How AI is turning every employee into a Data Analyst
While Preserving Expert Roles

Introduction
AI is transforming the workplace, empowering employees across every department to become data-driven decision-makers. No longer confined to data specialists, teams in marketing, finance, HR, and beyond are now actively engaging with data, driving smarter business choices. This transformation marks an exciting leap forward, making data accessible to everyone.
Consider Panasonic North America: their HR team leverages AI to analyze employee data and predict hiring needs, allowing them to make informed decisions without needing to be data experts. This kind of AI-driven insight is reshaping how companies operate, allowing non-technical staff to take on tasks previously reserved for data scientists.
By automating routine analysis, AI is democratizing data, fostering a data-driven culture across organizations. Yet, while AI enables employees to perform entry-level data tasks, the expertise of data professionals remains indispensable for tackling complex analysis and guiding long-term strategy.
In this article, we’ll explore how AI is transforming employees into data analysts and why skilled professionals are still key for advanced analytics and strategic insights.
The Key Data Obstacles Businesses face
Businesses face several key challenges when it comes to leveraging their data:
- Data complexity: Data is often filled with technical jargon, making it hard for non-technical users to engage with it.
- Data overload: Daily data overload, known as "digital debt," burdens teams with time-consuming documentation tasks. The volume of data can be overwhelming, leading to decision paralysis.
- Lack of technical expertise: Data analysis can require coding and statistical skills, which many decision-makers do not possess.
- Time constraints: Business professionals typically don’t have the time to sift through data to extract insights.
The Role and Core Functions of a Data Analyst
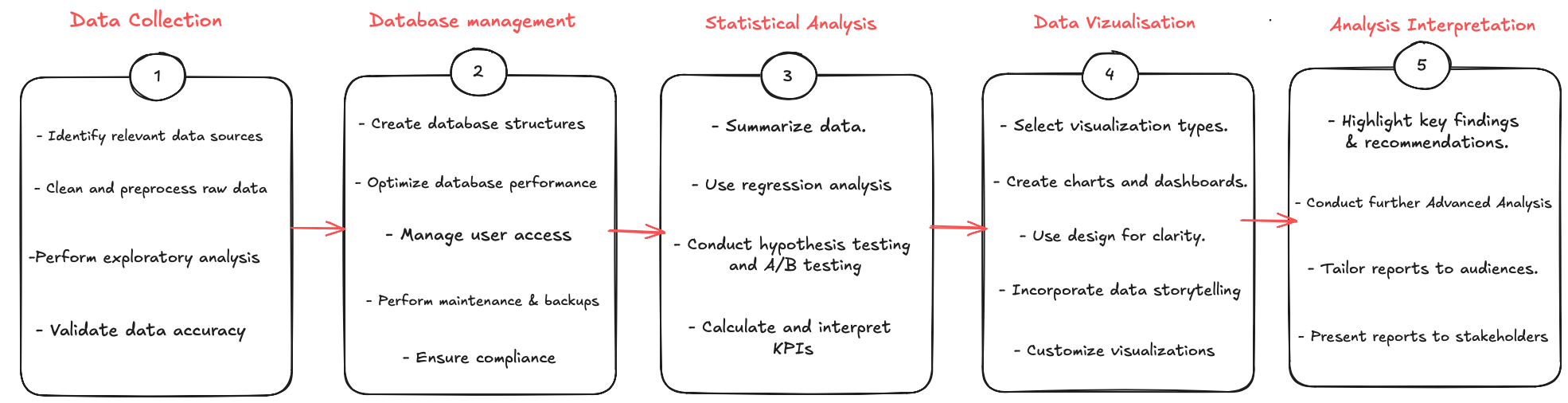
This is where Data Analysts come into play. Their job? To uncover valuable insights hidden within mountains of information:
“The role of data and analytics is to equip businesses, their employees and leaders to make better decisions and improve decision outcomes” Gartner
Let's break down what they do:
Data Analysts are both data collectors and number crunchers, gathering information from sources such as company databases, social media APIs, and web scraping (data collection).
Once they have the data, they ensure it’s neatly organized and accessible through database management.
After organizing the data, they apply statistical techniques to make sense of it (statistical analysis). Then, the magic happens: Data Analysts turn numbers into visuals (data visualization), creating charts and dashboards that make complex information easy to digest.
But it's more than just visuals. They compile reports that highlight key findings and actionable insights, seeking trends and patterns (analysis interpretation). Data Analysts work closely with companies’ domain teams to understand needs, translating business questions into data queries and results into strategy.
Using tools like Python, R, Tableau, Power BI, and SQL, they collaborate with data scientists to extract the full value from data. Ultimately, Data Analysts bridge the gap between raw data and informed decision-making, turning numbers into knowledge that drives business forward.
AI Making Data Analysis Accessible for All Employees
AI is transforming data analysis by enabling non-technical employees to handle many of the responsibilities traditionally managed by data analysts. The core functions we outlined—data collection, statistical analysis, visualization, and interpretation—can now be performed by non-technical teams, thanks to AI-driven tools.
For instance, marketing teams can use AI to predict campaign performance, HR can assess employee sentiment with sentiment analysis, and finance teams can forecast revenue trends, all without needing specialized technical skills. AI-powered platforms simplify tasks such as Natural Language Processing (NLP), allowing employees to ask questions like “What were our sales last quarter?” in plain language, eliminating the need for coding.
With tools like ChatGPT, non-technical users can generate queries, interpret results, and create reports effortlessly, bridging the gap between technical expertise and data-driven decision-making. As businesses increasingly rely on scalable, cloud-based AI tools, data analysis is becoming more accessible across the organization. By 2025, 95% of digital workloads will be on cloud-native platforms, empowering employees at all levels to engage with data insights and drive business outcomes.
Empowering Employees through Self-Service Analytics

AI is transforming how employees use data at work. Think of it like this: data analysis used to be like a meal kit - employees had to build everything from scratch. Now, it's more like a buffet. The dishes are already prepared, and staff just need to pick what they want and maybe add a little customization. This shift, known as self-service analytics, allows team members to generate reports and get answers without relying on data experts.
This transformation means that departments can work independently with data, reducing bottlenecks and speeding up decision-making processes.
Ultimately, AI serves as a translator, converting business needs into data queries, building trust in the results, and encouraging independent data exploration. Marketing, sales, and HR teams can now make quick, data-driven decisions, creating a more empowered workforce and boosting overall company performance and competitiveness.
AI’s Role in Automating Data Tasks
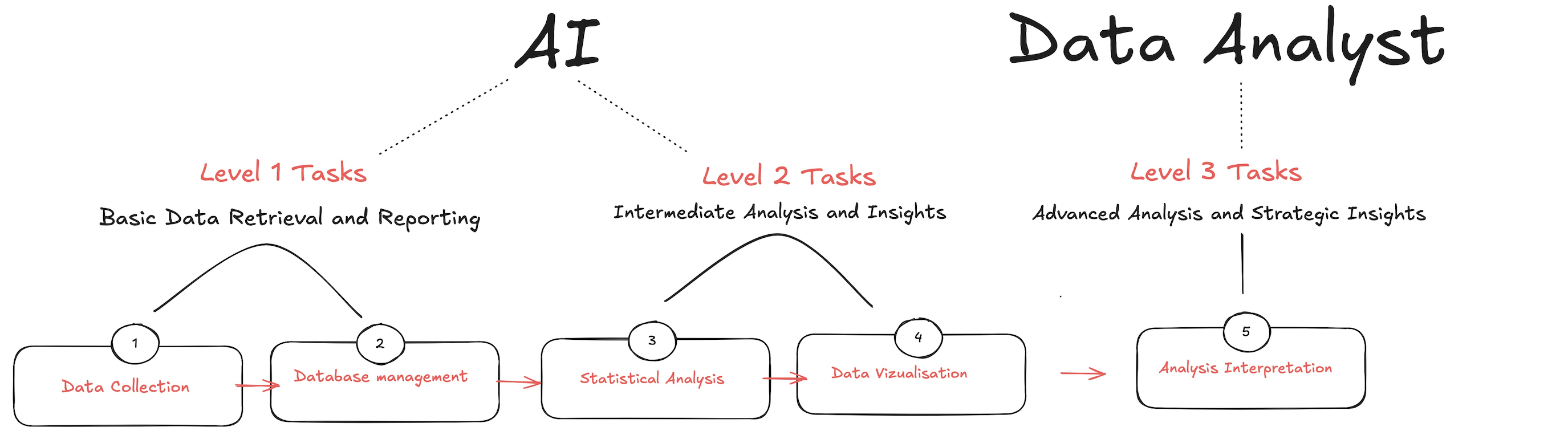
AI is transforming data teams by automating routine tasks, allowing employees to focus on more strategic work. As Tristan Mayer, our CEO, outlined in his recent post, these tasks fall into three levels:
Level 1 Tasks: Basic Data Retrieval and Reporting
AI-powered assistants like chatbots can handle routine queries such as "Where's the MRR dashboard?" or "What's the churn rate definition?" These tasks, typically handled by junior analysts, involve straightforward data extraction.
‍Level 2: Intermediate Analysis and Insights
AI is advancing in handling Level 2 support tasks, which typically require junior analyst skills like interpreting data, comparing metrics, and identifying performance drivers. For instance, tasks like “How does customer retention differ between regions?” or “What’s the impact of pricing changes on sales?” need more than just data extraction—they require analysis. While not fully autonomous at this level, AI copilots assist analysts by interpreting and visualizing data, though human judgment is still required.
Level 3: Advanced Analysis and Strategic Insights
This is where senior analysts operate, focusing on complex business challenges like identifying strategic initiatives or evaluating customer lifetime value. These tasks involve deep analysis, context interpretation, and decision-making, requiring a combination of technical expertise and business acumen.
AI is already automating Level 1 tasks and some Level 2 tasks. As AI technology continues to improve, non-technical users will gain even more capabilities for data analysis. However, human expertise will remain essential for interpreting nuanced business contexts and making strategic decisions.
Limitations to think about

Sure, AI is making data analysis easier for non-tech experts, but it still faces several limitations. While it's great for the basics, like pulling up reports or making chart, more advanced, strategic analysis (level 3 tasks) continues to require human expertise.
There are two key challenges: the human skills gap and AI's technical limitations. On one hand, many employees lack the advanced data analysis skills that AI can't yet replicate. On the other hand, AI has its own technical constraints that need human oversight to overcome.
The Human Skills Gap
Technical Proficiency and Critical Thinking
While AI can process large amounts of data, the quality of insights depends on the ability to ask the right questions and interpret the results. Organizations need people who can interpret and validate AI-generated results. It's about enhancing team capabilities, not replacing them.
Consider Spotify: AI creates personalized playlists, but human experts still curate selections to catch what AI might miss. This human touch ensures a well-rounded user experience.
Handling Nuance, Ambiguity, and Contradictory Information
When it comes to complex, ambiguous data - which is often the case in business - AI also has limitations. AI is great at handling organized data and spotting patterns, but it has trouble with unclear or messy information. Complex data with gaps or contradictions often needs human analysts to find hidden insights.
Netflix uses AI to recommend shows, but human analysts are needed when the data is mixed. AI might suggest promoting a show with high early views, but human analysts might see that many people don’t finish it, leading to more nuanced promotion strategies.
The Need for Business Domain Expertise
AI lacks the industry-specific knowledge that experienced professionals bring to the table. It can identify trends, but it doesn't understand a company's unique goals, market conditions, or culture. This context is crucial for translating data into effective business strategies.
For example, while AI at Netflix can predict what people will watch, human experts are still needed to decide which shows to create. Netflix’s Co-CEO, Ted Sarandos, highlights that human judgment is important for choosing shows and understanding cultural details that AI might overlook.
In essence, AI is most effective when combined with human expertise. The focus should be on leveraging AI to augment team skills, not replace them.
Technical Challenges in AI’s Adoption
Data Handling
Large Language Models (LLMs) aren’t designed to handle numerical data or complex datasets on their own. They can’t fully interpret graphs, tables, or perform detailed statistical analysis without human help. While AI can identify trends, humans are still needed to guide the analysis, ensure accuracy, and provide context to the results.
Algorithm Expertise
Despite AI's capabilities, it doesn't build itself. Organizations need data experts to create and fine-tune AI models for specific business needs. Without this expertise, companies might struggle to:
- Develop AI solutions tailored to their unique challenges
- Optimize AI performance for accuracy and efficiency
- Resolve issues when AI insights don't align with business realities
Evolving Technology
AI tools are changing rapidly, which brings its own set of challenges:
- AI tools aren't always consistent or reliable yet
- Many AI applications are still in early stages
- Integrating AI with existing business systems can be tricky
In essence, AI is not a plug-and-play solution. Organizations need to blend AI capabilities with human expertise to navigate these technical hurdles effectively. The key is to approach AI adoption strategically, keeping in mind both its potential and its current limitations.
Conclusion
AI is transforming how businesses approach data analysis, making it more accessible to non-technical employees by automating routine tasks like data retrieval and reporting. By empowering non-technical teams to work with data, AI boosts productivity and helps create a more data-centric workplace.
However, AI alone can't handle complex analysis or strategic decision-making. Human expertise remains crucial for interpreting nuanced data, understanding business context, and applying critical thinking to solve complex problems.
Ultimately, AI enhances the capabilities of employees, but its full potential is realized when combined with the insights and expertise of skilled data professionals. Together, AI and human analysts form a partnership that drives more effective, data-driven decision-making across the organization.
You might also like
Get in Touch to Learn More
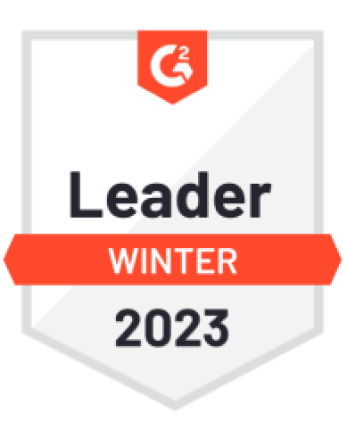
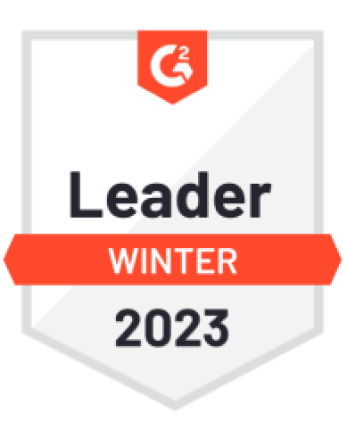

“[I like] The easy to use interface and the speed of finding the relevant assets that you're looking for in your database. I also really enjoy the score given to each table, [which] lets you prioritize the results of your queries by how often certain data is used.” - Michal P., Head of Data