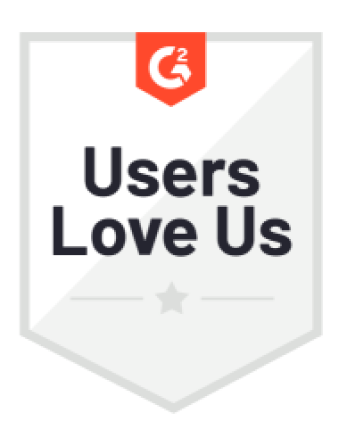
“[I like] The easy to use interface and the speed of finding the relevant assets that you're looking for in your database. I also really enjoy the score given to each table, [which] lets you prioritize the results of your queries by how often certain data is used.” - Michal P., Head of Data