What is a Data Glossary?
and what is the difference with data catalogs or data discovery tools?

Introduction
The phrase "data glossary" is becoming more common, but its meaning and purpose aren't always obvious. To make matters more confusing, people often use "data glossary," "data dictionary," and "data catalog" interchangeably. While these ideas are part of the same group, focused on data and metadata management, they have important differences. Knowing how they differ and work together is crucial for effective data management. This article will clarify what a data glossary is and how it connects to the other two concepts mentioned.
What is a data glossary?
A data glossary, sometimes called a "business glossary," is a collection of business terms and their definitions. Its main goal is to establish a shared understanding of concepts within an organization, creating a unified language. The idea of a data glossary is simple but crucial. A well-maintained business glossary ensures everyone in an organization speaks the same language, providing a single source of truth for all business terms.
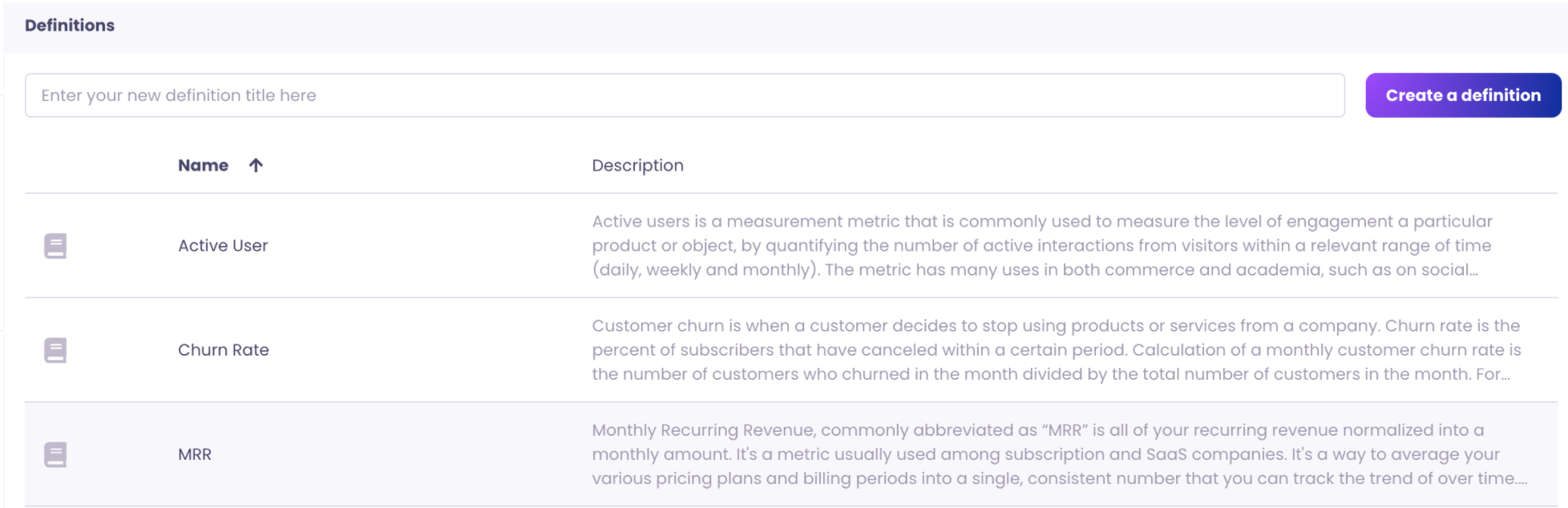
Why is a data glossary useful?
Creating and maintaining a business glossary involves building a repository of terms used in business operations and defining them. While a data glossary is simple to create and maintain, it can significantly impact business performance. In fact, business glossaries can help you:
1. Measure your KPI's
If you don't have a clear repository of business terms and their definitions, it's challenging to measure your KPIs. Different people in your company may come up with varying results for specific metrics, making it difficult to determine the correct numbers. Language is often open to interpretation, leading to misunderstandings about business term meanings.
For example, imagine your team analyzes customer churn and assumes "customers" are users active on your app in the past week. Meanwhile, the marketing team defines customers as people who have made a purchase through the app in the past month. This discrepancy can result in inconsistencies between analyses and dashboards, which can be exposed during presentations to clients or executives.
In short, a business glossary is essential. It's the foundation for calculating your KPIs, a fundamental activity for any organization. Successfully measuring KPIs and understanding growth drivers are the first steps in a successful data journey. Without a data glossary, it's challenging to build the data analysis and data science steps that typically follow.
2. Bring trust to your analyses
If employees in a company don't share a common understanding of business terms, trust can be lost, undermining analysis efforts. If an executive or client receives two conflicting reports about the same term without context, they may lose confidence in both reports, the team that produced them, and the company's data. Hiring a data team to create dashboards and reports is pointless if their work isn't trusted. Identifying the root of these errors can be challenging. With a well-maintained data glossary, users can easily find the meaning of specific terms. This accessibility boosts productivity, efficiency, and trust in company data.
3. Promote data governance
A well-maintained data glossary can serve as the foundation for successful data governance. Establishing clear standards for data terms and definitions enhances organizational knowledge. This organized knowledge repository helps users define rules and maintain access policies using data glossary terms. For instance, assigning security levels to specific business terms allows for defining data governance policies based on these levels. A data glossary can also include data quality rules, alerting users to data quality issues. As a result, a data glossary is a vital component in a data governance initiative.
In summary, building a glossary is a low-effort, high-impact initiative. Data glossaries are valuable to build, maintain, and share within an organization.
Data glossary vs data dictionary: the difference
Although referring to fundamentally different things, the terms 'data glossary' and 'data dictionary' are often used interchangeably. That's why I'd like to bring a bit of clarity on how they differ and how they can be related.
A data glossary is just a bunch of business terms and their definitions. We've covered this. On the flip side, a data dictionary seeks to enrich each database with metadata.
According to the DAMA Dictionary of Data Management, a data dictionary is:
“A place where business and/or technical terms and definitions are stored. Typically, data dictionaries are designed to store a limited set of metadata concentrating on the names and definitions relating to the physical data and related objects.“
It is a collection of information about data terms which can be database schemas, tables, or columns. In data dictionaries, a user will usually find information about:
- Data type
- Size
- Default values
- Constraints
- Relationship to other data
- The meaning/purpose of a given table or column
- Whether the information is PII or not
If you're not sure of the meaning of metadata, and why it's key for your company to collect and exploit it, here's an article that brings clarity on the matter.
Data dictionaries are usually used to provide consistency in data collection, enforce the use of data standards, and show the relationship between data assets.
Data dictionaries cater to technical users, such as data analysts and data scientists. Technical people consult the data dictionary to improve their understanding of the data, allowing them to manage, move, merge and analyze data efficiently. They're also more system-specific.

Data catalog: Tying the data glossary with the data dictionary to leverage your data seamlessly
.png)
We have a third term that's alternatively mixed up with a data dictionary and data glossary: the data catalog. the data catalog is the glue tying the data dictionary and the data glossary together. It's a metadata management solution offering the possibility to store both in a single repository. But why would you want to do so?
Although data dictionaries and business glossaries are different entities, they work as a great tandem that can ultimately produce a single source of truth about your data. Having information about both your data assets and business terms in the same repository opens a myriad of possibilities. For example, a data catalog allows various technical terms of the data dictionary to be tied with a single business concept in the business glossary. This helps conceptualizing technical elements using a business angle.
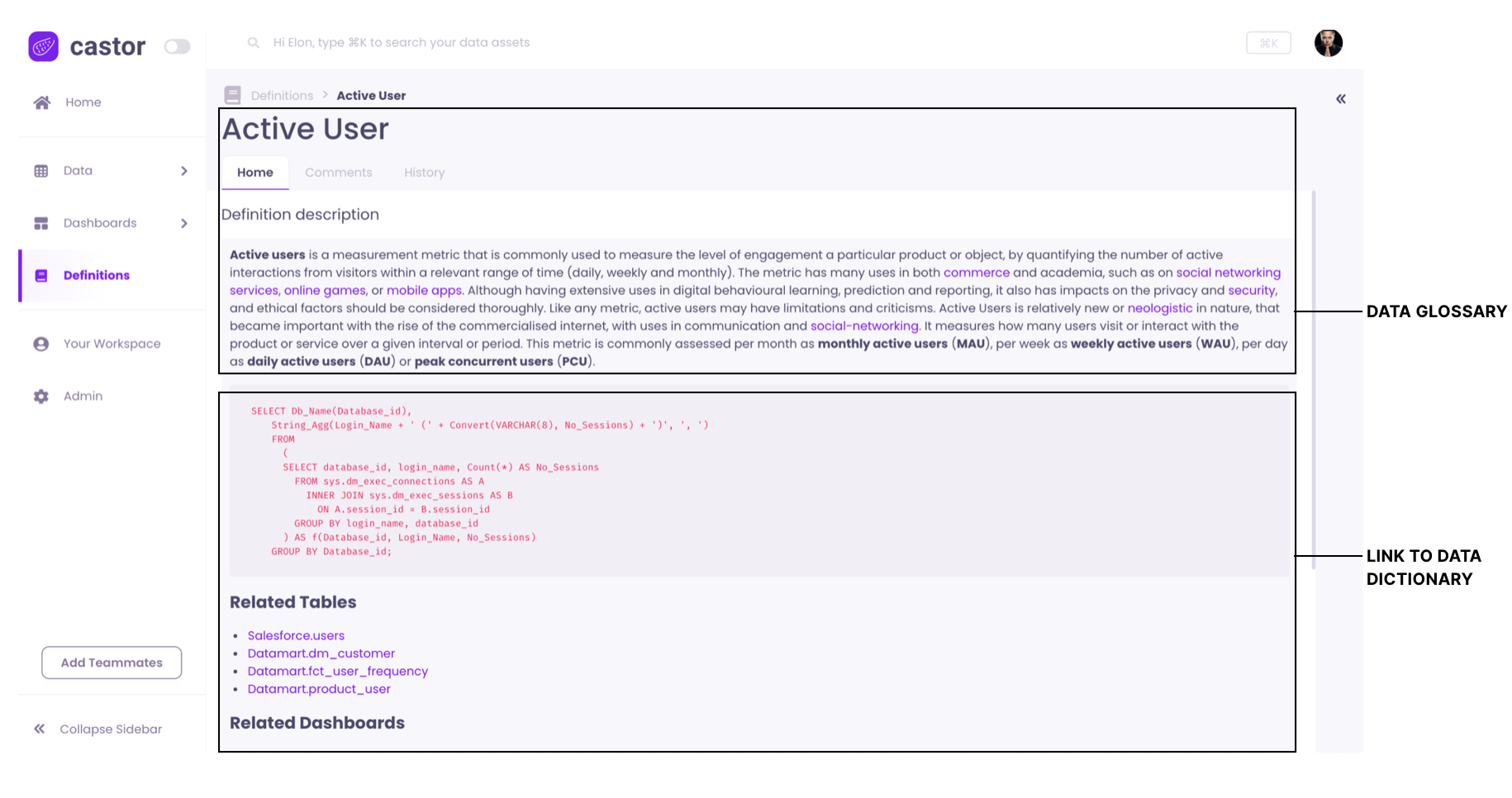
Combining your data glossary and data dictionary in one place benefits two main areas:
1. Enhanced data analysis
Linking your data dictionary and data glossary ensures that data analytics and business strategies align in your organization. Data catalog software connects business terms to specific tables and columns in a data dictionary, providing more context for each database. This connection is vital because clear business definitions have limited value if they don't relate to the underlying data. When data dictionaries and business glossaries are connected, users can quickly find data associated with a business term without needing help from the BI team. Also, if the technical documentation from the data dictionary isn't linked to the data glossary containing business definitions, the data team won't understand the context in which data sources are used.
2. Self-service analytics
Data manipulation was once exclusive to data scientists and data analysts, but now sales, marketing, and other operational departments use data daily. Self-service analytics allows non-technical users to access data for self-guided discovery, analysis, visualization, and hypothesis testing. When data assets are closely tied to business concepts through data catalog tools, it's easier for marketers and others to find the data they need.
Data catalogs provide open access to data for all users, regardless of their technical skills. This not only saves time for data users but also bridges the gap between a database and its related business context.
The key functionalities of a data catalog
An enterprise data catalog is a metadata management software, used for data governance and data discovery purposes. Looking at each use case:
- Data governance: As we have seen above, an enterprise data catalog links each data asset to its business context, making it easier for data users to understand the requirements around an asset. For example, for a given data asset, you understand both what the database contains and requirements around it: whether it contains PII information, how much time should pass before it is deleted, whether access to it should be open to the whole company, or restricted to a few roles only, etc
- Data discovery: With a data catalog, each data asset is enriched with context about what it contains, who imported it into the company, which dashboard and KPI it is related to, and any other information that can help data scientists locate it. In short, a data catalog makes your data discoverable by finally bringing clarity to your systems**.**
Modern data catalogs are completely automated tools, that use machine learning to enrich the documentation of your data warehouse. This way, they offer great support to data stewards, who don't have to document datasets manually thanks to these tools.
If you're looking for an enterprise data catalog, we've listed all the tools available on the market here (SaaS, open-source, etc..).
Subscribe to the Castor Blog
About us
We write about all the processes involved when leveraging data assets: from the modern data stack to data teams composition, to data governance. Our blog covers the technical and the less technical aspects of creating tangible value from data.
At Castor, we are building a data documentation tool for the Notion, Figma, Slack generation. We designed our catalog software to be easy to use, delightful and friendly.
Want to check it out? Learn more about CastorDoc with a free demo.
You might also like
Contactez-nous pour en savoir plus
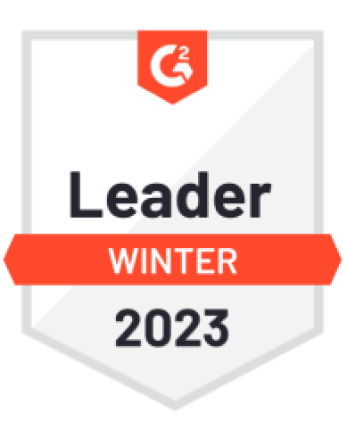
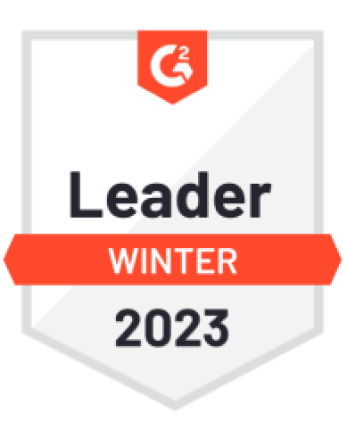

« J'aime l'interface facile à utiliser et la rapidité avec laquelle vous trouvez les actifs pertinents que vous recherchez dans votre base de données. J'apprécie également beaucoup le score attribué à chaque tableau, qui vous permet de hiérarchiser les résultats de vos requêtes en fonction de la fréquence d'utilisation de certaines données. » - Michal P., Head of Data.