Data Anomaly & Quality Monitoring: Importance, Impact & Roadmap
Learn about the critical role of data anomaly and quality monitoring in ensuring accurate and reliable insights.

Organizations rely heavily on the accuracy and reliability of their data. However, data anomalies and poor data quality can significantly impact the effectiveness of business operations. This article aims to shed light on the importance of data anomaly and quality monitoring, their impact on decision making, and strategies to create an effective roadmap for managing these issues.
Understanding Data Anomalies
Before diving into the world of data quality monitoring, it is important to understand what data anomalies are and their various types. A data anomaly refers to any deviation or irregularity in data patterns that cannot be explained by the standard process or expected behaviors. These anomalies can manifest in different forms, such as missing data, incorrect values, outliers, or inconsistent records.
Causes of data anomalies can vary, ranging from human error during data entry to technical glitches in data processing systems. Regardless of the cause, it is crucial to identify and address these anomalies promptly to ensure the integrity of data.
Data anomalies are not always negative; they can sometimes reveal valuable insights or trends that were previously unnoticed. For example, an outlier in sales data might indicate a sudden surge in demand for a particular product, prompting further investigation and potentially leading to strategic business decisions.
It is essential for organizations to establish robust data anomaly detection mechanisms to proactively monitor data quality and prevent potential issues before they escalate. This can involve setting up automated alerts, implementing data validation checks, and conducting regular data audits to maintain data accuracy and reliability.
The Significance of Data Quality Monitoring
Data quality monitoring plays a pivotal role in maintaining the accuracy, completeness, and consistency of data. By establishing an effective data quality monitoring system, organizations can ensure that their decisions are based on trustworthy and reliable information.
Implementing a comprehensive data quality monitoring strategy involves continuous evaluation of data sources, data processing methods, and data storage systems. This proactive approach allows organizations to detect and rectify data quality issues in real-time, minimizing the risk of making decisions based on flawed data.
Role of Data Quality in Business Decision Making
Businesses heavily rely on data-driven insights to make informed decisions. Data quality directly impacts the reliability and effectiveness of these insights. Poor data quality can lead to erroneous conclusions and flawed strategies, jeopardizing the success of the organization.
Moreover, in today's competitive business landscape, organizations that prioritize data quality gain a significant competitive advantage. High-quality data enables companies to respond swiftly to market changes, identify emerging trends, and capitalize on new opportunities, setting them apart from their competitors.
On the other hand, high-quality data enables organizations to gain deeper insights, identify patterns, and make reliable predictions. This reinforces the importance of investing in robust data quality monitoring practices.
Impact of Poor Data Quality
Poor data quality poses numerous risks and challenges for organizations. Inaccurate or incomplete data can lead to misguided decisions, negatively impacting operational efficiency, customer satisfaction, and financial performance.
Furthermore, the repercussions of poor data quality extend beyond internal operations. In the age of data privacy regulations and increasing consumer awareness, organizations that fail to maintain high data quality standards risk losing customer trust and loyalty. Data breaches, legal penalties, and reputational damage are all potential consequences of subpar data quality. Therefore, it is imperative to address data quality issues proactively.
The Interplay between Data Anomalies and Quality Monitoring
Data anomalies and quality monitoring share an intricate relationship. Anomalies can arise due to data quality issues, and effective monitoring can help detect and mitigate these anomalies.
Understanding the root causes of data anomalies is crucial in maintaining high data quality standards. Anomalies can stem from various sources such as human error during data entry, system glitches, or inconsistencies in data processing workflows. By conducting thorough root cause analysis, organizations can pinpoint the underlying issues contributing to anomalies and implement targeted solutions to prevent their recurrence.
How Anomalies Affect Data Quality
Data anomalies have a cascading effect on data quality. Anomalies can distort statistical analysis, hinder accurate reporting, and compromise data integrity. If left unchecked, anomalies can lead to a snowball effect, gradually eroding the trustworthiness of the entire dataset.
Moreover, data anomalies can have far-reaching implications beyond data quality issues. In sectors such as finance and healthcare, anomalies in critical datasets can result in erroneous decisions, financial losses, or even pose risks to public health and safety. Therefore, maintaining a vigilant stance towards anomaly detection and data quality monitoring is paramount in safeguarding the reliability and credibility of data-driven processes.
Using Quality Monitoring to Detect Anomalies
Data quality monitoring systems leverage various techniques and tools to detect anomalies effectively. Automated anomaly detection algorithms, statistical analysis, and machine learning models help identify patterns that deviate significantly from the norm.
Furthermore, proactive quality monitoring not only aids in anomaly detection but also facilitates continuous improvement initiatives. By analyzing historical data trends and patterns, organizations can proactively enhance data quality processes, optimize data collection methods, and strengthen data governance frameworks. This iterative approach to quality monitoring fosters a culture of data-driven decision-making and ensures that data anomalies are swiftly identified and addressed to maintain data integrity.
Roadmap to Effective Data Anomaly and Quality Monitoring
An effective roadmap is crucial for successfully implementing a data anomaly and quality monitoring system. It involves defining the right processes, utilizing the appropriate technology, and fostering a data-driven culture within the organization.
Building a solid foundation for data anomaly and quality monitoring begins with a comprehensive assessment of the organization's data ecosystem. This involves conducting data audits, understanding data flows, and mapping out data dependencies across systems and departments. By gaining a holistic view of the data landscape, organizations can identify potential vulnerabilities and areas for improvement.
Steps to Implementing a Data Quality Monitoring System
The first step towards effective data quality monitoring is understanding the organization's data landscape. This includes identifying key data sources, defining data quality metrics, and establishing data governance policies.
Once the groundwork is laid, organizations can invest in advanced data quality monitoring tools and build robust data pipelines. Continuous monitoring, regular data profiling, and periodic audits should be integrated into routine operations.
Enhancing data quality monitoring efforts involves leveraging machine learning algorithms and artificial intelligence to automate anomaly detection and data cleansing processes. By harnessing the power of predictive analytics, organizations can proactively identify and address data quality issues before they escalate.
Strategies for Effective Anomaly Detection and Management
Successful anomaly detection and management require a multi-faceted approach. Organizations should combine automated anomaly detection algorithms, statistical analysis, and manual validation processes to achieve optimal results.
Furthermore, organizations need to establish clear protocols for handling different types of anomalies. This includes defining escalation paths, assigning responsibility for resolution, and developing robust incident response plans.
Collaboration between data scientists, data engineers, and business stakeholders is essential for effective anomaly detection and management. By fostering cross-functional teamwork and knowledge sharing, organizations can enhance their ability to detect, investigate, and remediate anomalies in a timely manner.
Future Trends in Data Anomaly and Quality Monitoring
The field of data anomaly and quality monitoring is continuously evolving, driven by advancements in technology and the growing complexities of data ecosystems. Two significant trends that will shape the future of this field are the increased role of AI and machine learning and predictions for the future of data quality monitoring.
The Role of AI and Machine Learning
Artificial intelligence and machine learning have the potential to revolutionize data anomaly and quality monitoring. Advanced algorithms can detect anomalies in real-time and automatically adapt to changing data patterns. This minimizes human intervention and allows organizations to identify anomalies swiftly, ensuring data accuracy and reliability.
Furthermore, AI and machine learning can also be utilized to predict potential anomalies before they occur. By analyzing historical data patterns and trends, these technologies can forecast when anomalies are likely to happen, enabling proactive measures to be taken to prevent data quality issues.
Predictions for the Future of Data Quality Monitoring
As organizations grapple with increasingly complex data landscapes, the need for comprehensive data quality monitoring is expected to rise. Future solutions will focus on integrating data quality monitoring seamlessly within the data processing pipelines, leveraging automation and real-time monitoring techniques.
Moreover, the future of data quality monitoring is likely to see a shift towards more personalized and adaptive monitoring solutions. These solutions will be tailored to the specific needs and requirements of each organization, allowing for more targeted and efficient data quality management.
In conclusion, data anomalies and poor data quality have far-reaching implications for organizations. By prioritizing data quality monitoring and developing a robust roadmap, organizations can safeguard the accuracy of their data and make informed decisions with confidence.
You might also like
Contactez-nous pour en savoir plus
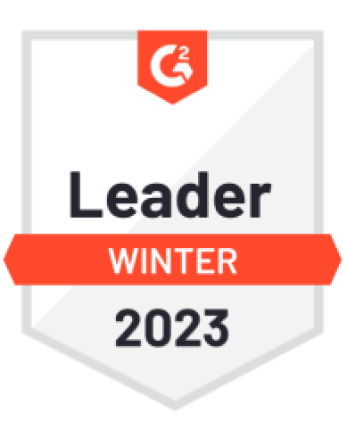
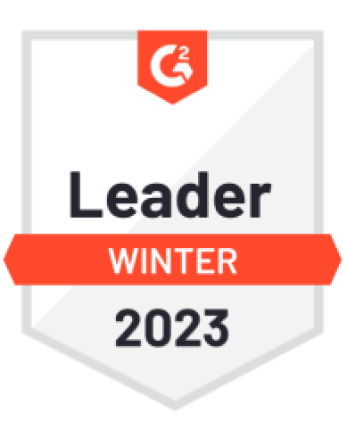

« J'aime l'interface facile à utiliser et la rapidité avec laquelle vous trouvez les actifs pertinents que vous recherchez dans votre base de données. J'apprécie également beaucoup le score attribué à chaque tableau, qui vous permet de hiérarchiser les résultats de vos requêtes en fonction de la fréquence d'utilisation de certaines données. » - Michal P., Head of Data.