Data Profiling: Definition, Techniques, Process, and Examples
Discover the ins and outs of data profiling
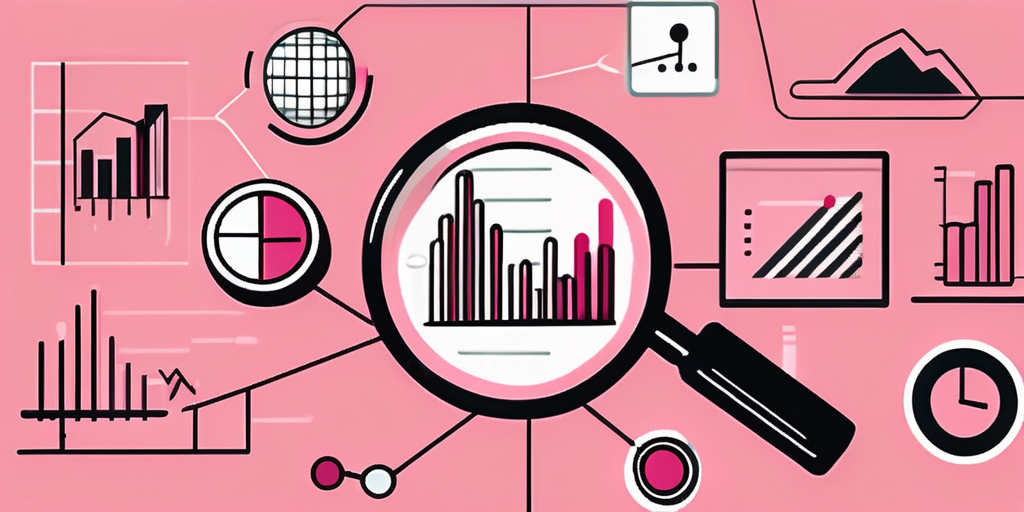
Data profiling plays a crucial role in the field of data analysis and business intelligence. It involves the systematic examination of data to gain a deeper understanding of its quality, structure, and overall content. By uncovering patterns, anomalies, and inconsistencies within datasets, data profiling helps organizations make informed decisions and improve the quality and reliability of their data.
Understanding Data Profiling
Data profiling is an essential step in data management and analytics. It provides insights into the characteristics of data, enabling businesses to identify data issues, rectify errors, and enhance data quality. By closely examining data, organizations can identify data types, data ranges, and data patterns, leading to a comprehensive understanding of their datasets.
The Importance of Data Profiling
Data profiling is imperative for several reasons. Firstly, it helps organizations ensure data accuracy and reliability. By analyzing datasets, businesses can identify and rectify errors, inconsistencies, and duplicates, ultimately improving data quality. This, in turn, leads to better decision-making and reliable business intelligence.
Secondly, data profiling enables businesses to discover data patterns and relationships. By understanding data dependencies, organizations can optimize data storage and identify valuable insights. Additionally, data profiling helps uncover potential risks and vulnerabilities in data, alerting organizations to potential security concerns.
Key Terms and Definitions in Data Profiling
Before delving into the techniques and process of data profiling, it is essential to familiarize ourselves with some key terms. Firstly, data completeness refers to the degree to which data sets are populated with values. Data consistency relates to the reliability and uniformity of data across various sources and instances. Data uniqueness refers to the absence of duplicate entries within a dataset.
Data profiling also involves identifying and understanding data patterns and relationships. Data patterns refer to regularities or trends within data, while data relationships represent the associations between data elements. Lastly, data profiling seeks to identify data anomalies, which are deviations from expected data values or patterns.
Furthermore, data profiling can provide organizations with valuable insights into data distribution. By examining the distribution of data values, businesses can gain a deeper understanding of the spread and concentration of their datasets. This information can be crucial for making informed decisions and optimizing data-driven strategies.
Moreover, data profiling techniques often involve statistical analysis. Through statistical measures such as mean, median, and standard deviation, organizations can quantify and analyze the central tendencies and variabilities within their data. This statistical understanding allows businesses to identify outliers and anomalies that may require further investigation.
Techniques Used in Data Profiling
Several techniques are commonly employed in data profiling. These techniques help organizations gain valuable insights into their datasets and identify areas for improvement. Let's explore some of the prominent techniques used in data profiling:
Column Profiling
Column profiling focuses on analyzing individual columns within a dataset. By examining data values, data types, and data ranges, column profiling provides an understanding of the characteristics of each column. This technique helps identify data quality issues such as missing values, outliers, or inconsistencies.
Column profiling can also reveal data distribution patterns, such as the most common values in a column or the frequency of certain data types. Understanding these patterns is crucial for data cleansing and normalization processes, as it allows organizations to standardize data formats and improve data accuracy.
Dependency Profiling
Dependency profiling aims to uncover relationships and dependencies between different columns or attributes within a dataset. By examining the interactions between data elements, organizations can identify hidden patterns or dependencies that may impact data quality or analysis. Dependency profiling helps enhance data integration and optimize data storage.
Furthermore, dependency profiling can assist in identifying key data drivers or factors that influence certain outcomes. By understanding these dependencies, organizations can make more informed decisions and improve predictive modeling accuracy.
Redundancy Profiling
Redundancy profiling focuses on identifying redundant data within a dataset. Redundant data refers to duplicated or unnecessary data entries that occupy unnecessary storage space. By identifying and eliminating redundant data, organizations can streamline their data storage and improve data efficiency.
In addition to optimizing storage space, redundancy profiling can also lead to improved data processing performance. By reducing the volume of redundant data, organizations can speed up data retrieval and analysis processes, ultimately enhancing overall operational efficiency.
The Process of Data Profiling
The process of data profiling involves several stages that organizations undertake to gain insights into their datasets. Let's explore the various stages of the data profiling process:
Initial Data Assessment
The first stage of data profiling involves a preliminary assessment of the data. Organizations examine the data sources, identify the data elements to profile, and gather necessary metadata. This stage helps define the scope and objectives of the data profiling process.
During the initial data assessment, organizations also evaluate the quality of the data, looking at factors such as completeness, accuracy, consistency, and timeliness. Understanding these aspects is crucial as it sets the foundation for the rest of the data profiling process. Additionally, data profiling tools may be utilized to automate the discovery of data characteristics and provide a comprehensive overview of the dataset.
Data Cleansing and Validation
Once data is assessed, organizations move on to data cleansing and validation. Data cleansing involves identifying and rectifying errors, inconsistencies, and duplicates within the datasets. Validation checks the accuracy and integrity of data, ensuring that it aligns with predefined rules and requirements.
During the data cleansing and validation stage, data profiling teams may employ various techniques such as outlier detection, data transformation, and standardization to enhance the overall quality of the data. By ensuring data cleanliness and accuracy, organizations can minimize the risks associated with making decisions based on flawed or incomplete information.
Final Data Analysis
The final stage of data profiling involves in-depth analysis and interpretation of the data. Organizations explore data patterns, relationships, and anomalies that emerge from the profiling process. This analysis helps identify data issues, improve data quality, and inform decision-making processes.
Moreover, in the final data analysis stage, advanced statistical methods and machine learning algorithms may be employed to uncover hidden insights within the data. By leveraging these sophisticated analytical tools, organizations can extract valuable knowledge from their datasets, enabling them to make informed strategic decisions and gain a competitive edge in their respective industries.
The Role of Data Profiling in Business Intelligence
Data profiling plays a critical role in business intelligence, enabling organizations to derive meaningful insights and make informed decisions. Let's explore some key roles of data profiling in the realm of business intelligence:
Enhancing Decision-Making
Data profiling provides organizations with a comprehensive view of their data, allowing decision-makers to base their judgments on accurate and reliable information. By identifying data quality issues and dependencies, data profiling helps minimize risks and enables strategic decision-making based on reliable data.
Improving Data Quality
Data profiling is instrumental in improving data quality. By identifying data inconsistencies, errors, and redundant data, organizations can rectify and refine their datasets. This leads to enhanced data accuracy and reliability, fostering trust in the data-driven decision-making process.
Facilitating Data Integration
Data profiling helps organizations integrate data from diverse sources. By identifying data dependencies and relationships, organizations can establish connections and linkages between disparate datasets. This integration leads to a holistic view of the data and enables organizations to derive valuable insights.
Moreover, data profiling goes beyond just identifying data issues. It also involves understanding the context and meaning of the data. This contextual understanding allows organizations to uncover hidden patterns, trends, and correlations within the data. For example, by analyzing customer purchase history and demographic data, businesses can identify customer preferences and target specific market segments with personalized offers and recommendations.
Furthermore, data profiling enables organizations to assess the data's fitness for specific purposes. It helps determine if the data is suitable for analysis, reporting, or other business intelligence activities. By evaluating the completeness, consistency, and timeliness of the data, organizations can ensure that their data meets the required standards and is fit for the intended use.
In conclusion, data profiling is a crucial process that organizations undertake to gain a comprehensive understanding of their data. By employing techniques such as column profiling, dependency profiling, and redundancy profiling, businesses can identify data issues and optimize their datasets. Through the stages of initial data assessment, data cleansing and validation, and final data analysis, organizations improve data quality and enhance decision-making processes. Ultimately, data profiling plays a vital role in business intelligence, fostering accurate insights and driving strategic outcomes.
You might also like
Contactez-nous pour en savoir plus
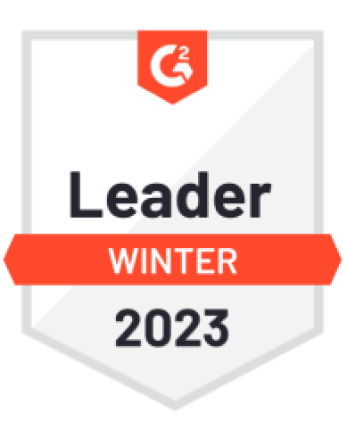
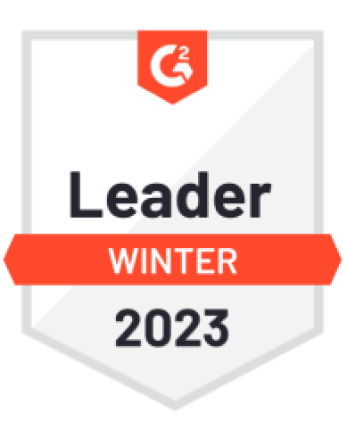

« J'aime l'interface facile à utiliser et la rapidité avec laquelle vous trouvez les actifs pertinents que vous recherchez dans votre base de données. J'apprécie également beaucoup le score attribué à chaque tableau, qui vous permet de hiérarchiser les résultats de vos requêtes en fonction de la fréquence d'utilisation de certaines données. » - Michal P., Head of Data.