Data Observability Tool Comparison: Datafold vs. Marquez
In this article, we will be delving into the world of data observability tools and comparing two popular options: Datafold and Marquez. Data observability has become increasingly important in today's data-driven world. It involves monitoring, measuring, and ensuring the quality and reliability of data pipelines and workflows. Let's explore why data observability is crucial and examine the key features of these two tools.
Understanding Data Observability
Data observability refers to the ability to gain insights into the health, performance, and accuracy of data pipelines. It enables data teams to track and identify issues and anomalies in their data workflows promptly. By implementing data observability practices, organizations can ensure data reliability and make better-informed decisions based on trustworthy insights.
The Importance of Data Observability
Data observability is essential in guaranteeing the quality of data pipelines and workflows. It ensures that data is accurate, consistent, and reliable, ultimately boosting confidence in data-driven decisions. Organizations heavily rely on data visibility to prevent costly errors, improve operational efficiency, and enhance customer experiences.
Key Features of Data Observability Tools
Data observability tools come equipped with various features that aid in monitoring, maintaining, and troubleshooting data pipelines. Some of the key features to look for include:
- Data Profiling: Providing insights into data schema, structure, and quality metrics.
- Data Lineage: Tracking the origin and transformation of data throughout the pipeline.
- Data Quality Monitoring: Detecting anomalies, outliers, and errors in the data.
- Data Drift Detection: Identifying changes in the statistical properties of data over time.
- Alerts and Notifications: Sending real-time alerts and notifications when issues arise.
Data profiling is a crucial aspect of data observability. It allows data teams to gain a deep understanding of the data they are working with. By analyzing the data schema, structure, and quality metrics, data profiling provides valuable insights that help identify potential issues or inconsistencies. This information is vital for ensuring data accuracy and reliability throughout the pipeline.
Data lineage is another key feature of data observability tools. It enables organizations to track the journey of data from its origin to its destination, including all the transformations it undergoes along the way. By visualizing the data lineage, data teams can easily identify any bottlenecks or issues that may arise during the data flow. This helps in troubleshooting and maintaining the data pipelines effectively.
In addition to data profiling and data lineage, data quality monitoring is an essential feature of data observability tools. It involves continuously monitoring the data for anomalies, outliers, and errors. By detecting and addressing these issues in real-time, organizations can ensure that the data being used for decision-making is accurate and reliable. This helps in preventing costly errors and maintaining the overall quality of the data pipelines.
Data drift detection is another valuable feature offered by data observability tools. It helps organizations identify changes in the statistical properties of data over time. By monitoring data drift, data teams can detect shifts in data patterns or distributions, which may indicate underlying issues or changes in the data source. This proactive approach allows organizations to address potential problems before they impact data accuracy and reliability.
Lastly, alerts and notifications play a crucial role in data observability. Real-time alerts and notifications keep data teams informed about any issues or anomalies that occur in the data pipelines. This enables them to take immediate action and resolve the problems promptly, minimizing any potential disruptions or negative impacts on data-driven decision-making processes.
In conclusion, data observability is a critical practice for organizations that rely on data-driven decision-making. By implementing data observability tools with features such as data profiling, data lineage, data quality monitoring, data drift detection, alerts, and notifications, organizations can ensure the accuracy, reliability, and performance of their data pipelines. This ultimately leads to better-informed decisions, improved operational efficiency, and enhanced customer experiences.
An Introduction to Datafold
Datafold is a comprehensive data observability tool that empowers data teams to achieve data reliability and streamline their data workflows. Let's explore datafold further with a brief overview.
Datafold provides end-to-end visibility into data pipelines, offering deep insights into data quality, schema changes, and data drift. It integrates seamlessly with modern data stacks and supports a range of data systems, including relational databases, data warehouses, and data lakes. With Datafold, data teams can easily identify and resolve issues, ensuring the accuracy and reliability of their data-driven initiatives.
But what sets Datafold apart from other data observability tools? Let's dive into some key features that make Datafold a game-changer in the industry.
Data Profiling: Comprehensive Insights into Your Data
Data profiling is a critical aspect of data observability, and Datafold excels in this area. With its comprehensive data profiling capabilities, Datafold enables data teams to gain deep insights into their data. From understanding the schema and data types to assessing data quality, Datafold provides a holistic view of your data. It helps you identify potential issues, such as missing values, outliers, or inconsistent data, allowing you to take proactive measures to ensure data accuracy.
Data Lineage Visualization: Unravel the Journey of Your Data
Have you ever wondered how your data flows and transforms throughout your pipeline? Datafold's data lineage visualization feature has got you covered. With clear and intuitive visualizations, you can easily track the journey of your data, from its source to its destination. This helps you understand the dependencies and transformations applied to your data, enabling you to troubleshoot issues and optimize your data workflows. Data lineage visualization in Datafold is a powerful tool that brings transparency and clarity to your data pipelines.
Anomaly Detection: Uncover Hidden Insights in Your Data
Data anomalies can have a significant impact on the accuracy and reliability of your data-driven initiatives. Datafold's advanced anomaly detection algorithms automatically identify anomalies and outliers in your data, helping you uncover hidden insights and potential issues. By detecting anomalies early on, you can take immediate action to rectify any data discrepancies and ensure the integrity of your data.
Alerting and Notifications: Stay Informed and Take Action
Keeping data teams informed of any issues or deviations is crucial for maintaining data reliability. Datafold's alerting and notifications feature allows you to set up customizable alerts based on specific conditions or thresholds. Whether it's detecting a sudden drop in data quality or a schema change that affects downstream processes, Datafold ensures that you are promptly notified. This empowers your team to take immediate action, minimizing the impact of any data-related issues.
Collaboration: Work Together, Resolve Issues Faster
Data observability is a team effort, and Datafold understands the importance of collaboration. With its streamlined collaboration features, Datafold enables teams to work together effectively. You can easily share insights, collaborate on data investigations, and resolve issues faster. Datafold provides a centralized platform where team members can communicate, annotate, and track the progress of data-related tasks. This promotes collaboration and ensures that everyone is on the same page, driving efficiency and productivity within your data team.
As you can see, Datafold offers a range of powerful features that enhance data observability. From comprehensive data profiling to advanced anomaly detection, Datafold equips data teams with the tools they need to ensure data reliability and streamline their data workflows. With Datafold, you can confidently embark on your data-driven initiatives, knowing that you have the necessary visibility and insights to make informed decisions.
An Introduction to Marquez
Marquez is another popular data observability tool that helps organizations gain better visibility into their data workflows. Let's explore Marquez with a brief overview.
Marquez: A Brief Overview
Marquez provides a unified and scalable metadata service for organizations to track and manage their data pipelines. It allows data teams to document, discover, and govern their data assets effectively. Marquez promotes data lineage and helps organizations achieve a higher level of data visibility and control.
Key Features of Marquez
Marquez offers a range of robust features that facilitate data observability, including:
- Data Discovery: A centralized catalog that enables users to discover and understand available data assets.
- Data Lineage Tracking: Detailed data lineage tracking that captures the flow of data from source to destination.
- Data Versioning: Version control capabilities that ensure accurate audit trails and reproducibility of data.
- Metadata Management: Efficient management of metadata to provide context and insights into data pipelines.
- Integration: Seamless integration with various data tools and frameworks, simplifying adoption for data teams.
Comparing Datafold and Marquez
Ease of Use: Datafold vs. Marquez
When it comes to ease of use, Datafold excels with its intuitive user interface and seamless integration capabilities. Its user-friendly design allows data teams to quickly adopt the tool and start gaining value from their data observability efforts. Marquez, on the other hand, offers robust functionality but may require more configuration and setup, making it slightly more complex to use initially.
Scalability: Datafold vs. Marquez
Both Datafold and Marquez are designed to scale with an organization's growing data needs. Datafold offers a highly scalable architecture that can handle large volumes of data and adapt to changing workloads seamlessly. Marquez, with its scalable metadata service, ensures a centralized view of data pipelines, making it well-suited for managing data at scale.
Performance: Datafold vs. Marquez
Datafold's performance is characterized by its fast data lineage tracking, efficient data profiling, and real-time anomaly detection capabilities. These features enable data teams to monitor and analyze their data pipelines with minimal latency. Marquez, on the other hand, provides reliable performance in capturing and maintaining data lineage, facilitating data discovery and governance.
Pricing Comparison
Datafold Pricing Structure
Datafold offers flexible pricing plans to cater to organizations of all sizes. Pricing is based on factors such as data volume, the number of users, and required features. For detailed pricing information, it's best to contact Datafold directly.
Marquez Pricing Structure
Marquez follows a similar pricing model, tailored to meet the specific needs of each organization. Pricing is determined based on factors such as data volume, usage, and the level of support required. For precise pricing details, it's recommended to reach out to the Marquez sales team.
Conclusion
In conclusion, both Datafold and Marquez offer powerful capabilities for data observability. Datafold stands out with its user-friendly interface, comprehensive data profiling, and collaborative features. On the other hand, Marquez provides a unified metadata service for managing and tracking data pipelines at scale. When selecting a data observability tool, organizations should consider their specific requirements and evaluate which tool aligns best with their needs and goals.
Remember to explore each tool thoroughly, take advantage of trial or demo versions, and consult with your data team to make an informed decision. With the right data observability tool in place, organizations can ensure data reliability, gain valuable insights, and make data-driven decisions with confidence.
If you're intrigued by the capabilities of data observability tools like Datafold and Marquez, you'll be excited to discover what CastorDoc has to offer. As a platform that integrates advanced governance, cataloging, and lineage features with a user-friendly AI assistant, CastorDoc stands out as a powerful ally for businesses pursuing self-service analytics. It's designed to not only streamline data management for data teams but also to democratize data access for business users through natural language interactions. Experience the future of data governance and unlock the full potential of your data with CastorDoc. Ready to see how CastorDoc compares to other tools in the modern data stack? Check out more tools comparisons here.
You might also like
Contactez-nous pour en savoir plus
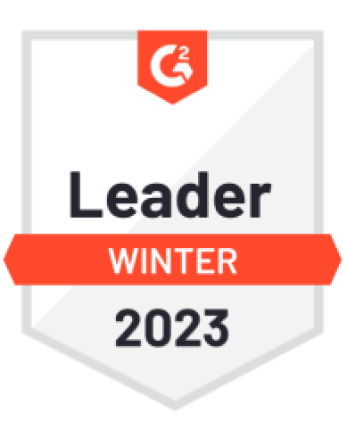
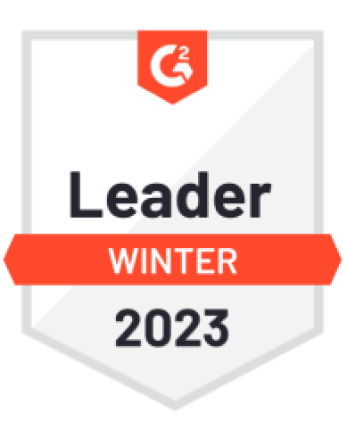

« J'aime l'interface facile à utiliser et la rapidité avec laquelle vous trouvez les actifs pertinents que vous recherchez dans votre base de données. J'apprécie également beaucoup le score attribué à chaque tableau, qui vous permet de hiérarchiser les résultats de vos requêtes en fonction de la fréquence d'utilisation de certaines données. » - Michal P., Head of Data.