What is Data Completeness? Definition and Explanation
Discover the significance of data completeness and its impact on business decisions.
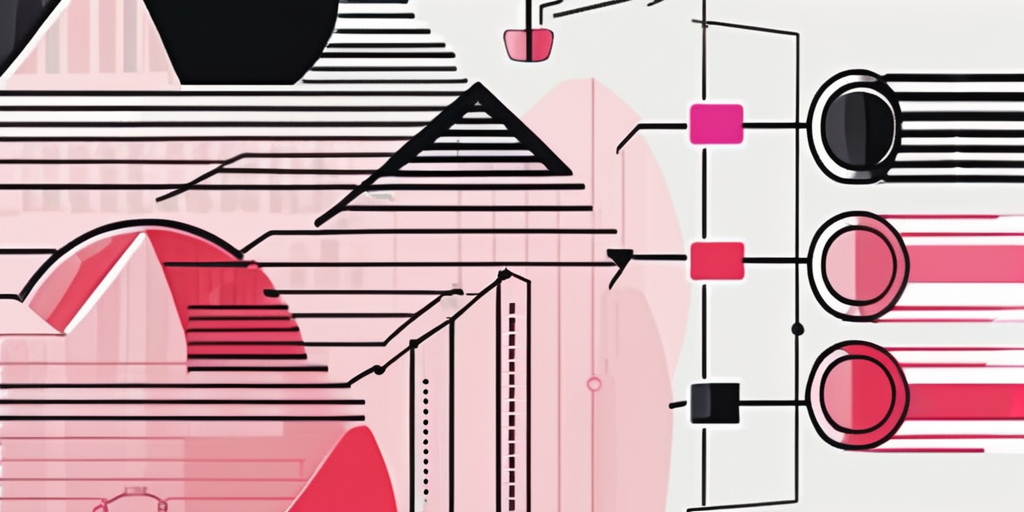
Data completeness is a crucial aspect of data analysis that ensures all necessary information is present and accurate. It refers to the state of having all the required data points or variables within a dataset. In other words, data completeness measures the extent to which data is present, without any gaps or missing values.
Understanding the Concept of Data Completeness
For data to be considered complete, it must contain all the relevant variables and values that are essential for the analysis or decision-making process. Without complete data, there is a risk of drawing inaccurate conclusions or making faulty predictions.
Data completeness is closely related to data quality and plays a vital role in ensuring the validity and reliability of analytical results. Incomplete datasets can introduce biases, hinder pattern recognition, and impede the ability to make informed decisions.
The Importance of Data Completeness in Data Analysis
Data completeness is fundamental to the accuracy and reliability of data analysis. It ensures that all aspects of a problem or situation are adequately represented, allowing for a comprehensive understanding of the subject matter. Without complete data, the findings may lack depth, leading to misleading conclusions.
Moreover, data completeness enables researchers and analysts to identify trends, patterns, and relationships within the dataset. It allows for a holistic view of the data, which can uncover valuable insights and drive evidence-based decision-making.
Key Components of Data Completeness
To achieve data completeness, several key components need to be considered:
- Variables: All relevant variables must be included in the dataset. This involves ensuring that no critical data points are missing or excluded.
- Timeframe: The data should cover the required timeframe to provide a complete picture of the phenomenon being analyzed. This may vary depending on the nature of the analysis.
- Data Integrity: The data should be accurate, reliable, and free from errors. Incomplete datasets with inaccurate or inconsistent values can lead to flawed conclusions.
Another important aspect of data completeness is data consistency. Consistency ensures that the data is uniform and follows a standardized format. Inconsistent data can create confusion and hinder the analysis process. For example, if one variable is measured in pounds and another in kilograms, it can lead to incorrect calculations and misinterpretations.
Furthermore, data completeness also involves considering the source of the data. It is crucial to ensure that the data is collected from reliable sources and is representative of the population or phenomenon being studied. Using data from biased or unrepresentative sources can introduce errors and distort the analysis results.
Lastly, data completeness requires attention to detail and thoroughness in data collection. It is essential to have robust data collection processes in place to minimize the chances of missing or omitting critical variables or values. This may involve implementing data validation checks, conducting regular audits, and ensuring data entry accuracy.
The Role of Data Completeness in Different Industries
Data Completeness in Healthcare
In the healthcare industry, data completeness is crucial for accurate patient diagnosis, treatment planning, and research. Complete patient data, including medical history, test results, and demographic information, is essential for healthcare providers to make informed decisions and provide quality care.
Without complete healthcare data, there is a risk of misdiagnosis, inappropriate treatment, and compromised patient safety. Complete data also facilitates population health management, enabling healthcare organizations to identify trends, track diseases, and implement preventive measures effectively.
Furthermore, data completeness in healthcare plays a significant role in advancing medical research and innovation. Researchers rely on comprehensive patient data to study diseases, develop new treatments, and improve healthcare outcomes. By ensuring data completeness, healthcare institutions contribute to the collective knowledge of the medical community, driving progress and innovation in the field.
Data Completeness in Finance
In the finance industry, data completeness is essential for risk management, regulatory compliance, and investment decision-making. Complete financial data ensures accurate financial reporting, supports auditing processes, and helps identify potential fraud or irregularities.
Complete financial data also allows for effective risk assessment and modeling, enabling financial institutions to make informed investment decisions and manage their portfolios efficiently.
Moreover, data completeness in finance is crucial for market transparency and investor confidence. By maintaining complete and accurate financial records, companies and financial institutions build trust with investors and stakeholders, fostering a stable and reliable financial environment. Transparent financial data also enhances market efficiency, enabling investors to make well-informed decisions and promoting healthy competition within the financial sector.
Measuring Data Completeness
Ensuring data completeness is a crucial aspect of data management, as it directly impacts the reliability and accuracy of analytical insights derived from the data. By measuring data completeness, organizations can identify gaps in their datasets and take necessary actions to improve data quality.
Tools for Assessing Data Completeness
Various tools and techniques can be employed to assess data completeness effectively:
- Data Quality Dashboards: These interactive dashboards offer real-time insights into data completeness metrics, enabling users to track the completeness levels of their datasets effortlessly. Visual representations such as progress bars, pie charts, and heat maps make it easier to identify incomplete data points and prioritize data enhancement efforts.
- Data Profiling Tools: Advanced data profiling tools utilize algorithms to scan datasets for missing values, duplicates, and inconsistencies. By generating comprehensive data quality reports, these tools empower data stewards to address data completeness issues proactively.
- Data Completeness Scorecards: Scorecards provide a quantitative measure of data completeness, assigning scores based on the presence or absence of critical data elements. These scores help organizations set benchmarks, track improvements over time, and compare data completeness levels across different datasets.
Common Challenges in Measuring Data Completeness
Despite the availability of tools and best practices, measuring data completeness can be fraught with challenges:
- Data Sources: Integrating data from disparate sources poses a significant challenge to data completeness efforts. Variations in data formats, structures, and definitions across sources can result in incomplete datasets. Establishing data governance policies and standardizing data formats are essential steps to address this challenge.
- Data Entry Errors: Human errors during data entry, such as typos, missing values, or outdated information, can compromise data completeness. Implementing data validation rules, automated data cleansing processes, and user training programs can help minimize the impact of data entry errors on data completeness.
- Technological Limitations: Legacy systems and outdated data management tools may lack robust features for assessing data completeness effectively. Investing in modern data integration platforms and data quality solutions can enhance the organization's ability to measure and improve data completeness.
Strategies to Improve Data Completeness
Best Practices for Data Collection
Implementing the following best practices can improve data completeness:
- Standardized Data Collection Processes: Establishing standardized processes and guidelines for data collection ensures consistency and reduces the risk of missing data.
- Automated Data Validation: Implementing automated data validation checks during data entry minimizes errors and improves data completeness.
Furthermore, it is essential to train staff members on the importance of accurate data collection and provide ongoing support to ensure compliance with data collection protocols. Regular audits and reviews of data collection processes can also help identify areas for improvement and ensure data completeness is maintained over time.
The Role of Data Governance in Ensuring Completeness
Data governance plays a crucial role in ensuring data completeness. Data governance frameworks and policies define data requirements, standards, and quality objectives. They provide guidelines for data collection and maintenance, making data completeness an integral part of organizational data management practices.
Effective data governance also involves establishing clear roles and responsibilities for data stewards who oversee data quality and completeness. These individuals are responsible for monitoring data collection processes, resolving data quality issues, and ensuring that data remains accurate and complete throughout its lifecycle.
The Impact of Incomplete Data on Business Decisions
Risks Associated with Incomplete Data
Businesses relying on incomplete data face several risks:
- Decision-Making Bias: Incomplete data can lead to biased decision-making as critical aspects of the problem may be omitted or misrepresented.
- Missed Opportunities: Incomplete data can result in missed business opportunities, as crucial insights and trends may remain undiscovered.
Moreover, incomplete data can also lead to inefficiencies in resource allocation. Without a comprehensive understanding of the situation, businesses may invest in the wrong areas or overlook areas that require attention. This can ultimately impact the bottom line and hinder overall business growth.
Overcoming the Consequences of Incomplete Data
Despite the challenges, there are strategies businesses can employ to overcome the consequences of incomplete data:
- Data Integration: Integrating data from various sources can help fill gaps and improve data completeness. However, this requires careful data mapping and alignment.
- Data Imputation: When faced with missing data points, data imputation techniques can be used to estimate values based on existing data or statistical analysis.
Additionally, establishing clear data collection protocols and ensuring data quality checks are in place can help prevent incomplete data from entering the system. Regular audits and reviews of data collection processes can identify gaps and inconsistencies, allowing businesses to take corrective actions promptly.
In conclusion, data completeness is a critical element in data analysis across different industries. It ensures the accuracy and reliability of analytical results, enables informed decision-making, and helps organizations uncover valuable insights. By understanding the concept of data completeness, measuring it effectively, and implementing strategies to improve completeness, businesses can mitigate the risks associated with incomplete data and maximize the value of their data assets.
You might also like
Contactez-nous pour en savoir plus
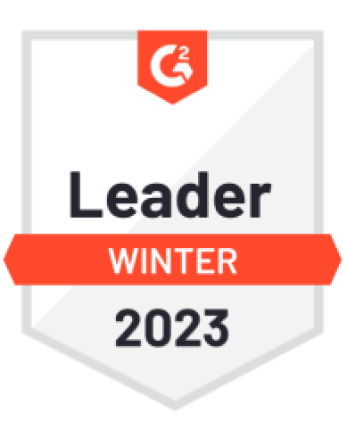
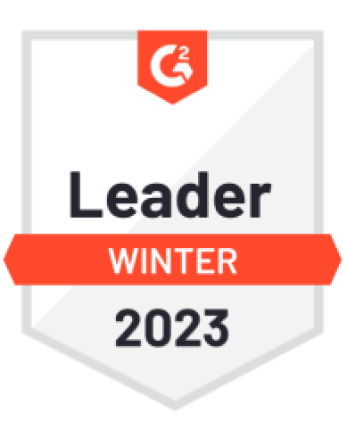

« J'aime l'interface facile à utiliser et la rapidité avec laquelle vous trouvez les actifs pertinents que vous recherchez dans votre base de données. J'apprécie également beaucoup le score attribué à chaque tableau, qui vous permet de hiérarchiser les résultats de vos requêtes en fonction de la fréquence d'utilisation de certaines données. » - Michal P., Head of Data.