What Is Master Data Governance?
Understand what MDM, Informatica/SAP/Oracle, Data Mesh means

Master Data Governance (MDG) is a set of processes, policies, standards, and tools that ensure the quality, consistency, and proper management of master data within an organization. "Master data" refers to the core data that is essential for business operations, like customer, product, or supplier information.
It plays a pivotal role in deriving insightful analytics, steering successful digital transformations, and in the broader spectrum, fostering cost control and agility.
In this article, we'll understand what master data governance is, in detail.
What Is Master Data?
Master data refers to the consistent and uniform set of identifiers and extended attributes that describes the core entities of an enterprise including customers, prospects, citizens, suppliers, sites, hierarchies, and chart of accounts. It provides a common point of reference across the organization, enabling a clear and connected view of business information.
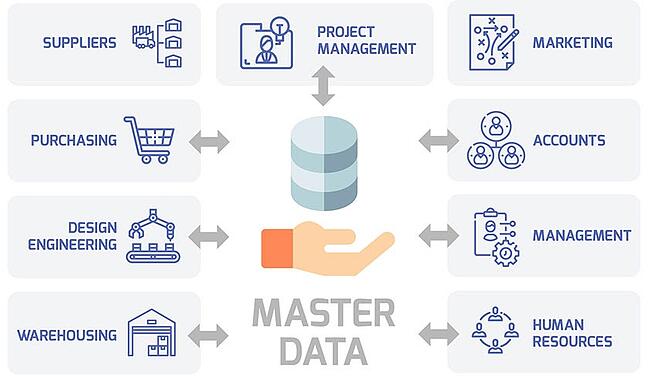
History of MDM
The history of Master Data (and by extension, Master Data Management and Governance) is intertwined with the evolution of information technology and business needs. Here's a brief timeline of its evolution:
1970s and 1980s: Emergence of Database Management Systems
- Early Database Systems: The emergence of database management systems (DBMS) like IBM's IMS and later relational databases like Oracle, allowed businesses to store structured data in a centralized manner.
- Data Redundancy Issues: As businesses adopted multiple systems (like financial, HR, and inventory systems), there was often redundant and inconsistent data across these systems.
Late 1980s and 1990s: Emergence of ERP Systems
- Enterprise Resource Planning (ERP): Systems like SAP and Oracle emerged, aiming to integrate multiple business functions into a single system. This reduced data redundancy but required significant efforts to standardize data.
- Data Warehousing: To solve the problem of fragmented data, businesses started building data warehouses to consolidate data from different sources, leading to the need for consistent master data.
2000s: Rise of Master Data Management (MDM)
- Recognition of Master Data: Organizations began to recognize that inconsistencies in master data (like customer or product data) across systems led to operational inefficiencies and poor decision-making.
- MDM Solutions: Vendors like Informatica, IBM, SAP, and others began offering MDM solutions to centralize, cleanse, and synchronize master data across systems.
- Regulations and Compliance: Events like the Sarbanes-Oxley Act (following corporate scandals) highlighted the need for data accuracy and accountability, further propelling the importance of MDM.
2010s: Expansion of Master Data Use Cases
- Digital Transformation: As businesses underwent digital transformations, the need for consistent data became paramount in areas like e-commerce, customer experience, and supply chain management.
- Big Data & Analytics: The rise of big data technologies and advanced analytics underscored the importance of clean, standardized master data to derive meaningful insights.
- Data Governance: With data becoming a strategic asset, organizations started focusing on data governance to ensure data quality, privacy, and compliance.
Master Data is crucial as it is foundational in ensuring consistency and control in the handling of data across the organization. Effective management of Master Data supports accurate analytics, reporting, and decision-making, fostering enhanced operational processes and ensuring regulatory compliance. It acts as the single source of basic business data used across all systems and processes within an organization, thereby eliminating data silos and redundancy.
What is Master Data Governance?
Master Data Governance refers to the practices, processes, and frameworks used to manage an organization’s critical data. It helps in ensuring accuracy, consistency, and accountability in the handling, integration, and usage of master data across the organization. It primarily focuses on the quality and lifecycle management of master data to facilitate improved decision-making and operational processes.
MDG explained to a 5-year old
Master Data Governance (MDG) is like having rules and referees for the important information a company uses. Imagine a game where everyone needs to agree on the rules to play it correctly. In a company, there's a lot of information (like details about customers, products, suppliers, etc.) that everyone needs to use. MDG makes sure this information is correct, up-to-date, and used the right way by everyone.
MDG explained in more details
Master Data Governance (MDG) is a set of processes, policies, standards, and tools that ensure the quality, consistency, and proper management of master data within an organization. "Master data" refers to the core data that is essential for business operations, like customer, product, or supplier information. With MDG:
- There's a consistent way to define, collect, and use this data across the organization.
- Errors and inconsistencies in data are minimized.
- People have clear roles and responsibilities for managing this data.
- There are checks and controls to make sure data is used correctly and safely.
MDG for Data Governance Experts
Master Data Governance (MDG) is a strategic discipline focused on the management of an organization's critical data assets to improve data quality, enhance operational efficiency, and ensure regulatory compliance. It encompasses:
- Processes and Workflows: Structured methods to create, validate, approve, and maintain master data records.
- Policies and Standards: Define how master data is classified, used, and archived.
- Data Stewardship: Assigning roles and responsibilities to individuals or teams to oversee and ensure the quality and proper use of master data.
- Data Quality Management: Implementing controls, metrics, and tools to continuously measure and improve the accuracy, consistency, completeness, and reliability of master data.
- Integration with IT Systems: Ensuring master data is consistently and effectively integrated across ERP, CRM, and other enterprise systems.
- Auditing and Compliance: Regular checks to ensure adherence to internal and external data standards and regulations.
The objective of MDG is to provide a single version of the truth for master data, reduce operational inefficiencies, mitigate risks, and enable better decision-making across the enterprise.
The Objective of Master Data Governance
The primary goal of the Master Data Governance strategy is to make sure everyone in an organization is on the same page when it comes to data. Imagine having a shared dictionary that everyone refers to, ensuring they're all using the same definitions and understanding.
By setting straightforward rules for data from the moment it's created until it's no longer needed, Master Data Governance makes sure our data is both trustworthy and consistent. This not only helps companies make smarter decisions but also streamlines daily operations, ticks off compliance boxes, and drives them toward their bigger-picture goals.
Core Components of Master Data Governance
The Master Data Governance program is constructed on several pillars. Data Stewardship is about appointing stewards to maintain and oversee data. Data Quality is essential to ensure the information is accurate and reliable. It also involves establishing Data Policies and Standards, which serve as the rules and guidelines for managing data.
To make all this work seamlessly, the right Data Processes and Workflow, coupled with suitable Data Architecture and Technology Solutions, are implemented.
A Step-by-Step Approach to Master Data Governance
Here's a comprehensive step-by-step approach to, how to implement master data governance initiatives systematically:
Step 1: Define the Master Data
Start by taking a close look at the data your organization deals with daily. It's crucial to pinpoint exactly what falls under the umbrella of 'master data' for your business. This usually means zeroing in on key sources like your customer records, the products you offer, your suppliers, and more.
So, let's say we're talking about your customers. We're not just talking names here, think contact info, their shopping habits, likes, and even dislikes.
Step 2: Establish Governance Roles and Responsibilities
Once the master data is defined, the next step is to establish the roles and responsibilities. This might involve creating a data governance council or committee, assigning data stewards, and explaining their individual duties.
Step 3: Develop Governance Policies and Procedures
The main aspects of master data governance lie in its policies and procedures. This is where you establish the guidelines for how master data is to be collected, stored, accessed, updated, and deleted within the organization. It should also cover aspects like data security, privacy, and quality control for effective master data governance.
Step 4: Implement Data Quality Measures
Ensuring the quality of the master data is a critical aspect of data governance. This involves setting up data quality measures, which might include data validation rules, data cleaning processes, and data quality metrics. These measures help to identify and correct errors, ensuring improved data quality so that the master remains accurate and consistent.
Step 5: Establish a Data Audit Process
A data audit process is essential for ensuring ongoing compliance with the governance policies and procedures. Regular audits can help identify any gaps or weaknesses in the data governance framework, allowing for timely corrective actions.
Step 6: Choose the Right Tools
Select appropriate technologies and tools that support your governance framework. These tools will help in implementing the defined policies and processes effectively and ensure seamless management of data.
Step 7: Regularly Review and Update the Governance Framework
Master data governance is not a one-time task but an ongoing process. As your business evolves, your data governance framework should also evolve. Regular reviews and updates ensure that the framework remains relevant and effective.
Challenges in Master Data Governance
Here are a few challenges associated with master data governance -
- Data Silos: Different departments often store their data separately, making it difficult to have a unified view. These isolated data pockets can hinder the seamless flow and integration of information.
- Quality Issues: Inaccuracies, duplications, or outdated information can creep into databases, affecting decision-making and operational efficiency.
- Organizational Change Management: Shifting to a new data governance model means changing existing processes. Resistance from employees or lack of clarity in the transition can lead to hiccups.
- Unclear Ownership: Without clear roles and responsibilities, it’s hard to pinpoint who is accountable for data quality and maintenance. This ambiguity can lead to neglect.
- Existing Technology Constraints: Outdated systems or those not equipped to handle advanced data governance might slow down or complicate the process.
Benefits of Effective Master Data Governance:
Here are a few benefits of data governance for master data -
- Enriched Business Intelligence: With reliable data, analytics become sharper, leading to more informed business decisions.
- Improved Customer Satisfaction: Consistent and accurate data can result in better customer insights and interactions, enhancing their experience.
- New Revenue Avenues: Clean, integrated data can spotlight previously unseen opportunities, paving the way for business growth.
- Reduced Operational Costs: Efficient data management minimizes errors and redundancies, leading to cost savings.
- Streamlined Compliance: Effective governance ensures that data adheres to both internal standards and external regulations, simplifying compliance efforts.
You might also like
Contactez-nous pour en savoir plus
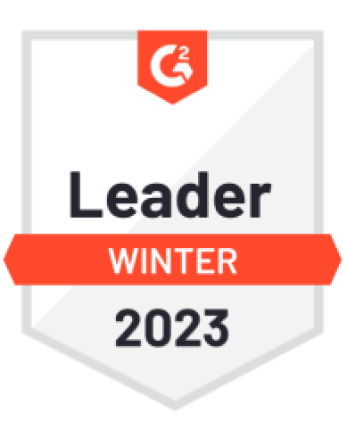
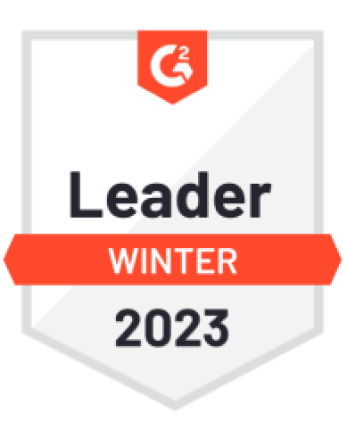

« J'aime l'interface facile à utiliser et la rapidité avec laquelle vous trouvez les actifs pertinents que vous recherchez dans votre base de données. J'apprécie également beaucoup le score attribué à chaque tableau, qui vous permet de hiérarchiser les résultats de vos requêtes en fonction de la fréquence d'utilisation de certaines données. » - Michal P., Head of Data.