Data Warehouse Tool Comparison: BigQuery vs. Teradata
In this article, we will delve into the world of data warehousing tools and compare two prominent ones: BigQuery and Teradata. To make an informed decision about which tool is right for your business, it's vital to understand the fundamentals of data warehousing, as well as the key features and advantages each tool offers.
Understanding Data Warehousing
Data warehousing is an essential component of modern business intelligence. It involves the process of collecting, organizing, and analyzing vast amounts of data from various sources to provide meaningful insights for decision-making. A data warehouse acts as a central repository, storing data in a structured format and optimizing it for reporting and analysis.
The Role of Data Warehousing in Business
Data warehousing plays a crucial role in enabling organizations to make data-driven decisions. By consolidating data from disparate sources, companies can gain a holistic view of their operations, customers, and market trends. This comprehensive understanding empowers decision-makers to identify patterns, trends, and opportunities that drive business growth and innovation.
Key Features of a Good Data Warehouse Tool
When evaluating data warehouse tools like BigQuery and Teradata, several key features must be considered:
- Scalability: The ability to handle large volumes of data and seamlessly scale as business needs grow.
- Performance: Efficient data processing and query execution to ensure fast and reliable analysis.
- Cost-effectiveness: Balancing the tool's capabilities with the costs involved in implementation, maintenance, and utilization.
- Security: Robust security measures to protect sensitive data from unauthorized access or breaches.
Scalability is a critical factor to consider when choosing a data warehouse tool. As businesses grow and expand, the amount of data they generate and need to analyze increases exponentially. A good data warehouse tool should be able to handle this growth without compromising performance. It should have the capability to scale seamlessly, allowing businesses to add more storage and processing power as needed, without disrupting ongoing operations.
Performance is another crucial aspect to evaluate. In today's fast-paced business environment, decision-makers need real-time insights to make informed choices. A data warehouse tool should be able to process and analyze data quickly, enabling users to run complex queries and generate reports in a timely manner. This ensures that decision-makers have access to up-to-date information, empowering them to make agile and effective decisions.
Cost-effectiveness is also an important consideration. Implementing and maintaining a data warehouse can be a significant investment for any organization. Therefore, it is essential to choose a tool that provides the right balance between capabilities and costs. While some tools may offer advanced features, they may come with a higher price tag. It is crucial to assess the specific needs of the business and choose a tool that meets those requirements without exceeding the budget.
Lastly, security is of utmost importance when dealing with sensitive data. A good data warehouse tool should have robust security measures in place to protect against unauthorized access, data breaches, and other security threats. This includes features such as encryption, access controls, and regular security audits. By ensuring the security of the data warehouse, organizations can maintain the trust of their customers and stakeholders, and comply with relevant data protection regulations.
Introduction to BigQuery
BigQuery, developed by Google, is a fully managed, serverless data warehouse tool designed for big data analytics. With its cloud-native architecture, BigQuery offers several unique advantages for organizations seeking high scalability and performance.
Organizations across various industries are increasingly turning to BigQuery for its robust capabilities in handling massive datasets and complex analytical queries. The platform's ability to effortlessly scale resources based on demand, without the need for manual intervention, provides users with a seamless and efficient data processing experience.
Overview of BigQuery
BigQuery utilizes a distributed, columnar storage system that enables lightning-fast, parallel query execution. Its powerful SQL-based querying capabilities and seamless integration with other Google Cloud services make it a popular choice among enterprises and data professionals.
Furthermore, BigQuery's architecture is designed to ensure data reliability and security, with built-in encryption mechanisms and access controls. This focus on data protection is crucial for organizations dealing with sensitive information and regulatory compliance requirements, making BigQuery a trusted solution for data analytics.
Key Features of BigQuery
Some noteworthy features of BigQuery include:
- Automatic Scaling: BigQuery automatically adjusts resources to handle queries of any size, ensuring consistent performance.
- Cost Optimization: Google's flexible pricing model allows organizations to pay only for the data and processing capacity they need, reducing overall costs.
- Real-time Data Analysis: BigQuery supports real-time data ingestion, enabling timely and accurate insights from streaming data sources.
- Advanced Analytics: The integration of machine learning capabilities, such as BigQuery ML, enhances predictive modeling and data analysis within the tool itself.
Moreover, BigQuery's seamless integration with popular business intelligence tools like Tableau and Looker empowers users to visualize and explore data insights in a user-friendly manner. This interoperability with external applications enhances the overall data analytics workflow, enabling organizations to derive actionable insights from their data more effectively.
Introduction to Teradata
Teradata is a well-established data warehousing platform known for its impressive performance and scalability. It caters to organizations that require robust solutions to handle their data-intensive workloads. With a history dating back to the 1980s, Teradata has continuously evolved to meet the changing needs of the data analytics industry.
Teradata's commitment to innovation and customer satisfaction has solidified its position as a leader in the data warehousing market. Its platform is trusted by numerous enterprises worldwide, spanning various industries such as finance, healthcare, retail, and telecommunications.
Overview of Teradata
Teradata's architecture combines a parallel processing framework with shared-nothing architecture, enabling efficient data retrieval and processing across multiple nodes. This distributed approach allows Teradata to seamlessly scale to petabyte-sized data warehouses, accommodating the ever-growing data volumes of modern businesses.
Furthermore, Teradata's architecture is designed for high availability and fault tolerance, ensuring continuous operations even in the face of hardware failures or network issues. This reliability is crucial for organizations that rely on real-time data analytics to drive their decision-making processes.
Key Features of Teradata
Teradata brings several compelling features to the table, making it a preferred choice for enterprises seeking a powerful data warehousing solution:
- Massive Parallel Processing: Teradata's MPP architecture ensures high-performance data retrieval and analytics, even with vast amounts of data. By distributing workloads across multiple nodes, Teradata can deliver rapid query responses and support complex analytical queries.
- Optimized Workload Management: Resource allocation and workload balancing features allow organizations to achieve optimal performance across different workloads. Teradata's workload management capabilities help prioritize critical tasks and ensure that resources are efficiently utilized.
- Advanced In-Database Analytics: Teradata's integrated analytics capabilities enable powerful analysis and modeling within the database itself, reducing data movement and enhancing efficiency. This feature accelerates insights generation and simplifies the analytics workflow for data scientists and analysts.
- Strong Data Security: Teradata prioritizes data security, offering a comprehensive set of measures to protect sensitive information. From encryption and access controls to auditing and compliance features, Teradata ensures that data remains secure throughout its lifecycle.
Comparing BigQuery and Teradata
Now that we have a good understanding of both tools, let's compare them across different aspects that are crucial for organizations considering a data warehouse solution.
Performance Comparison
When it comes to performance, both BigQuery and Teradata shine in their respective domains. BigQuery's columnar storage and distributed computing infrastructure deliver exceptional query response times, especially for complex analytical queries. On the other hand, Teradata's parallel processing architecture allows it to handle massive workloads, making it an ideal choice for data-intensive environments.
Cost Comparison
Cost is an important consideration for any organization. BigQuery's pay-as-you-go pricing model, combined with its automatic scaling, provides cost optimization by only charging for the resources consumed. Teradata, being an on-premises solution, requires substantial upfront investment in hardware, licensing, and maintenance. While it offers long-term cost savings for large-scale deployments, it may not be the most cost-effective option for smaller businesses or those with fluctuating workloads.
Scalability Comparison
Scalability is a significant factor for businesses anticipating growth or experiencing unpredictable fluctuations in data volumes. BigQuery's cloud-native architecture allows it to scale effortlessly, adding computing resources as needed, making it highly adaptable to changing demands. Teradata, although flexible, requires careful planning and resource provisioning to accommodate scalability, which may involve additional expenses and administrative efforts.
Security Comparison
Both BigQuery and Teradata prioritize data security. BigQuery benefits from Google Cloud's robust security infrastructure, which includes encryption, access controls, and data segregation. Teradata, on the other hand, offers extensive security features such as robust authentication mechanisms, end-to-end data encryption, and comprehensive audit logging capabilities. The choice between the two would depend on specific security requirements and compliance obligations of the organization.
Choosing the Right Data Warehouse Tool
Choosing between BigQuery and Teradata ultimately depends on your specific business needs and priorities. Here are some factors to consider:
Factors to Consider
1. Data Volume: Evaluate the scale of your data and whether it requires a cloud-based solution like BigQuery or an on-premises option like Teradata.
2. Budget: Consider the monetary implications of both short-term and long-term investments, factoring in hardware costs, licensing fees, and operational expenses.
3. Use Case Complexity: Analyze the complexity of your analytical workloads and determine which tool can provide the most efficient and insightful analysis for your specific use cases.
4. Integration Capabilities: Assess the ease of integration with your existing systems and other tools in your data ecosystem.
Making the Decision
When making the final decision, involve your data engineering and analytics teams to gain insights into their requirements and preferences. Consider conducting a proof-of-concept to evaluate the performance, scalability, and cost-effectiveness of both tools in your specific environment before committing to a long-term contract or investment.
Remember, the data warehouse tool you choose will play a crucial role in shaping your organization's data-driven decisions and future growth. Taking the time to thoroughly analyze your requirements and evaluate the features of tools like BigQuery and Teradata will ensure you make an informed decision that maximizes your return on investment and accelerates your journey towards deriving valuable insights from your data.
As you consider the pivotal role of data warehousing tools like BigQuery and Teradata in your organization's growth, don't overlook the importance of effective data governance and accessibility. CastorDoc is designed to complement your data warehouse solution by integrating advanced governance, cataloging, and lineage capabilities with a user-friendly AI assistant. This powerful combination enables self-service analytics, allowing your teams to manage, understand, and utilize data with unprecedented ease and efficiency. Whether you're looking to enhance data visibility for your data professionals or empower business users through intuitive access, CastorDoc is your partner in unlocking the full potential of your data. To explore more tools comparisons and discover how CastorDoc can transform your data strategy, check out more tools comparisons here.
You might also like
Contactez-nous pour en savoir plus
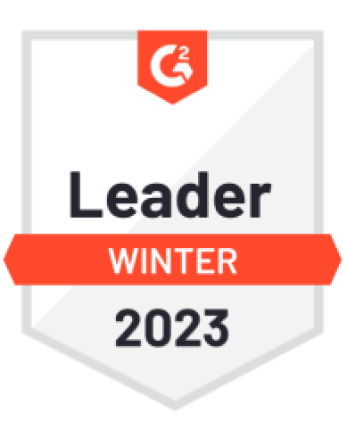
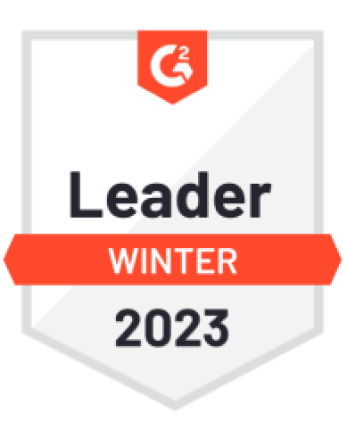

« J'aime l'interface facile à utiliser et la rapidité avec laquelle vous trouvez les actifs pertinents que vous recherchez dans votre base de données. J'apprécie également beaucoup le score attribué à chaque tableau, qui vous permet de hiérarchiser les résultats de vos requêtes en fonction de la fréquence d'utilisation de certaines données. » - Michal P., Head of Data.