Fluid Truck Builds a Scalable Data Structure With CastorDoc
How we use CastorDoc to supercharge our decentralization efforts
.png)
This customer story was contributed by Connor Swatling, the Head of Business Intelligence at Fluid Truck. Fluid Truck is technology driven commercial fleet rental company that enables small businesses to access a fleet of vehicles at the push of a button in any city across the US.
Introduction
In my role as a head of BI, I oversee the department that focuses on delivering actionable insights through enterprise reporting and forecasts.
Our objective at Fluid Truck is to ensure our customers, primarily renters, enjoy straightforward access to vehicles on our platform. Additionally, we leverage data internally to offer a holistic perspective on fleet management with the goal of boosting operational efficiency.
The way we achieve this goal is to continue to build a self-serve platform and entertain a decentralized data model.
In 2022, we partnered with CastorDoc to support our decentralization efforts. CastorDoc played a valuable role in helping us de-clutter the data warehouse, making it much easier for stakeholders to navigate.
I - The challenge: centralized models don’t scale
“The quantity of data we needed to manage […] was overwhelmingly large, beyond what the team could comfortably handle. These data volumes made it very hard for understanding the potential downstream implications of any changes we made.” -Connor Swatling, Head of Business Intelligence, Fluid Truck
Fluid Truck has experienced significant growth over the past five years, expanding from a team of 10 individuals in 2017 to over 400 employees. When I joined the company in 2021, the business intelligence group was tasked with migrating our analytics database from legacy systems to Google Cloud Platform (GCP).
Additionally, we were responsible for generating ad hoc reports for senior leadership. During this period, our reporting process was centralized and primarily transactional in nature. Stakeholders would make requests, and the team would work diligently to fulfill those requests.
However, as our organization continued to grow, new departments emerged and sought to scale their operations. Simultaneously, our product development team was launching new features. These developments resulted in an influx of new tools and widgets aimed at facilitating our customers' acquisition of fleet vehicles for their small businesses. Consequently, we faced the challenge of building a scalable ecosystem while simultaneously providing timely reporting insights.
Although we significantly expanded the size of our Business Intelligence team, we came to the realization that the existing ticket-based request system no longer met our needs.
As our organization scaled, it became increasingly unfeasible for the BI team to amass enough domain knowledge to address the influx of tickets effectively.
Additionally, the quantity of data we needed to manage across our assets, tables, and dashboards was overwhelmingly large, beyond what the team could comfortably handle. These data volumes made it very hard for understanding the potential downstream implications of any changes we made.
II - The Solution: People, Processes, Tools
“CastorDoc met our needs right at the gate, without requiring a lengthy setup process or extensive calls. The setup was instantaneous and didn't demand a substantial initial investment or effort.” - Connor Swatling, Head of Business Intelligence, Fluid Truck
Our central Business Intelligence team couldn't master all the different areas, so we needed experts in those areas to get good at using data. We started a program to teach domain experts to become data champions.
This is a lot like the movie Armageddon. In the movie, an asteroid is about to hit Earth, and they need to blow it up. Instead of teaching astronauts to drill, they teach drillers to become astronauts.
The analogy is a fitting one for our data champion program: Instead of turning our Business Intelligence team into jacks of all trades, we're teaching domain experts how to better understand and utilize data, much like training drillers to be astronauts.
These individuals act as the catalysts for fostering a data-driven culture within the organization, bridging the gap between the Business Intelligence team and the wider business operations.
Yet, as we continued to grow and our data landscape became more complex, we needed a more robust solution to manage and navigate our data. This is when we started looking for a data cataloging tool.
Our requirements were clear. We needed a tool that could enhance our visibility into the data warehouse, given the large data volumes. Additionally, the tool had to feature robust and user-friendly data lineage capabilities, enabling us to trace the dependencies between data assets. This understanding would help us foresee the potential impacts of any changes made by the data team. Lastly, it was crucial that the tool could seamlessly integrate with our GCP data environment.
CastorDoc emerged as our top choice as it fulfilled all these prerequisites. What’s more, CastorDoc met our needs right at the gate, without requiring a lengthy setup process or extensive calls. We appreciated the efficiency of communicating via Slack. The setup was instantaneous and didn't demand a substantial initial investment or effort. This was crucial for us, as we needed a solution that could be implemented swiftly.
III - The impact: A de-cluttered environment
With CastorDoc, we've managed to achieve some impressive clean-up of our data clutter. We had a lot of objects in our data warehouse that were essentially redundant, including tables that were created years ago and no longer served any purpose.
These unused tables were creating confusion as our growing team of analysts began reporting based on these irrelevant resources.
We decided to de-clutter our data warehouse, and CastorDoc proved invaluable in this process. It helped us identify 93 objects that were no longer necessary or relevant.
Consequently, we executed a substantial decluttering operation by taking decisive action on 73.9% (69 out of 93) of these obsolete items. These objects included outdated content, such as dashboards that were no longer valid.
Scanning the data warehouse manually to spot these outdated items would have taken us days of work. But CastorDoc was able to do this for us automatically, which saved a lot of time.
This de-cluttering effort meant that anyone navigating through our data warehouse would encounter only relevant, useful information. This ensured we had a strong and reliable basis for reporting.
This clean-up has had a significant impact on productivity - working in a streamlined ecosystem saves so much time and energy. Plus, our team no longer worries that what they’re building might be flawed or useless. It creates a higher degree of trust in our data and our systems.
In our long-term strategic plan, we envision CastorDoc playing a more central role within our data team. We plan to integrate with the CastorDoc API, and to open up access to CastorDoc to more stakeholders in the company.
By doing so, we hope to foster a more efficient and dynamic data management process, improving response times and the overall quality of decision-making.
Subscribe to the newsletter
About us
We write about all the processes involved when leveraging data assets: from the modern data stack to data teams composition, to data governance. Our blog covers the technical and the less technical aspects of creating tangible value from data.
At Castor, we are building a data documentation tool for the Notion, Figma, Slack generation.
Or data-wise for the Fivetran, Looker, Snowflake, DBT aficionados. We designed our catalog software to be easy to use, delightful and friendly.
Want to check it out? Reach out to us and we will show you a demo.
You might also like
Contactez-nous pour en savoir plus
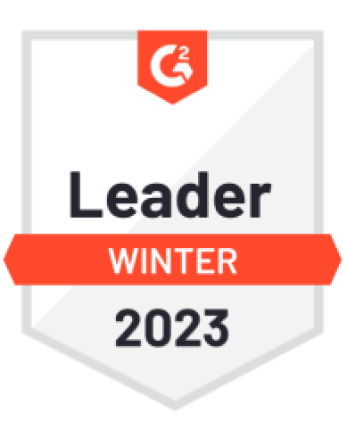
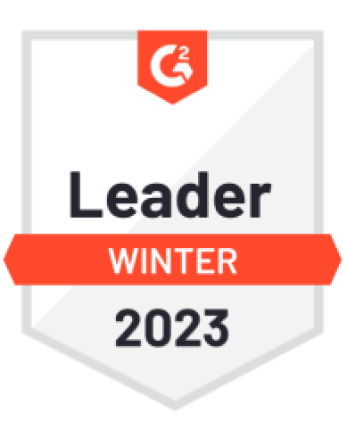

« J'aime l'interface facile à utiliser et la rapidité avec laquelle vous trouvez les actifs pertinents que vous recherchez dans votre base de données. J'apprécie également beaucoup le score attribué à chaque tableau, qui vous permet de hiérarchiser les résultats de vos requêtes en fonction de la fréquence d'utilisation de certaines données. » - Michal P., Head of Data.