Information Science vs Data Science: 5 Key Differences!
Discover the 5 key differences between Information Science and Data Science in this article.
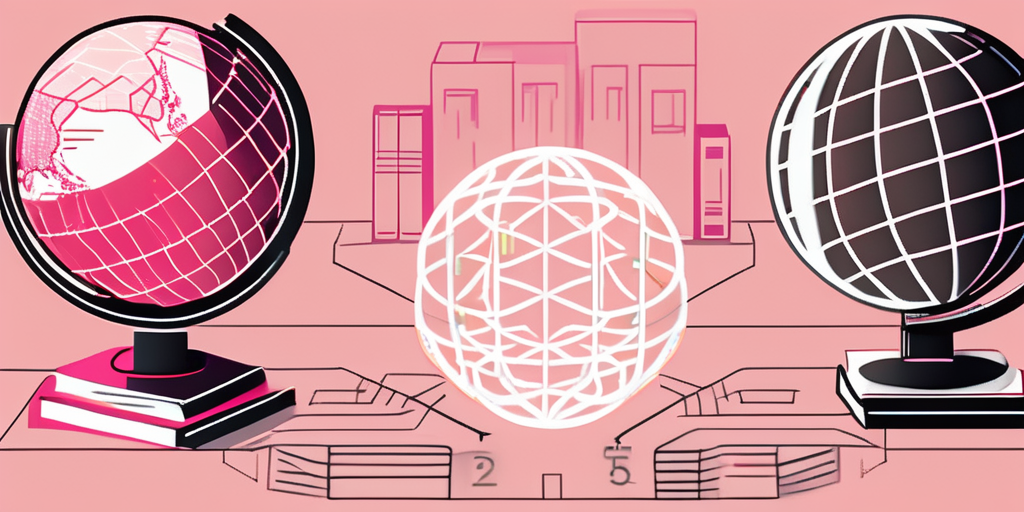
Information Science and Data Science are two rapidly growing fields in today's technology-driven world. While they may seem similar at first glance, there are distinct differences between them that warrant exploration.
Understanding Information Science
Information Science is a multidisciplinary field that focuses on the collection, organization, and retrieval of information. It encompasses various aspects such as information management, knowledge representation, and information architecture. The primary objective of Information Science is to facilitate efficient access to information resources.
One of the key components of Information Science is the study of information behavior, which examines how people seek, use, and share information. Understanding information behavior is essential for designing effective information systems and services that meet the needs of users. Researchers in Information Science often conduct studies to analyze user interactions with information resources and improve the design of information systems based on user feedback.
The Role of Information Science
Information Science plays a crucial role in managing vast amounts of data and converting it into valuable knowledge. It involves studying how information is created, organized, and disseminated across different platforms. Additionally, Information Science explores the impact of information on society, individuals, and organizations.
Another significant aspect of Information Science is information governance, which focuses on establishing policies and procedures for managing information assets within an organization. Information governance ensures that information is accurate, secure, and compliant with regulations. It also involves developing strategies for data quality management, data privacy protection, and risk mitigation in information systems.
Core Concepts in Information Science
Core concepts in Information Science include information retrieval, data modeling, metadata management, and information ethics. These concepts provide a foundation for understanding the principles and processes that govern the organization and dissemination of information.
Information retrieval techniques are essential for enabling users to access relevant information efficiently. Data modeling involves designing structures to represent information in databases and other information systems. Metadata management focuses on creating standardized descriptions of information resources to improve discoverability and interoperability. Information ethics address the ethical considerations surrounding information access, use, and dissemination, ensuring that information practices align with ethical standards and principles.
Delving into Data Science
Data Science, on the other hand, focuses on extracting valuable insights from large, complex datasets. It involves the application of statistical analysis, machine learning algorithms, and data visualization techniques to make informed decisions and predictions.
One of the key aspects of data science is its interdisciplinary nature, drawing from fields such as mathematics, computer science, and domain-specific knowledge. This multidisciplinary approach allows data scientists to tackle a wide range of problems and generate innovative solutions that drive business growth and innovation.
The Function of Data Science
Data Science primarily aims to solve complex problems by uncovering patterns and trends hidden within the data. It leverages statistical and computational techniques to transform raw data into meaningful information. By utilizing advanced algorithms, Data Science enables businesses to extract actionable insights and gain a competitive edge.
Furthermore, data science plays a crucial role in shaping various industries, including healthcare, finance, and marketing. In healthcare, data science is used to analyze patient data and improve treatment outcomes, while in finance, it helps in fraud detection and risk management. Marketing benefits from data science by enabling targeted advertising and personalized customer experiences.
Fundamental Principles of Data Science
At the core of Data Science lie statistical modeling, data exploration, and data engineering. Statistical modeling allows data scientists to identify patterns and relationships within the data, while data exploration involves visualizing the data to gain a deeper understanding. Additionally, data engineering encompasses the processes of data collection, cleaning, and transformation.
Data science also involves ethical considerations, as the insights derived from data can have far-reaching implications on individuals and society as a whole. Ensuring data privacy, transparency, and fairness in algorithms are critical aspects of responsible data science practice. By upholding ethical standards, data scientists can build trust with stakeholders and contribute to positive societal impact.
Difference 1: The Focus of Study
One key difference between Information Science and Data Science lies in their focus of study. Information Science revolves around the management and organization of information, whereas Data Science is centered on extracting insights from data.
Information Science delves into the ways in which information is created, stored, retrieved, and shared. This field encompasses a wide range of topics, including information architecture, knowledge management, and information retrieval systems. Professionals in Information Science often work to ensure that information is accessible, accurate, and secure, playing a crucial role in the design and maintenance of databases, libraries, and digital archives.
On the other hand, Data Science involves the use of statistical and computational techniques to analyze and interpret complex data sets. Data Scientists are skilled in programming languages such as Python and R, as well as in machine learning algorithms. They play a key role in identifying patterns, trends, and correlations within data, helping organizations make data-driven decisions and predictions.
Difference 2: The Tools and Techniques Used
Information Science heavily relies on tools and techniques such as information retrieval systems, database management systems, and data modeling techniques. These tools are essential for organizing, storing, and retrieving vast amounts of information efficiently. Information retrieval systems help users find relevant data quickly by searching through large databases, while database management systems ensure data is stored securely and can be accessed and manipulated as needed. Data modeling techniques are used to create visual representations of data structures, helping to understand complex relationships and patterns within the information.
Data Science, on the other hand, utilizes programming languages such as Python, R, and statistical software like TensorFlow and PyTorch, to analyze and manipulate data effectively. Python is a versatile language commonly used for data manipulation, analysis, and visualization due to its extensive libraries such as Pandas and NumPy. R is another popular language among data scientists, known for its statistical computing capabilities and data visualization tools. TensorFlow and PyTorch are powerful deep learning frameworks that enable the creation and training of complex neural networks for tasks like image recognition and natural language processing.
Difference 3: The Skill Sets Required
Both fields require a solid foundation in mathematics and computer science. However, Information Science emphasizes skills in information management, metadata organization, and knowledge representation, whereas Data Science places a greater emphasis on statistical analysis, machine learning algorithms, and data visualization techniques.
Information Science professionals are often tasked with designing and implementing systems for organizing and accessing large volumes of data efficiently. They need to have a deep understanding of how information is structured, stored, and retrieved to ensure that users can easily find and utilize the information they need. Additionally, proficiency in database management systems and information retrieval techniques is crucial for Information Science specialists to excel in their roles.
On the other hand, Data Science professionals focus on extracting insights and patterns from complex datasets to drive decision-making processes. They are skilled in developing and applying advanced statistical models to analyze data, identify trends, and make predictions. Data Scientists also possess expertise in programming languages such as Python or R, which are commonly used for data manipulation and analysis. Furthermore, they are adept at creating visually appealing data visualizations that effectively communicate findings to stakeholders and facilitate data-driven decision-making.
Difference 4: The Career Opportunities
Information Science offers a wide range of career opportunities, including information architect, data analyst, librarian, or knowledge manager. On the other hand, Data Science opens doors to roles such as data scientist, data engineer, machine learning engineer, or business analyst. The demand for skilled professionals in both fields continues to grow rapidly.
Information architects are responsible for designing the structure and organization of information systems to help users find and manage information more effectively. Data analysts, on the other hand, focus on interpreting data, analyzing results, and providing insights to support decision-making processes within organizations. Librarians play a crucial role in organizing and managing information resources in libraries, ensuring easy access for users and preserving knowledge for future generations. Knowledge managers are tasked with creating, sharing, and utilizing knowledge within organizations to improve efficiency and innovation.
On the Data Science side, data scientists are experts in analyzing and interpreting complex data to help companies make strategic decisions. Data engineers focus on designing and maintaining the infrastructure that allows for the processing of large volumes of data. Machine learning engineers specialize in developing algorithms and models that enable machines to learn and make predictions based on data patterns. Business analysts bridge the gap between data analytics and business strategy, helping organizations make data-driven decisions to achieve their goals.
Difference 5: The Impact on Business Decisions
Information Science primarily focuses on facilitating information access and ensuring its relevance to business operations. In contrast, Data Science empowers businesses to make data-driven decisions by providing insights and predictions that drive growth, enhance customer experience, and optimize processes.
Choosing Between Information Science and Data Science
When deciding between Information Science and Data Science as a career path, several factors need to be considered.
Factors to Consider
It is important to assess your interests, skills, and long-term career goals. If you have a passion for organizing and managing information, Information Science may be the right choice for you. On the other hand, if you possess a strong affinity for data analysis and predictive modeling, Data Science may align better with your aspirations.
Future Trends in Information and Data Science
As technology evolves, both Information Science and Data Science are expected to witness significant advancements. The increasing availability of big data and the continued development of machine learning algorithms are anticipated to shape the future of these fields. It is crucial for professionals in both areas to stay updated with emerging technologies and industry trends.
In conclusion, while Information Science and Data Science share certain similarities, they differ in their focus, tools, skill sets, career opportunities, and impact on business decisions. Choosing the right path depends on individual interests, skills, and aspirations. Regardless of the choice, a career in either field promises exciting opportunities in the rapidly evolving world of information and data.
You might also like
Contactez-nous pour en savoir plus
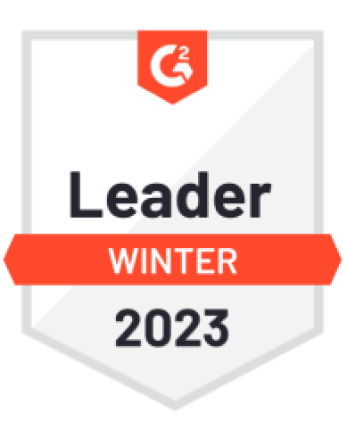
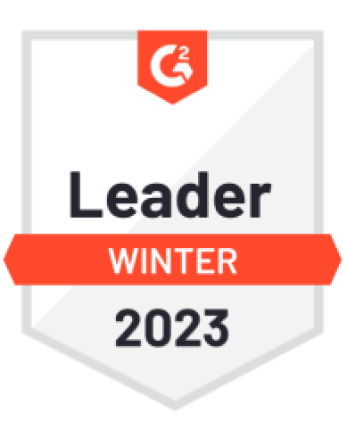

« J'aime l'interface facile à utiliser et la rapidité avec laquelle vous trouvez les actifs pertinents que vous recherchez dans votre base de données. J'apprécie également beaucoup le score attribué à chaque tableau, qui vous permet de hiérarchiser les résultats de vos requêtes en fonction de la fréquence d'utilisation de certaines données. » - Michal P., Head of Data.