Your Guide to Building an Effective Data Governance Framework
Lessons from an Expert on Building Data Governance that Works
%202.png)
Introduction
There are plenty of data governance frameworks out there, from McKinsey to DAMA DMBOK. While these frameworks are great at laying a theoretical foundation, they often leave practitioners wondering how to adapt them to their specific context and challenges.
This is where learning from experience becomes interesting. I recently interviewed Charlotte Ledoux, who coordinates the data governance program at Pernod Ricard, to understand how frameworks translate into real-world implementation. Charlotte has faced and solved concrete challenges that many organizations experience, from adapting frameworks for specific industry needs to measuring the real impact of governance initiatives.
Her insights offer practical guidance whether you're in consumer goods dealing with retail data challenges, in healthcare managing patient data privacy, or in any industry trying to improve data quality and usage.
But first, let's get clear on what a data governance framework is.
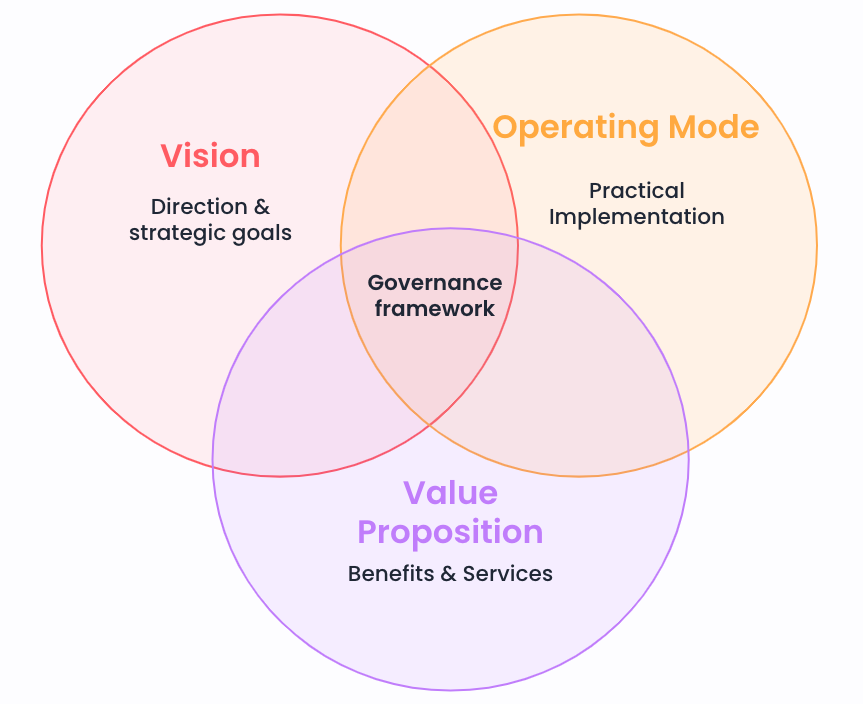
A data governance framework is a comprehensive methodological structure that serves multiple key purposes in an organization's data management approach. At its core, a data governance framework helps build and define three aspects:
First, it establishes the vision of the Data Governance team - what they aim to achieve and how they fit into the broader organization. This vision component is crucial as it sets the direction for all governance activities.
Second, it defines the operating mode - the practical aspects of how the Data Governance team will function. This includes determining how the team should interact with other departments, what resources they'll need, and how they'll carry out their responsibilities.
Third, it outlines the team's value proposition - what specific benefits and services the Data Governance team will provide to the organization.
In this article, we'll unpack what it takes to implement a successful data governance framework, based on Charlotte's hands-on experience.
We'll begin by examining the pillars of any robust framework - elements like data quality, accessibility, compliance, and security that shape how your governance team operates.
From there, we'll look at the practical steps of starting your governance initiative, especially relating to aligning your framework with business needs and building a team with the right mix of skills.
You'll learn how to measure your governance initiatives' impact, moving beyond basic metrics to understand real business value.
Finally, we’ll look at where AI fits in your data governance initiative - and how you can leverage it to draft your framework and lighten the weight of documentation, amongst other things.
I hope you’re ready - let’s dive in.
I - Key pillars of a data governance framework
You can think of a data governance framework as the nervous system of your data organization - it needs to sense, respond, and coordinate across multiple dimensions simultaneously. These dimensions are the following:

Data Quality
Your data must be reliable. This means keeping data accurate and consistent across all your tools and users. Without quality data, other initiatives will fail.
Data Accessibility
Here's a paradox every data team faces: how do you make data both accessible and secure? This requires clear rules about who can access what data and when.
Compliance
Compliance has become a critical pillar of data governance with the increasing complexity of data privacy regulations such as GDPR, HIPAA, and CCPA. Establishing clear policies and procedures for data collection, storage, and usage is an essential part of any governance framework.
Data Security
Remember the last major data breach that made headlines? That's exactly what data security prevents. Protecting sensitive data from unauthorized access, breaches, and misuse is a top priority for any data governance framework.
Documentation
Good documentation explains what data exists and how to use it. This includes recording metadata, tracking where data comes from, and defining key terms. Documentation helps everyone understand and use data correctly.
Data Structure and Taxonomy
How do you make sense of a data landscape that's growing exponentially? Organizations need a clear system for organizing their growing data. A good structure makes it easier to find, understand, and use data for decision-making.
Organizations need a clear system for organizing their growing data. A good structure makes it easier to find, understand, and use data for decision-making.
These six components must work together. Your framework will succeed when you can implement all of them in a coordinated way.
Let's look at how to put these components into practice...
II - Starting your Data Governance Journey
A data governance framework needs two essential elements before you begin implementing it. First, make sure your framework aligns with how your business actually operates. Second, assemble a team that matches your specific requirements. While it's tempting to immediately start using frameworks and tools, these two foundational steps must come first.
Start with the Business Reality
A data governance framework must solve actual business problems to be useful. At Pernod Ricard, Charlotte started by talking to the people who use data every day.
Through business team interviews, patterns of data challenges typically emerge:
- Teams struggling to access basic operational data
- Dashboards showing conflicting numbers
- Data jumping between systems and losing consistency
- Hours lost to manual Excel reconciliations
These problems point to specific governance needs in the organization. By focusing on these real issues first, you can create a framework that addresses actual problems instead of theoretical ones.
Build the Right Team From the Start
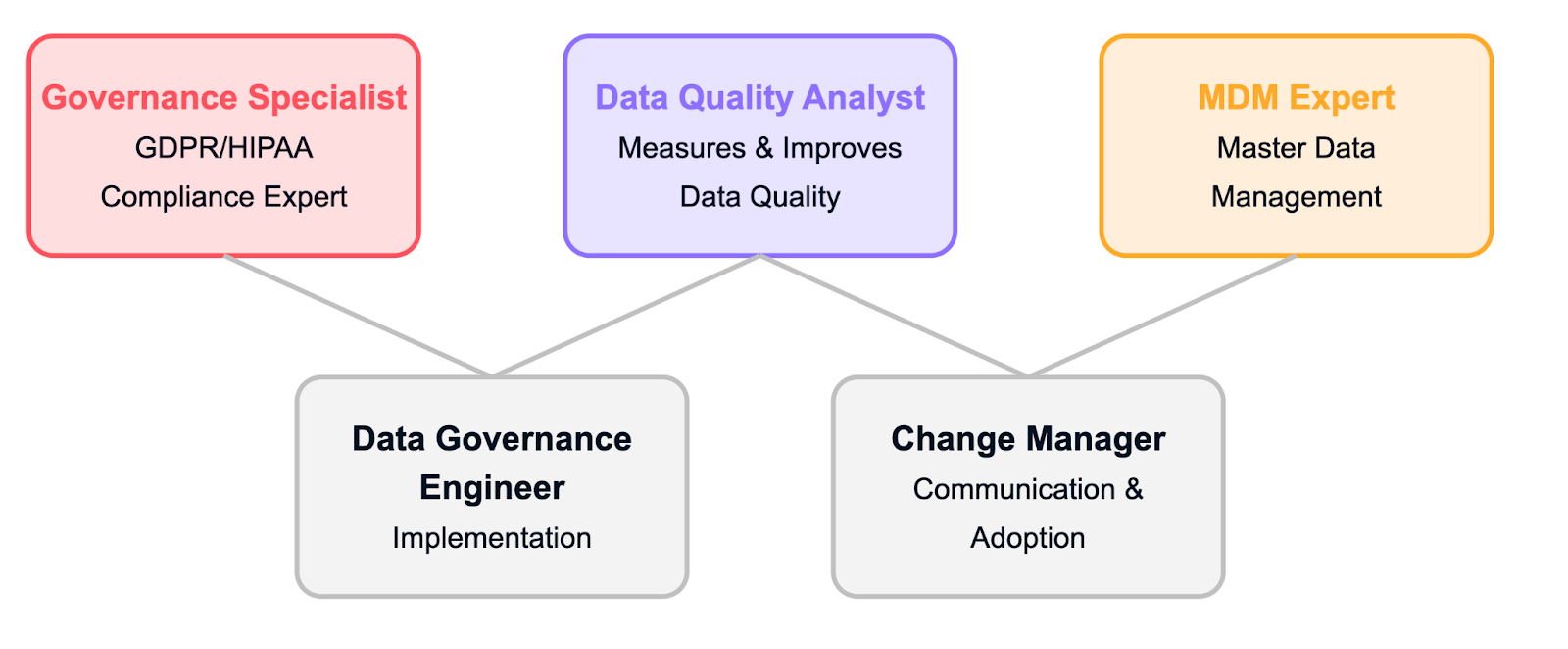
Different data problems need different types of experts. Here's what to consider when building your team:
In healthcare or banking, you need governance specialists who understand GDPR or HIPAA regulations. These experts design systems that keep data flows compliant with laws.
When data quality is the main problem, Data Quality Analysts are essential. They measure exactly where and how data problems occur, replacing vague complaints with clear evidence.
Some organizations need Master Data Management (MDM) experts. As Charlotte notes, MDM works well with governance, especially for managing complex data hierarchies.
A newer role is the Data Governance Engineer. This person connects governance theory to practical implementation, making sure frameworks actually work in real systems.
One crucial skill is change management and communication. Your team needs people who can explain governance clearly to different groups. Without this, even the best framework will fail because people won't use it properly.
This approach works because it starts with real problems. When you build a team that matches your actual needs, your governance framework begins delivering value immediately. The goal isn't governance for its own sake - it's solving business problems through better data management.
III - Measuring the Success of Your Data Governance Initiative
Measuring the success of data governance is crucial but often challenging. Charlotte shared a practical approach that moves beyond typical implementation metrics to track real business impact.
The Two-Tier Measurement Approach
Basic tracking metrics are important but not enough. While you should count things like how many areas are governed and who has what roles, these numbers don't show the real business impact.
Charlotte shared how Pernod Ricard measures success effectively. They track how much time teams spend on data tasks each day, week, and month. This shows them if their data operations are becoming more efficient.
Industry-Specific Governance Goals
Each industry has its own reasons for implementing data governance. Make sure you measure success based on what matters to your industry:
Consumer goods companies like Pernod Ricard focus on better forecasting. For example, they struggle to predict wine and spirit sales in retail stores when data is inconsistent. The governance initiative helps them create clear rules for collecting store data and setting quality standards in their provider contracts. They measure the success of the governance initiative by how accurately they can predict sales and plan deliveries. A 10% improvement in forecast accuracy means millions in saved logistics costs.
Software companies want to make better operational decisions. Their success metrics focus on operational efficiency and customer insights. They track how quickly teams can access reliable data for decisions, measuring reduced time spent on data preparation and fewer conflicts between different data sources.
Healthcare organizations like Doctolib must protect patient data and follow regulations. They measure success first through compliance metrics: number of data breaches prevented, percentage of data access properly tracked, and time to respond to patient data requests. While they start with these requirements, they discover additional metrics matter too: fewer data errors in patient records, faster access to correct information by authorized staff, and increased user confidence in handling sensitive data.
Choose your success metrics based on what your industry needs most from data governance.
The Defensive-Offensive Framework
This diversity in impact measurement aligns with what Charlotte describes as "defensive" versus "offensive" governance approaches. Each approach needs different success metrics:
The defensive approach focuses on protection. Companies measure success through risk indicators: number of data incidents prevented, compliance requirements met, and security breaches avoided. Banks and healthcare companies often start here.
The offensive approach aims to create business value. Success metrics include improved sales forecasts, faster product launches, or better customer insights. Consumer goods and technology companies typically take this route.
Most organizations use both approaches. A retail company might track both their compliance with privacy laws and how their better data helps increase sales. A healthcare company might measure both patient data protection and how cleaner data speeds up treatment decisions.
Choose metrics that match your approach. If you focus on defense, track risk and compliance. If you focus on offense, measure business improvements. If you use both, balance your metrics accordingly.
IV - Where does AI fit in my Data Governance Framework?
AI is becoming a useful tool to help with data governance. Here's what I learned from Charlotte about how organizations can leverage AI as part of their governance initiative.
Accelerating Framework Development
AI can help your organization set up its governance framework faster. It can quickly read and summarize documentation, find common themes in team interviews, and suggest where improvements are needed most. This is especially helpful when dealing with large amounts of information from different teams.
Finding Patterns and Reducing Bias
AI analyzes data governance objectively by tracking how often problems occur, which departments face which challenges, what needs fixing first, and how different teams' needs connect. This helps ensure all departments get equal attention, even when governance teams work more closely with some groups than others.
Improving Documentation
AI makes documentation work easier by analyzing existing documents, finding missing pieces in frameworks, suggesting improvements, and helping maintain consistent documentation. However, Charlotte emphasizes an important point: AI should help humans make better decisions, not replace their judgment. The best results come from combining AI's efficiency with human expertise.
Understanding How People Use Data
When people interact with AI-powered data tools, we learn valuable information. Charlotte explains that AI shows us which data people use most often, what questions they commonly ask AI assistants, and where they have trouble finding or using data. This helps create intent-based governance frameworks that better match what people actually need.
Conclusion
Implementing data governance isn't just about following a framework - it's about solving real business problems. Through Charlotte's experience, we've learned several key lessons:
Start with your business reality. Talk to your teams, understand their data challenges, and build your framework around their actual needs. A framework that doesn't solve real problems won't succeed.
Build the right team for your specific challenges. Whether you need compliance experts, data quality analysts, or change management specialists, match your team's skills to your organization's needs.
Measure what matters to your industry. Consumer goods companies might track forecast accuracy, while healthcare organizations focus on patient data protection. Choose metrics that reflect your specific goals.
You might also like
Contactez-nous pour en savoir plus
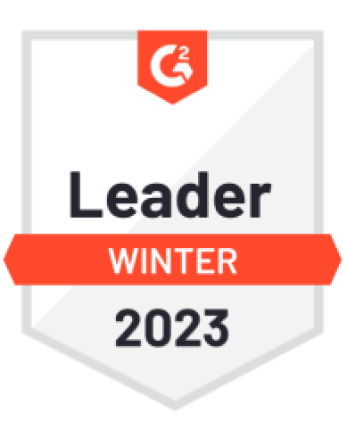
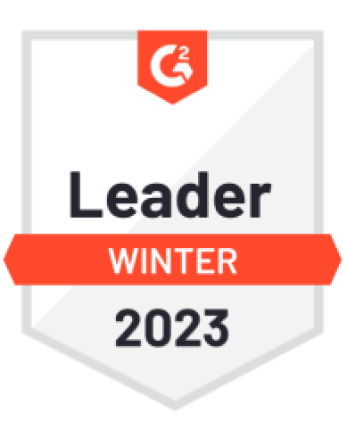

« J'aime l'interface facile à utiliser et la rapidité avec laquelle vous trouvez les actifs pertinents que vous recherchez dans votre base de données. J'apprécie également beaucoup le score attribué à chaque tableau, qui vous permet de hiérarchiser les résultats de vos requêtes en fonction de la fréquence d'utilisation de certaines données. » - Michal P., Head of Data.