Essential Data Quality Measures for Your Business
Learn the essential data quality measures your business needs to implement for accurate and reliable data.

In the digital era, data has become a critical asset for businesses. It is the backbone of decision-making, strategic planning, and operational efficiency. However, the value of data is directly proportional to its quality. Poor data quality can lead to inaccurate insights, misguided strategies, and ultimately, business failure. Therefore, it is crucial for businesses to implement effective data quality measures. In this discussion, we will delve into the essential data quality measures that your business should adopt.
Data Quality Dimensions
Before we delve into the specific measures, it is vital to understand the dimensions of data quality. These dimensions provide a framework for assessing and improving the quality of your data.
The first dimension is accuracy. This refers to whether the data correctly represents the real-world entity or event it is supposed to represent. Inaccurate data can lead to erroneous conclusions and decisions.
Completeness is another critical dimension. It refers to whether all necessary data is present. Missing data can lead to incomplete analysis and insights.
Consistency, the third dimension, refers to whether the data is consistent across all data sources and over time. Inconsistent data can lead to confusion and misinterpretation.
The final dimension is timeliness. This refers to whether the data is available when it is needed. Delayed data can lead to missed opportunities and outdated decisions.
Implementing Data Quality Measures
Now that we understand the dimensions of data quality, let's explore how to implement effective data quality measures.
Data Governance
Data governance is a crucial measure for ensuring data quality. It involves establishing policies, procedures, and standards for data management. This ensures that data is handled consistently and accurately throughout its lifecycle.
Effective data governance involves defining roles and responsibilities for data management, establishing data quality standards, and implementing mechanisms for monitoring and enforcing these standards. It also involves creating a culture of data quality within the organization.
Data Profiling
Data profiling is another essential measure. It involves analyzing the data to identify patterns, anomalies, and inconsistencies. This helps in identifying data quality issues and taking corrective action.
Data profiling involves various techniques such as frequency analysis, pattern matching, and cross-field validation. These techniques help in identifying inaccurate, incomplete, inconsistent, and outdated data.
Data Cleansing
Data cleansing involves correcting or removing inaccurate, incomplete, inconsistent, and outdated data. This ensures that the data is accurate, complete, consistent, and timely.
Data cleansing can be a complex and time-consuming process. It involves various techniques such as data validation, data transformation, and data enrichment. It also requires a deep understanding of the data and its context.
Continuous Data Quality Improvement
Implementing data quality measures is not a one-time activity. It is a continuous process of monitoring, assessing, and improving data quality.
Data Quality Monitoring
Data quality monitoring involves continuously tracking the quality of the data against the established standards. This helps in identifying any deviations and taking corrective action in a timely manner.
Data quality monitoring involves various techniques such as data quality dashboards, data quality reports, and data quality alerts. These tools provide real-time visibility into the quality of the data and enable proactive data quality management.
Data Quality Assessment
Data quality assessment involves periodically evaluating the quality of the data against the established standards. This helps in identifying any gaps and areas for improvement.
Data quality assessment involves various techniques such as data quality audits, data quality surveys, and data quality benchmarks. These techniques provide a comprehensive view of the quality of the data and enable informed decision-making.
Data Quality Improvement
Data quality improvement involves taking corrective action to address the identified data quality issues and enhance the quality of the data. This ensures that the data remains accurate, complete, consistent, and timely.
Data quality improvement involves various techniques such as data cleansing, data enrichment, and data integration. These techniques ensure that the data is clean, complete, consistent, and up-to-date.
Conclusion
In conclusion, data quality is a critical aspect of data management. It is the foundation of reliable insights, informed decisions, and successful strategies. Therefore, businesses should implement effective data quality measures such as data governance, data profiling, data cleansing, data quality monitoring, data quality assessment, and data quality improvement. These measures ensure that the data is accurate, complete, consistent, and timely, thereby maximizing its value and potential.
You might also like
Contactez-nous pour en savoir plus
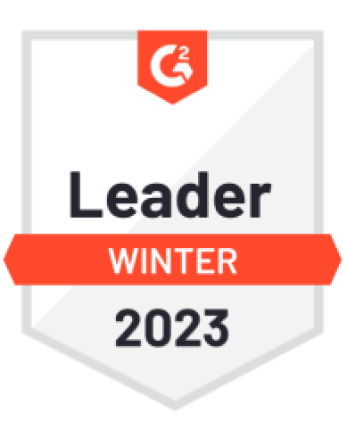
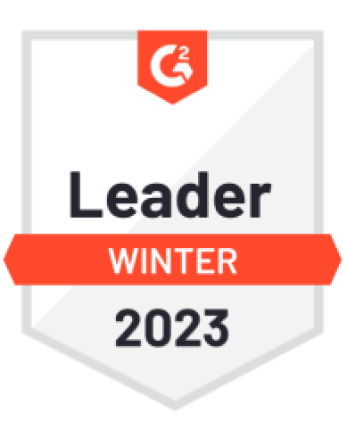

« J'aime l'interface facile à utiliser et la rapidité avec laquelle vous trouvez les actifs pertinents que vous recherchez dans votre base de données. J'apprécie également beaucoup le score attribué à chaque tableau, qui vous permet de hiérarchiser les résultats de vos requêtes en fonction de la fréquence d'utilisation de certaines données. » - Michal P., Head of Data.