What Is Data Quality And Why Is It Important?
Learn about the significance of data quality and its impact on businesses.

From customer information to sales transactions, data plays a crucial role in every aspect of business operations. However, not all data is created equal. There is a stark difference between high-quality data and poor-quality data, and this difference can have significant implications for organizations. In this article, we will delve into the concept of data quality, its importance, how it can be measured, strategies for improving it, and the challenges that organizations face in maintaining data quality.
Understanding the Concept of Data Quality
Data quality refers to the accuracy, completeness, consistency, and relevancy of data. In simple terms, it is about having trustworthy and reliable information that can be used for decision-making and analysis. Whether it is demographic data, sales figures, or inventory data, maintaining high-quality data is essential for organizations to extract meaningful insights and drive business success.
Definition of Data Quality
Defining data quality can be a complex task as it varies depending on the context and requirements of each organization. However, at its core, data quality can be measured by its fitness for use in a given environment or system. In other words, data is of high quality if it meets the specific needs and expectations of the users.
Key Components of Data Quality
Data quality is multidimensional and encompasses various aspects. The key components of data quality include:
- Accuracy: Data should be precise, free from errors, and reflect the true values or attributes it represents. Inaccurate data can lead to incorrect analyses and flawed decision-making.
- Completeness: Data should be comprehensive and include all relevant information. Incomplete data can hinder the ability to gain a holistic view of the subject matter.
- Consistency: Data should be consistent across different sources, systems, and time periods. Inconsistencies can arise from data entry errors, system integration issues, or duplications, leading to confusion and unreliable insights.
- Relevancy: Data should be relevant to the purpose for which it is collected or analyzed. Irrelevant data can clutter databases, complicate decision-making, and waste valuable resources.
Ensuring data accuracy involves implementing robust data validation processes, such as double-entry verification and automated error detection algorithms. These measures help identify and rectify any discrepancies or inconsistencies in the data, ensuring that the information is reliable and trustworthy.
Furthermore, data completeness can be achieved by establishing clear data collection protocols and ensuring that all relevant data points are captured and recorded accurately. Regular data audits and quality checks can help identify any missing or incomplete data, allowing organizations to take corrective actions and maintain a comprehensive dataset.
Consistency in data can be maintained through standardized data formats, naming conventions, and data integration practices. By establishing clear guidelines and protocols for data entry and integration, organizations can minimize the risk of inconsistencies and ensure that data remains reliable and consistent across different sources and systems.
Lastly, data relevancy can be ensured by aligning data collection efforts with the specific goals and objectives of the organization. By clearly defining the purpose and scope of data collection, organizations can avoid unnecessary data clutter and focus on gathering information that is directly relevant to their decision-making processes.
The Importance of High-Quality Data
The significance of high-quality data cannot be understated. It serves as the foundation for effective decision-making, informed strategies, and accurate analysis. Let's explore how data quality plays a vital role in two key areas: decision making and business performance.
High-quality data is not just about having a large volume of information; it's about ensuring that the data is accurate, relevant, and up-to-date. In today's fast-paced business environment, decisions need to be made swiftly and confidently. This is where the importance of data quality shines through. Imagine a scenario where a company is considering launching a new product line. By analyzing high-quality data on market trends, consumer preferences, and competitor strategies, decision-makers can assess the viability of the new product with confidence. On the other hand, relying on outdated or inaccurate data could result in a costly misstep that impacts the company's bottom line.
Role of Data Quality in Decision Making
When making critical business decisions, leaders rely on data to guide them. Data quality ensures that decision-makers have confidence in the information they are basing their choices on. By having accurate and reliable data at their disposal, organizations can make well-informed decisions that drive growth, mitigate risks, and capitalize on opportunities. On the other hand, poor-quality data can lead to misguided decisions, missed opportunities, and costly errors.
Moreover, high-quality data fosters a culture of data-driven decision-making within an organization. When employees trust the data they are working with, they are more likely to rely on data insights to support their proposals and initiatives. This not only streamlines decision-making processes but also promotes a more collaborative and transparent work environment.
Impact of Data Quality on Business Performance
Data quality directly impacts business performance and outcomes. High-quality data enables organizations to analyze trends, identify patterns, and spot opportunities for improvement. It provides a solid foundation for forecasting, predicting customer behavior, and optimizing operations. On the contrary, poor data quality can lead to misguided strategies, wasted resources, and missed revenue-generating opportunities.
Furthermore, in today's data-driven economy, the quality of data can also impact customer satisfaction and loyalty. By maintaining accurate customer data, businesses can personalize their marketing efforts, improve customer service, and build long-lasting relationships with their clientele. This personalized approach not only enhances the customer experience but also drives repeat business and referrals, ultimately boosting the company's bottom line.
Measuring Data Quality
Accurately measuring data quality is essential for understanding the current state of data and identifying areas that require improvement. Let's explore the criteria for assessing data quality and common tools used for measurement.
Criteria for Assessing Data Quality
When assessing data quality, several criteria need to be considered:
- Validity: The extent to which data adheres to the defined data models, validation rules, and constraints.
- Consistency: The degree of agreement between data elements within and across databases or systems.
- Completeness: The extent to which data contains all the required attributes and relevant information.
- Timeliness: The degree to which data is up-to-date and available within the required timeframe.
- Accuracy: The level of correctness or preciseness of the data values.
Common Tools for Data Quality Measurement
Several tools are available to measure data quality, ranging from manual methods to automated software solutions. These tools help organizations establish data quality baselines, identify data issues, and track data quality improvements over time. Some commonly used tools include:
- Data profiling tools: These tools analyze data to discover patterns, anomalies, and data quality issues.
- Data cleansing tools: These tools automate the process of identifying and correcting data errors, inconsistencies, and redundancies.
- Data validation tools: These tools verify whether data adheres to predefined rules and constraints.
- Data quality monitoring tools: These tools continuously monitor data quality in real-time, alerting organizations to any anomalies or issues.
Strategies for Improving Data Quality
Improving data quality is an ongoing process that requires a proactive approach and adherence to best practices. Let's explore some strategies that organizations can implement to enhance data quality.
Best Practices for Data Collection
The quality of data starts at the point of collection. To ensure high-quality data, organizations should consider the following best practices:
- Standardize data entry: Implement standardized data entry procedures and formats to minimize errors and inconsistencies.
- Validate data at the point of entry: Implement validation checks to ensure the accuracy and completeness of data during the data entry process.
- Train data collectors: Provide comprehensive training to individuals responsible for data collection to ensure consistency and accuracy in data capture.
- Implement data governance: Establish data governance frameworks and policies to enforce data quality standards and accountability.
Techniques for Data Cleaning and Validation
Data cleaning and validation are essential steps in improving data quality. Consider the following techniques:
- Remove duplicate records: Identify and eliminate duplicate records to avoid data redundancy and improve accuracy.
- Standardize and format data: Apply data standardization techniques to ensure consistent data formats and eliminate inconsistencies.
- Conduct data profiling: Use data profiling techniques to identify anomalies, outliers, and data quality issues.
- Implement automated validation rules: Utilize validation rules to identify and correct data quality issues automatically.
Challenges in Maintaining Data Quality
While organizations strive for high-quality data, they often face several challenges in maintaining data quality. Let's explore two common challenges that organizations encounter:
Issues in Data Integration
Organizations often have data stored in various systems and databases, making data integration a challenging task. Data integration issues can result in data inconsistencies, conflicts, and inaccuracies. It requires careful planning, robust data integration strategies, and governance frameworks to ensure data integrity and quality during the integration process.
Dealing with Incomplete or Inaccurate Data
Incomplete or inaccurate data can significantly impact the reliability and usability of information. Missing data elements or incorrect entries can skew analyses and lead to erroneous insights. Organizations need to implement data validation checks, establish data quality controls, and leverage automated data cleansing techniques to manage incomplete or inaccurate data.
Conclusion
Data quality is of utmost importance in today's data-driven world. High-quality data enables organizations to make informed decisions, drive business performance, and gain a competitive edge. By understanding the concept of data quality, measuring it accurately, implementing strategies to improve it, and overcoming challenges in maintaining it, organizations can harness the power of data to unlock new opportunities and achieve sustainable growth.
Understanding the importance of data quality is just the beginning. To truly harness the power of your data and ensure it's working for you, it's essential to have the right tools in place. CastorDoc is designed to elevate your data governance and analytics to the next level, integrating advanced governance capabilities with a user-friendly AI assistant to streamline self-service analytics. With CastorDoc, you can confidently manage your data's quality, compliance, and utilization, empowering both data teams and business users alike. Don't let data quality challenges hold you back. Try CastorDoc today and unlock the full potential of your data to drive informed decision-making and foster sustainable growth.
You might also like
Contactez-nous pour en savoir plus
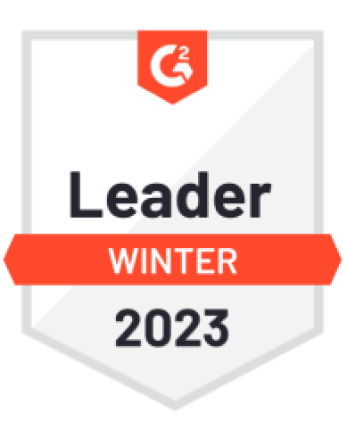
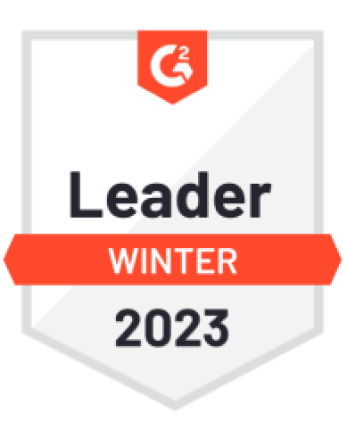

« J'aime l'interface facile à utiliser et la rapidité avec laquelle vous trouvez les actifs pertinents que vous recherchez dans votre base de données. J'apprécie également beaucoup le score attribué à chaque tableau, qui vous permet de hiérarchiser les résultats de vos requêtes en fonction de la fréquence d'utilisation de certaines données. » - Michal P., Head of Data.