Stop Data Anomalies: 5 Effective Strategies to Follow in 2024
Discover the top 5 effective strategies to stop data anomalies in 2024. Learn how to ensure data accuracy and integrity with these approaches.
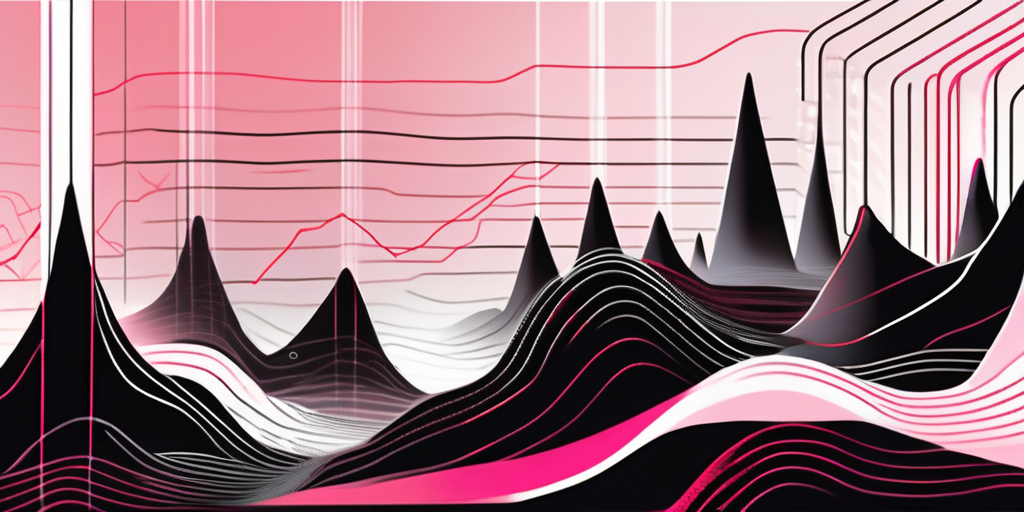
Organizations rely heavily on data for decision-making and business operations. However, data anomalies can significantly impact the reliability and accuracy of these processes. Data anomalies are deviations or abnormalities in data that do not conform to the expected patterns or behavior. Addressing these anomalies is crucial to ensuring data quality and maintaining the integrity of business operations.
Understanding Data Anomalies
Defining Data Anomalies
Data anomalies refer to irregularities or outliers in a dataset that do not fit the overall pattern. These anomalies can be caused by various factors, such as data entry errors, software glitches, or intentional manipulation. Identifying and rectifying these anomalies is essential for organizations to make informed decisions and avoid potential risks.
One common type of data anomaly is known as a "spike," which represents a sudden and extreme increase or decrease in data values. Spikes can distort the analysis of the dataset and lead to misleading conclusions if not properly addressed. Another type of anomaly is a "shift," where the entire dataset is shifted up or down by a constant value, impacting the overall trend analysis.
The Impact of Data Anomalies on Business Operations
Data anomalies can have profound implications on the effectiveness and efficiency of business operations. For instance, inaccurate financial data can lead to incorrect budgeting decisions or misrepresentation of financial statements, potentially resulting in financial losses or legal consequences. Similarly, anomalies in customer data can lead to misguided marketing campaigns or inaccurate customer profiling, negatively impacting customer satisfaction and loyalty.
Moreover, data anomalies can also affect decision-making processes within an organization. If anomalies go undetected, they can influence strategic planning and forecasting, leading to suboptimal outcomes. It is crucial for businesses to implement robust data validation processes and anomaly detection techniques to ensure the accuracy and reliability of their data-driven decisions.
The Importance of Data Quality Management
Role of Data Quality in Decision Making
Data quality management plays a vital role in ensuring reliable, accurate, and consistent data. High-quality data enables organizations to make informed decisions, identify patterns, and generate meaningful insights. By addressing data anomalies, organizations can improve the overall data quality and enhance decision-making processes.
Furthermore, data quality management is crucial for regulatory compliance and risk management. In industries such as healthcare and finance, accurate data is essential for meeting legal requirements and minimizing potential risks. By maintaining high data quality standards, organizations can avoid penalties, protect sensitive information, and build trust with stakeholders.
How Poor Data Quality Leads to Anomalies
Poor data quality is a major contributing factor to data anomalies. Incomplete, inconsistent, or outdated data can introduce errors and deviations that impact the integrity of the overall dataset. Inaccurate data entry, data duplication, or incompatible data formats can all lead to anomalies. By implementing robust data quality management practices, organizations can mitigate the risk of anomalies and maintain high-quality data.
Moreover, poor data quality can also result in operational inefficiencies and increased costs. When organizations rely on inaccurate data for decision-making, it can lead to wasted resources, missed opportunities, and decreased productivity. By investing in data quality management tools and processes, businesses can streamline operations, reduce errors, and optimize performance.
Strategy 1: Implementing Robust Data Validation Techniques
Understanding Data Validation
Data validation is the process of assessing and ensuring the accuracy, completeness, and consistency of data. By validating the data against predefined rules or criteria, organizations can identify and rectify anomalies in real-time. This proactive approach helps maintain data integrity and reduces the impact of anomalies on business operations.
Ensuring data accuracy is crucial for decision-making processes within an organization. Inaccurate data can lead to faulty insights and misguided strategies, ultimately affecting the overall performance and competitiveness of a business. Data validation acts as a safeguard, allowing companies to rely on trustworthy information for critical business decisions.
Key Techniques for Data Validation
Effective data validation techniques involve a combination of manual and automated processes. Manual validation includes verifying data against set criteria, performing data profiling, and validating data relationships. Automated techniques, such as data rule engines and data validation software, can expedite the validation process, detect anomalies, and generate comprehensive reports for further analysis and remediation.
Data profiling plays a vital role in data validation by analyzing the content, structure, and quality of data sets. This process helps in understanding the data distribution, identifying patterns, and uncovering anomalies that may require attention. By gaining insights through data profiling, organizations can tailor their validation strategies to address specific data quality issues effectively.
Strategy 2: Regular Data Auditing
The Need for Regular Data Audits
Data audits are systematic examinations of data to assess its quality, accuracy, and consistency. Regular data audits are essential to identify and address anomalies, detect data discrepancies, and ensure compliance with industry standards and regulations. By conducting audits at regular intervals, organizations can proactively identify potential anomalies and mitigate their impact on business operations.
In addition to the benefits mentioned, regular data audits also play a crucial role in enhancing data security. By consistently reviewing and analyzing data, organizations can identify vulnerabilities and potential security breaches. This proactive approach helps in strengthening data protection measures and safeguarding sensitive information from unauthorized access or cyber threats.
Steps in Conducting a Data Audit
A comprehensive data audit involves several key steps. First, organizations need to define audit objectives and establish audit criteria. Next, they gather and analyze the data, looking for inconsistencies, patterns, and anomalies. Once identified, organizations can validate the anomalies, investigate root causes, and take corrective actions to rectify them. Finally, organizations document the audit findings and implement preventive measures to minimize future anomalies.
Furthermore, conducting a data audit also involves evaluating data governance practices within an organization. This includes assessing how data is collected, stored, managed, and shared across different departments. By reviewing data governance policies and procedures during an audit, organizations can identify areas for improvement and enhance overall data management practices to ensure data integrity and compliance with regulatory requirements.
Strategy 3: Utilizing Automated Anomaly Detection Tools
The Role of Automation in Anomaly Detection
With the increasing volume and complexity of data, manual anomaly detection becomes challenging and time-consuming. Automated anomaly detection tools leverage machine learning algorithms and statistical models to identify unusual patterns or deviations in data. By automating the detection process, organizations can quickly detect anomalies, reduce false positives, and streamline anomaly resolution.
Automated anomaly detection tools can offer a wide range of benefits beyond just identifying anomalies. They can provide real-time monitoring capabilities, allowing organizations to respond swiftly to emerging issues. Additionally, these tools often come with visualization features that help users interpret data patterns more effectively, leading to better decision-making.
Selecting the Right Anomaly Detection Tool
Choosing the appropriate anomaly detection tool is crucial to maximize its effectiveness. Factors to consider include the specific data types, the complexity of anomalies, scalability requirements, and integration capabilities. It is essential to evaluate different tools based on their features, performance, and customization options to ensure a proper fit for the organization's unique needs.
When selecting an anomaly detection tool, organizations should also assess the level of support and training provided by the tool vendor. A robust support system can significantly impact the successful implementation and utilization of the tool. Furthermore, considering the tool's compatibility with existing data infrastructure and the ease of integration into current workflows is key to achieving seamless operations.
By following these strategies, organizations can mitigate the impact of data anomalies and ensure reliable, high-quality data for effective decision-making and streamlined business operations. Proactive data anomaly management contributes to building trust, optimizing resources, and gaining a competitive edge in today's data-centric world.
You might also like
Contactez-nous pour en savoir plus
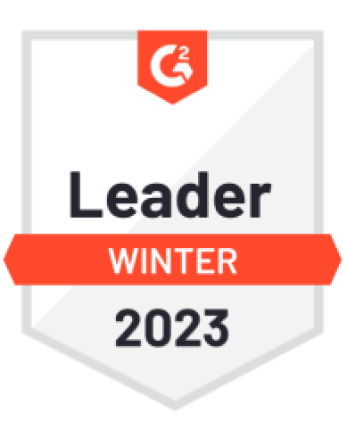
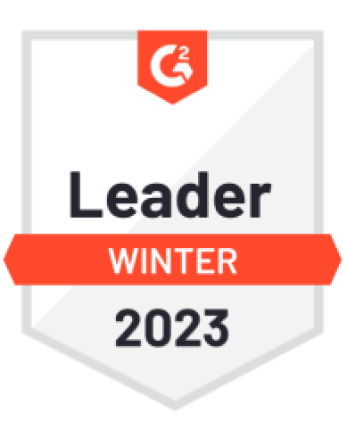

« J'aime l'interface facile à utiliser et la rapidité avec laquelle vous trouvez les actifs pertinents que vous recherchez dans votre base de données. J'apprécie également beaucoup le score attribué à chaque tableau, qui vous permet de hiérarchiser les résultats de vos requêtes en fonction de la fréquence d'utilisation de certaines données. » - Michal P., Head of Data.