Data Mesh ROI - A Primer
A Comprehensive Guide to Data Mesh ROI and Business benefits.

Recently, I read that before starting a long self-improvement journey (a recovery, developing new skills, etc), it's essential to nail down your "why?". Your why is what keeps you going when you feel like giving up, when you don’t have time, when you start making excuses, etc. It reminds you of the reasons why you started the journey in the first place, and helps you keep going.
This approach can also be adapted to companies who are moving towards a data mesh
Implementing a data mesh is an organizational journey, not a personal one. But it’s long and arduous enough that you should have your why pinned down.
Why should you enable self-service? Why should you treat data as a product? Why is it all worth it?
There's plenty of literature on data mesh, yet much of it falls short in precisely articulating the tangible business value derived from transitioning to a data mesh architecture.
"Data mesh enables the construction of more scalable systems."
"Data mesh dismantles existing silos."
"Data mesh eliminates data team bottlenecks."
These answers often pop up when asking, "Why should I move to a data mesh?" And, yes, they're valid. But they're also kind of fuzzy. And fuzzy stuff just doesn't cut it when you're thinking about jumping into a long, transformational journey. It’s not a solid enough “why”.
This article aims to pin down the tangible value of data mesh. To be honest, it's a tough one to write. For most companies that have started their data mesh journey, it's still too soon to see tangible results.
Big names like Hellofresh or Blablacar have shared their data mesh experiences, but their journeys aren't over yet. However, I've managed to compile examples of the tangible business impact data mesh can deliver.
Data mesh forces organizations to think of data from the use case and business value down to the data, not the other way around” - Forrester, ‘Data Mesh in 2023 and Beyond’
There are three aspects of your organization that a data mesh infrastructure should impact: data discovery, operational efficiency, and data quality.
For each aspect, I'll point out the metrics you should expect to see improve after implementing a data mesh. Whenever possible, I'll use real-life company examples or survey findings to give you an idea of what you might expect for your own organization.
Data mesh: a refresher
I assume if you’re reading this you're already somewhat familiar with what a data mesh is. But just to set the context, let's do a quick rundown.
Data mesh is a way of organizing data in a company by breaking it down into smaller, manageable parts called "domains." Each domain represents a specific area or team within the organization. An example of a domain in data mesh could be a "sales domain" that contains data related to sales performance and customer interactions.
Instead of a completely siloed system, data mesh connects these autonomous domains through a universal layer with standard rules, making collaboration smoother. This approach boosts flexibility and scalability in data usage within an organization.
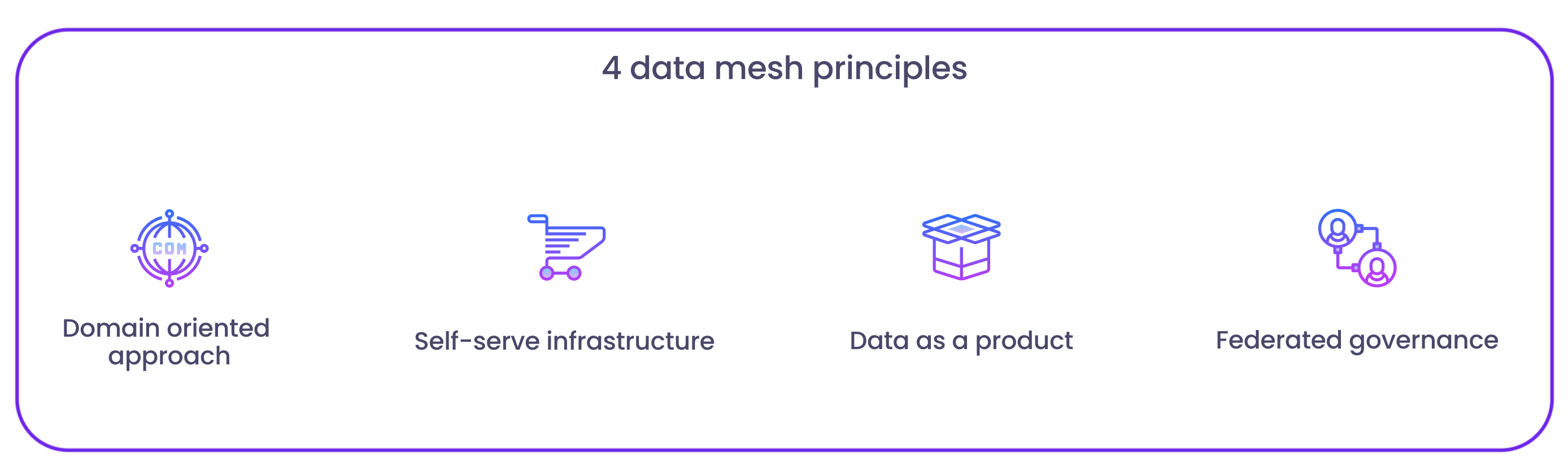
A data mesh architecture revolves around four key principles:
- It adopts a domain-oriented approach, distributing data ownership across specific teams.
- It treats data as a product and assigns ownership to relevant teams.
- It promotes self-serve data infrastructure for easier data product creation.
- It embraces federated data governance for maintaining order and fostering collaboration.
Now that we've got a clearer picture, let's dive into how this infrastructure is meant to impact your business.
I - Enhanced data discovery and usability
By shifting to a data mesh architecture, you can expect stakeholders from all backgrounds to find and use data in a more self-sufficient manner. This should unlock new levels of efficiency and productivity across your organization.
In this section, I explore two key aspects of data discovery and usability that you can measure by implementing a data mesh: a) improved data discoverability, and b) a focus on product thinking for data.

Let’s dive in!
A. Improved data discoverability: Fostering a user-friendly data ecosystem for easier data finding, access, and usage.
A data mesh architecture enhances data discoverability by decentralizing data ownership and promoting a domain-oriented approach.
By assigning responsibility for specific data domains to individual teams, data mesh encourages the development of user-friendly data products, complete with proper documentation.
Data domain teams have superior understanding and expertise in managing their specific datasets, compared to centralized data teams who can be disconnected from business value.
This deep understanding of their data ensures they can provide the relevant context around datasets, making it simpler for users to find, understand and use the data they need.
So, what's the business value of this improved accessibility and discoverability? You can track it using two key metrics: data discovery time and onboarding time, which should both decrease after transitioning to a data mesh infrastructure.
Data discovery time is the time it takes for users to locate the appropriate data for a specific task. With a data mesh, this should be reduced as data is better organized and more easily discoverable. By reducing the time it takes for data professionals to find the right data, the efficiency of data projects can be increased, allowing for more data projects to be completed each year. This, in turn, unlocks incremental business value by enabling more data-driven decisions to be made, such as improving marketing spend efficiency.
Additionally, onboarding time measures the time required for new users to become autonomous in navigating and using the data platform. Enhanced discoverability due to a data mesh structure will also drive onboarding time down. If employees can find and understand the data better, they become autonomous with the data environment much quicker. You can measure this by analyzing user logs, conducting surveys, or using onboarding metrics to track the time it takes for new users to complete certain tasks. The impact of reduced onboarding time can be significant for businesses. Faster onboarding time means that new users can become productive more quickly, allowing them to contribute to data-driven initiatives sooner. This can lead to increased productivity and faster time-to-value.
For instance, when Vestiaire Collective enriched their data with proper documentation and metadata, they experienced a 80% decrease in onboarding time—from roughly two weeks to less than two days. This also eliminated the need to assign a mentor to each new employee to explain how the system works, further streamlining the process and adding efficiency to the mentor’s capacity to work on higher value projects.
B. Enhanced Data Product Quality
The data mesh architecture also puts a strong emphasis on product thinking for data. This means focusing on the user experience throughout the end-to-end data lifecycle.
By treating data as a product, teams are encouraged to prioritize the needs and expectations of their users, ensuring that data products are designed with the end user in mind.
In a data mesh, each team is responsible for their domain's data products, from creation to maintenance.
This sense of ownership drives teams to deliver high-quality data products that cater to the specific needs of their users.
One metric to track the impact of product thinking for data is user satisfaction with data products. This metric measures how happy users are with the data products they access, based on factors such as ease of use, documentation, and relevance.
The increased business value of a data mesh comes from the direct link between user satisfaction and improved decision-making. When users are satisfied with the data products they have access to, they can more easily consume and analyze the data to make informed decisions. As a result, this can lead to increased operational efficiency, improved business performance, and accelerated innovation.
Additionally, the emphasis on high-quality data products in a data mesh infrastructure can help to reduce the time and resources spent on data cleaning and preparation. This, in turn, allows data analysts and scientists to focus on higher-value tasks, such as extracting actionable insights from data, which can directly contribute to the company's bottom line.
For example, Printify saw stakeholders' satisfaction with data documentation increase by 87%, after implementing a data mesh architecture. This showcases the potential of product thinking for data.
But that’s not all, you can also expect your mesh architecture to improve operational efficiency. This will be the focus of the next section.
II- Increased operational efficiency and cost-effectiveness
Organizations constantly strive to boost operational efficiency and cost-effectiveness in their data management processes. Adopting a data mesh architecture leads to significant improvements in these areas, creating a less expensive data environment.
In this section, I delve into two key aspects of operational efficiency and cost-effectiveness that benefit from a data mesh implementation: a) faster time to market for data products, and b) cost savings and resource optimization through reduced bottlenecks.

A. Faster time to market for data products
Implementing a data mesh will usually lead to faster time to market for data products.
Federated governance and crowdsourced documentation means data products can be developed and launched much quicker than when there is a centralized data team in place.
Instead of burdening a single data steward, the responsibility for documentation and data management is distributed among domain-specific teams. This method simplifies development and speeds up data product delivery to users.
Data mesh's impact on time to market can be assessed using two key metrics: data product development time and time to value.
Data product development time tracks the period from when a new data product idea is conceived to when it's released. A decrease in development time signifies a more efficient process, leading to quicker time to market.
Time to value evaluates the duration it takes for a new data product to begin offering value to an organization, such as generating insights or enhancing decision-making. A shorter time to value implies that the organization can reap the benefits of the data product more rapidly.
Upon adopting a data mesh, you can expect to witness improvements in both of these metrics.
B. Cost Savings: eliminating bottlenecks
When adopting a data mesh architecture, reducing bottlenecks can lead to significant cost savings. Traditional data teams are a major source of bottlenecks, and there are two key reasons for this.
First, regulations such as GDPR or CCPA require data access to be tightly controlled. With centralized data teams, stakeholders may have to wait days to access a dataset due to the access granting requirements.
Second, in the traditional structure, data teams are responsible for documentation and maintenance of datasets. Thus, when stakeholders have a question, they typically file a ticket for the data team, which acts as a bottleneck again.
However, in a data mesh architecture, users can access well documented data products through a self-serve infrastructure. This has two benefits. First, it removes the bottleneck of having to go through the data team for data access or questions. But this also frees up the data analytics team to focus on higher value tasks than answering data tickets.
One way to track the impact of a data mesh on resource optimization is to look at the number of tickets filed for the data team. When the number of tickets drops, it means stakeholders have become autonomous with the data.
For example, the data team might get a lot of requests from the marketing team about attribution. In an architecture with self-service and well documented data products, the marketing team should become less dependent on the data team for this kind of issue, ultimately driving the number of attribution-related requests to zero.
For example, Stuart improved documentation of their data products across the company as part of their data mesh journey. This led to a significant reduction in data-related inquiries, which dropped by 50%. This reduction in support tasks allowed the data engineering team to allocate more time to higher-value activities, ultimately contributing to the overall ROI of the data mesh initiative.
III - Improved health: data quality and risk mitigation
Last but not least, you should see data health improve a lot after implementing a data mesh. In this section, I delve into two critical aspects of data quality and health that are positively impacted by adopting a data mesh: a) improved data quality and reliability, and b) risk mitigation through federated governance.

A. Improved data quality and reliability
In a data mesh architecture, domain teams are naturally encouraged to prioritize these aspects, simply because it leads to more accurate business insights and better decision making.
Domain oriented ownership means that each domain-specific team is accountable for producing, maintaining, and sharing high-quality datasets. Distributing data quality responsibility is efficient, because domains teams are close to business impact, and thus more incentivized than centralized teams to maintain good data quality standards.
In a data mesh, federated data governance is used to ensure that data quality standards are maintained across the organization. This approach promotes collaboration and standardization, reducing the risk of data quality issues caused by siloed data management practices.
Consequently, data consumers such as data scientists, analysts, or other data domain teams can trust the data they receive, leading to better decision-making.
In a webinar co-hosted with Monte Carlo, Corentin Limier, a former data engineer at Vestiaire Collective, discussed how implementing federated governance and intelligent ownership allocation fostered greater trust in data at Vestiaire.
To measure the impact of a data mesh on data quality and reliability, you can also monitor the data quality score, an aggregated metric that evaluates data quality across various dimensions, including accuracy, completeness, consistency, and timeliness.
A good data quality score across the board impacts your business across three key dimensions. First, with accurate and reliable data, your organization can make data-driven choices and optimize resource allocation. Additionally, high-quality data reduces time spent on data cleaning, allowing teams to focus on value-added tasks. And finally, consistent and reliable data promotes a data-driven culture, enhancing cross-functional teamwork, and ensuring that organizations meet regulatory requirements; this ultimately minimizes risks associated with inaccurate or incomplete data.
B. Risk mitigation through federated governance
Implementing a data mesh architecture also enables risk mitigation through federated governance.
By decentralizing the governance model, you can enforce data policies and best practices more effectively, mitigating risks associated with data privacy, security, and compliance.
A federated governance model means that each domain-specific team is responsible for data governance in their own area. They can then enforce and monitor compliance with organizational data policies and best practices.
This approach ensures that each team is well-versed in the specific requirements and regulations relevant to their data domain, reducing the risk of non-compliance and potential associated penalties. For example, Google was fined €50 million by France's data protection authority, CNIL, in 2019 for GDPR non-compliance related to transparency and consent for personalized ads. By having teams that are knowledgeable about regulations like GDPR, companies can better avoid such fines and maintain a good reputation.
To gauge the impact of federated governance on risk mitigation, you can track the data governance compliance rate. This metric represents the percentage of data products and processes that adhere to the organization's data governance policies and best practices, including data privacy, security, and ethical guidelines.
Conclusion
In conclusion, the data mesh architecture has the potential to revolutionize the way organizations approach their data ecosystems. By decentralizing data ownership and promoting a domain-oriented approach, data mesh can deliver significant benefits across various aspects of data management, including enhanced data discovery and collaboration, increased operational efficiency, and improved data quality and risk mitigation.
Although data mesh is a relatively new concept, early adopters have already begun to reap some benefits. The primary objective for implementing data mesh should be to unlock the business value it offers. This article aimed to identify the business value associated with data mesh and provide insights on how to effectively measure it.
Subscribe to the newsletter
About us
We write about all the processes involved when leveraging data assets: the modern data stack, data teams composition, and data governance. Our blog covers the technical and the less technical aspects of creating tangible value from data.
At Castor, we are building a data documentation tool for the Notion, Figma, Slack generation.
Or data-wise for the Fivetran, Looker, Snowflake, DBT aficionados. We designed our catalog software to be easy to use, delightful, and friendly.
Want to check it out? Reach out to us and we will show you a demo.
You might also like
Contactez-nous pour en savoir plus
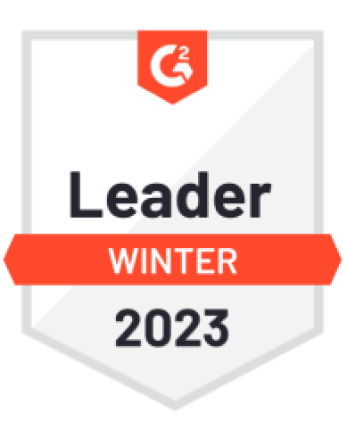
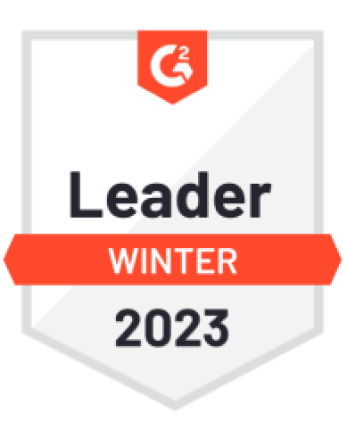

« J'aime l'interface facile à utiliser et la rapidité avec laquelle vous trouvez les actifs pertinents que vous recherchez dans votre base de données. J'apprécie également beaucoup le score attribué à chaque tableau, qui vous permet de hiérarchiser les résultats de vos requêtes en fonction de la fréquence d'utilisation de certaines données. » - Michal P., Head of Data.