What is AI Analytics?
Harnessing AI for Smarter Data Analysis: A Guide to Implementation and Future Trends
%202.png)
Introduction
If you ask 10 different experts for a definition of AI, you'll get 10 different answers. For the purpose of this article, let's use a simple definition:
AI is the "science of making machines smart." Demis Hassabis, CEO of DeepMind
Similarly, AI Analytics means making your data smart. Compared to traditional analytics, AI analytics speeds and improves the data analysis process, promising to transform how you use your data.
Instead of relying on pixel perfect dashboards curated by a team of data experts, companies leading their industries are using AI analytics to go further and empower everyone with data-driven decision making. Doing so, however, requires understanding the potential of this technology, how it works, and potential benefits and use cases.
But what exactly is AI analytics, and why is it important? We'll delve into its evolution, its distinct advantages over traditional methods, and why it has become indispensable for businesses. We'll also have a look at the key challenges surrounding AI Analytics.
I. A Paradigm Shift in Data Analysis: from Traditional Analytics to AI Analytics
AI analytics is changing how companies use and understand their data. Think of it like solving a big jigsaw puzzle. Traditional analytics is like doing it by hand - it takes ages and you might miss stuff. Now imagine having a super-smart robot helper. This robot can look at all the pieces at once, spot patterns quickly, and even guess what the picture will be. That's what AI analytics does for your data.
"AI analytics uses machine learning algorithms to constantly monitor and analyze huge amounts of data, automating the time-consuming work normally done by a data analyst” Anodot
By mixing AI with regular data analysis, AI analytics helps businesses make better decisions faster. It's becoming a big deal:
- AI investments have doubled in a year.
- Half of all organizations use AI in some part of their business.
- Almost 80% of corporate strategists see AI and analytics as crucial for their success.
However, this current state of AI adoption in the analytics space is the result of a long evolutionary process over 5 generations.
The Evolution of Analytics

Analytics have not always looked as they do today and have come a long way. In the early days, a skilled data team managed complex technologies that delivered spreadsheets:
- 1960s-1980s: Manual data collection and basic spreadsheets - similar to using a notepad and pencil to keep track of your expenses.
- 1990s: Data warehousing and OLAP tools for complex analysis - similar to upgrading to a filing cabinet to organize your paperwork.
- 2000s: Advanced analytics, data mining, and self-service BI - similar to using a calculator and color-coded spreadsheets to analyze your finances.
- 2010s: Big data technologies and real-time analytics - similar to switching to a powerful computer that can instantly process large amounts of data.
- 2020s: AI and machine learning with predictive analytics, natural language processing and automation - similar to having a smart assistant that predicts future expenses and automates your budgeting.
Today, organizations can serve all users and use cases with the right experiences, and with large language models like OpenAI’s ChatGPT, the possibilities are limitless. As we progress further into the 2020s, adopting AI analytics isn't just about having new technology—it's essential for staying competitive.
"Leveraging analytics and AI for more efficient, insightful strategy decisions is one of the biggest challenges, and opportunities, corporate strategists face this year." David Akers, Director of Research at Gartner.
Companies that don't adapt risk falling behind AI-enabled competitors.
II - AI Analytics: The GPS for Modern Businesses
AI analytics is like a modern-day "GPS for businesses," guiding companies through their data to make smarter decisions, improve efficiency, and predict future trends. It's making data tools available to everyone in a company, not just tech experts. This means teams can adapt quickly to market changes. However, just like with GPS technology, the benefits come with risks such as data privacy concerns and the potential for over-reliance.
Better Decision-Making
AI analytics quickly analyzes vast amounts of data to uncover where to make the right turn or to avoid unpractical routes. AI Analytics helps businesses make better decisions. It can uncover trends and patterns that humans might miss, allowing companies to act on opportunities and avoid risks more effectively.
Higher Efficiency and Productivity
AI analytics boosts efficiency and productivity by automating routine tasks. This frees up employees to focus on more important work. Automation reduces costs, minimizes errors, and streamlines data management.
Improved Predictive Capabilities
AI analytics can forecast market trends, understand customer behavior, and identify potential risks before they materialize. This foresight helps companies plan ahead and stay ahead in the market.
Industry-Wide Impact
AI analytics is transforming various industries. Whether you’re in retail to banking to healthcare, you could already be benefitting from AI analytics. In healthcare, it's helping detect diseases early and create personalized treatment plans. Retailers are using it to manage inventory better and give customers tailored recommendations. Financial services are using AI for real-time fraud detection and automated trading.
Challenges and Considerations
However, AI analytics comes with its own set of challenges. Companies need to deal with data quality, privacy, and ethical issues. There's a risk of reinforcing biases in the data, which can be a big problem in areas like hiring or lending. Handling large amounts of data also raises privacy concerns, especially with sensitive personal information.
Implementing AI analytics often requires new skills. Companies might need to train their staff or hire experts in data science, machine learning, and AI ethics. Integrating AI with existing systems can also be tricky and expensive, especially for businesses with older technology.
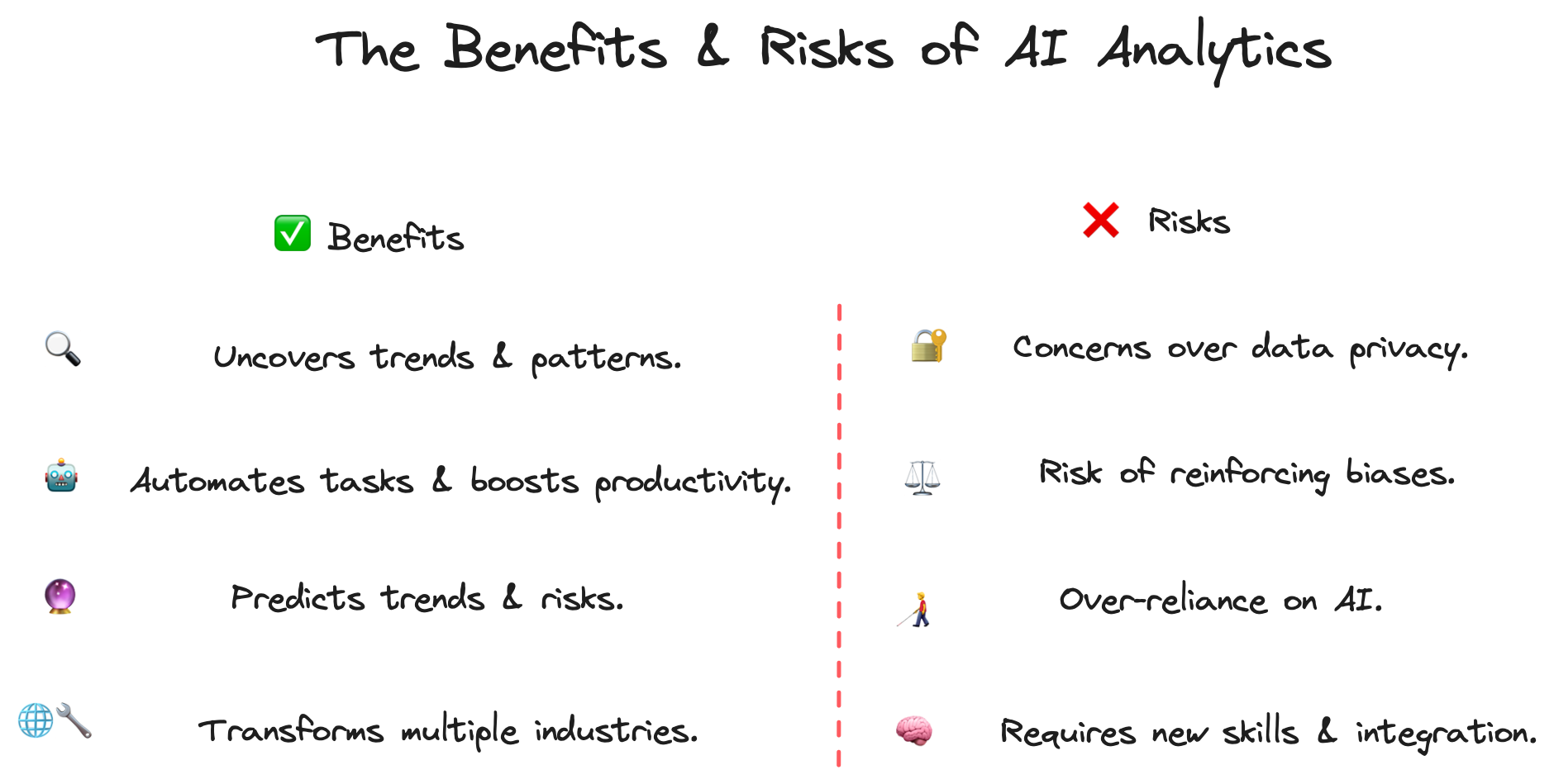
Despite these challenges, the potential of AI analytics to transform business operations and decision-making is huge. As companies embrace this powerful tool, it's crucial to foster a culture of data literacy and responsible use.
The real challenge now isn't whether to use AI analytics, but how to implement it effectively and responsibly, ensuring that it serves as a reliable "GPS" guiding businesses to better and data driven decision-making.
III - How can companies implement AI Analytics?
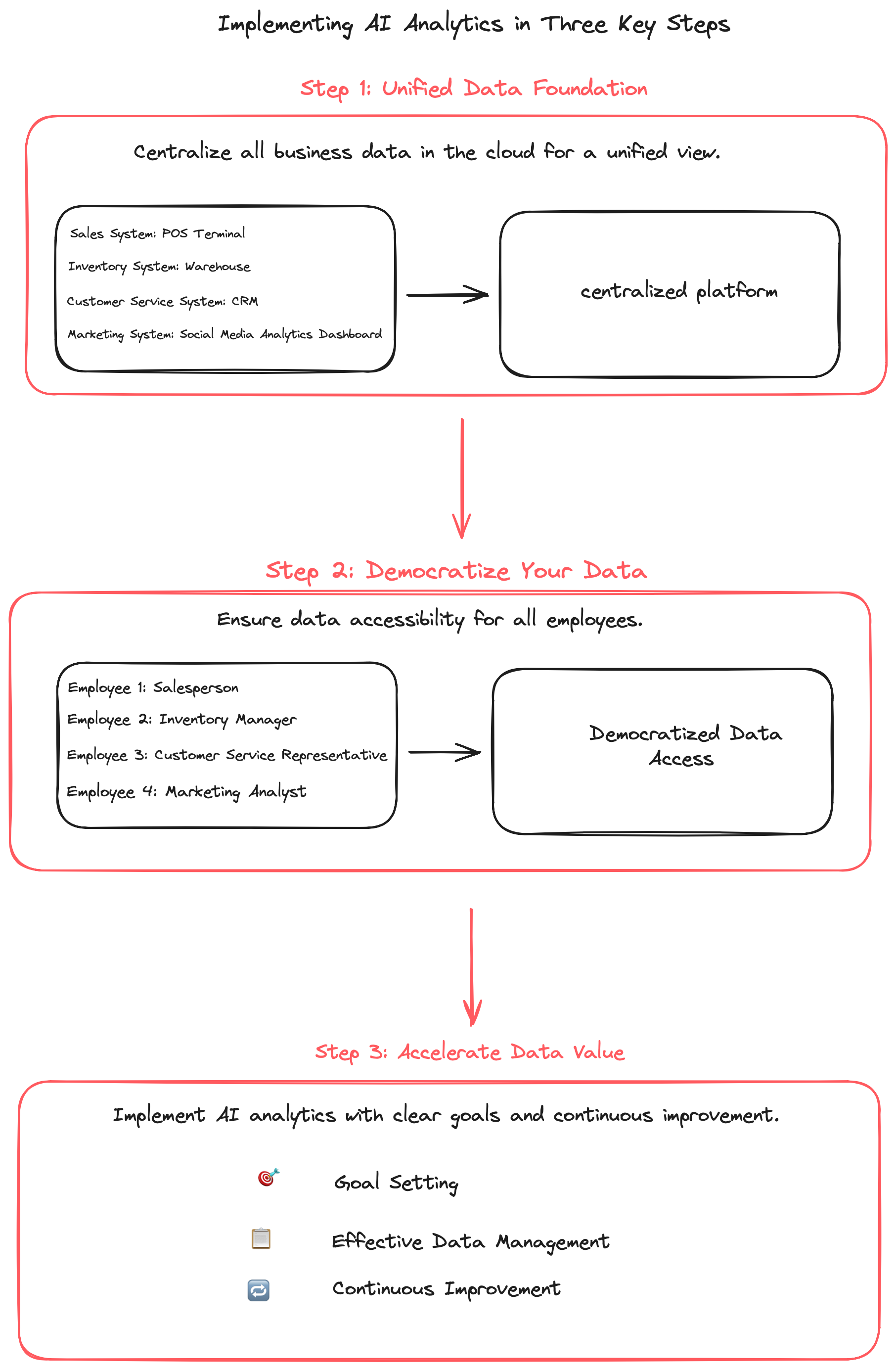
How can you effectively use AI Analytics while managing its challenges? We’ve identified 3 key steps:
Step 1: Create a Unified Data Foundation in the Cloud
First, companies need to bring all their data together in one place, usually in the cloud. This means combining data from different systems, like sales, inventory, customer service, and marketing, into a single, centralized platform.
Building a unified data foundation is crucial for enabling true self-service analytics. By centralizing data, companies can create a "buffet" of high-quality, trusted analyses that users can easily access.
Imagine a big retail company with different departments:
- Sales uses a point-of-sale system.
- Inventory uses a warehouse management system.
- Customer service uses a CRM system.
- Marketing uses social media analytics tools.
Traditionally, these systems work separately, making it hard to get an overall view of the business. But with a unified data foundation in the cloud, all this data comes together in one place, transformed into a consistent format. Now, anyone in the company can access this unified data, making it easier to run analytics and AI models on it.
A unified data foundation enables companies to leverage generative AI for automating routine data tasks and unlocking new data capabilities.This centralized approach helps companies manage data better, making it easier to make informed decisions and stay competitive.
Step 2: Responsibly Democratize Your Data
Once the data is unified, the next step is to make it accessible to the right people. This means ensuring that employees can easily access and use the data they need, even if they’re not tech experts. By having open data policies and sharing agreements, companies can empower more employees to use AI and analytics to gain insights.
While democratizing data access is important, companies must be cautious to avoid the self-service paradox. Striking a balance between empowering users and maintaining governance is crucial.
To address this challenge, companies can implement AI-powered tools that help users navigate the data landscape. AI can act as a bridge, translating business questions into data language and guiding users to the most relevant, high-quality insights.
Step 3: Accelerate Data Value Creation
Finally, companies need a structured approach to implementing AI analytics. This includes setting clear business goals, managing data effectively, and continuously improving their processes.
To accelerate data value creation, companies should focus on preparing their technology, data, and guidelines for AI integration. This includes evaluating options for using pre-trained models, ensuring data readiness, and establishing robust AI usage policies.
Additionally, companies should invest in data and AI literacy programs to empower employees. Developing comprehensive training on AI principles and data management builds upon existing data literacy and enables employees to maximize the value of AI analytics.
As we move further into the AI era, the companies that embrace these principles will thrive.
IV - What’s Next for AI Analytics?
Looking ahead, AI analytics will continue to shape industries and change how we work. Here are some key developments to watch for:
- Better Predictions: AI will get even better at predicting future trends.
- Integration with Blockchain and IoT: Think smart cities using IoT sensors for real-time monitoring, AI for predictions, and blockchain for secure data.
- Wider Access to AI Tools: More people in companies will use AI for innovation, thanks to easy-to-use, low-code, and no-code platforms.
This wider access will be a game-changer, allowing non-tech people to create and use AI models.
But this future has challenges too. Ethical issues, especially around privacy and bias, need strong governance and ethical rules to ensure responsible AI use. Companies that bring their data together and make it accessible now will be ready to take advantage of new AI opportunities and stay competitive.
By embracing these advancements and addressing potential challenges, businesses can fully harness AI analytics to drive innovation.
You might also like
Get in Touch to Learn More
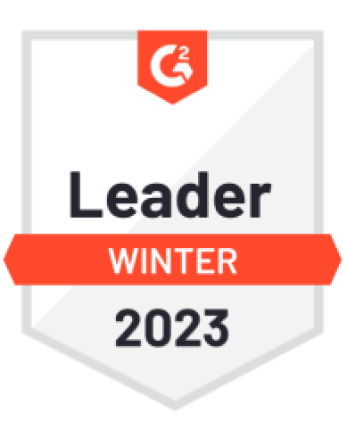
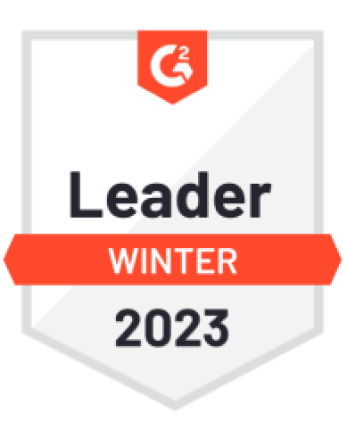

“[I like] The easy to use interface and the speed of finding the relevant assets that you're looking for in your database. I also really enjoy the score given to each table, [which] lets you prioritize the results of your queries by how often certain data is used.” - Michal P., Head of Data