Data Lineage & Data Observability: Why Are They Important?
Explore the crucial role of data lineage and data observability in modern data management.

It is crucial to have a clear understanding of where data comes from and how it is transformed throughout its lifecycle. This is where data lineage and data observability come into play. These concepts play a vital role in enabling organizations to gain insights, ensure data quality, and make informed decisions. To fully appreciate their significance, let's delve into the intricacies of data lineage and data observability.
Understanding the Concepts: Data Lineage and Data Observability
Defining Data Lineage
Data lineage refers to the ability to track and visualize the movement, transformation, and processing of data throughout its journey within an organization's systems. It provides a detailed understanding of the data's origins, its transformations, and the final destination. By tracing the lineage, organizations can identify any potential bottlenecks, errors, or discrepancies that may arise during data processing.
Understanding data lineage is crucial for regulatory compliance, data governance, and decision-making processes within an organization. It helps in ensuring data quality, improving data transparency, and enhancing data-driven decision-making. By having a clear understanding of how data flows through various systems and processes, organizations can also enhance their data security measures by identifying potential vulnerabilities in the data flow.
Defining Data Observability
Data observability, on the other hand, is the capability to monitor and infer the integrity, health, and quality of data in real-time. By continuously monitoring the data at each stage of its lifecycle, organizations can detect anomalies, ensure data integrity, and identify potential issues that could impact its quality. In essence, data observability aims to provide stakeholders with a clear picture of the trustworthiness and reliability of data.
Implementing data observability practices involves the use of advanced monitoring tools, anomaly detection algorithms, and automated alerting systems. These tools help organizations in proactively identifying data issues, ensuring data compliance, and maintaining data quality standards. Data observability also plays a crucial role in enabling organizations to make timely decisions based on accurate and reliable data, ultimately leading to improved operational efficiency and better business outcomes.
The Interconnection Between Data Lineage and Data Observability
How Data Lineage Supports Data Observability
Data lineage plays a pivotal role in supporting data observability. By understanding the lineage of data, organizations gain insights into the potential points of failure or degradation, allowing them to proactively monitor and address issues. The ability to trace data lineage provides stakeholders with the necessary context to identify the root cause of data anomalies and take appropriate corrective actions. It ensures that data observability is not limited to real-time monitoring but is extended to the entire data journey.
Furthermore, data lineage serves as a historical record of data transformations and movements, capturing the evolution of data from its source to its destination. This historical perspective offered by data lineage is essential for compliance, auditing, and regulatory purposes. It enables organizations to demonstrate data provenance and ensure data governance by tracking how data has been used, modified, or shared over time.
The Role of Data Observability in Enhancing Data Lineage
Data observability complements data lineage by providing real-time insights into the quality metrics and performance indicators of data. It enables organizations to detect and rectify data issues before they impact downstream processes. By incorporating data observability into the data lineage framework, organizations can enhance the accuracy and reliability of the lineage information. This, in turn, promotes trust in the data lineage and ensures the integrity of analytical insights and decision-making processes.
In addition, data observability tools offer features such as data monitoring, anomaly detection, and data quality assessments, which contribute valuable metadata to enrich the data lineage information. This enriched lineage not only helps in understanding the flow of data but also provides insights into the health and reliability of the data at each stage of its journey. The synergy between data observability and data lineage creates a robust data management framework that empowers organizations to make informed decisions based on a comprehensive understanding of their data ecosystem.
The Importance of Data Lineage and Data Observability
Ensuring Data Quality and Accuracy
Data lineage and data observability go hand in hand to ensure data quality and accuracy. By understanding the lineage and continuously monitoring the data, organizations can identify any inconsistencies or inaccuracies that may occur during its transformation. This enables them to rectify the issues promptly, ensuring that downstream processes are built on the foundation of high-quality and accurate data.
For example, let's consider a retail company that relies heavily on customer data to drive its marketing campaigns. By implementing data observability measures, such as real-time monitoring and anomaly detection, the company can identify any discrepancies in the data, such as duplicate or missing entries. This level of data observability allows them to take immediate action, ensuring that their marketing efforts are based on reliable and accurate customer information.
Facilitating Regulatory Compliance
In today's highly regulated environment, compliance with data privacy and security regulations is paramount. Data lineage and data observability provide organizations with the ability to demonstrate the traceability and integrity of data, which is essential for compliance purposes. By maintaining a comprehensive record of data lineage and enacting data observability measures, organizations can ensure adherence to regulatory requirements and minimize the risk of non-compliance.
Take, for instance, a healthcare organization that handles sensitive patient data. By implementing data lineage and observability practices, they can track the journey of patient data from collection to storage and ensure that it is handled in compliance with regulations like HIPAA. This not only helps them avoid hefty fines and legal repercussions but also builds trust with patients who rely on the organization to protect their private information.
Enhancing Decision-Making Processes
Data-driven decision-making relies on the availability of reliable and trusted data. By leveraging data lineage and data observability, organizations can enhance the accuracy and trustworthiness of the data used in decision-making processes. Through a clear understanding of the data's lineage and continuous monitoring, stakeholders can make informed decisions based on data they can rely on, leading to improved business outcomes.
Consider a financial institution that needs to make critical investment decisions. By implementing data lineage and observability, they can ensure that the data used for analysis is accurate, complete, and up-to-date. This level of confidence in the data empowers decision-makers to make strategic investment choices that are based on a solid foundation of trustworthy information, ultimately driving better financial performance for the institution and its clients.
Challenges in Implementing Data Lineage and Data Observability
Technical Challenges
Implementing data lineage and data observability can pose various technical challenges. Extracting lineage information can be complex, especially in environments with multiple data sources and complex transformations. Ensuring the scalability and performance of the systems responsible for capturing and maintaining data lineage can also be a challenge. Adequate integration with existing data infrastructure and technologies is necessary to overcome these hurdles.
Another technical challenge in implementing data lineage and data observability is the need for robust metadata management. Metadata, which provides context and information about the data, must be accurately captured and maintained to ensure the effectiveness of data lineage tracking. This requires careful planning and implementation of metadata repositories and tagging mechanisms to link data elements across the organization.
Organizational Challenges
Organizational challenges can hinder the successful implementation of data lineage and data observability. Resistance to change, lack of awareness, and siloed approaches can impede the establishment of a data-centric culture. Additionally, aligning stakeholders and establishing clear roles and responsibilities for data governance and monitoring is crucial for successful implementation.
Furthermore, fostering collaboration and communication across different departments and teams is essential to address organizational challenges. Creating cross-functional teams dedicated to data lineage and observability initiatives can help break down silos and promote a culture of data transparency and accountability. Leadership support and buy-in at all levels of the organization are also vital to drive the necessary cultural shifts towards data-driven decision-making.
Overcoming the Challenges
Adopting the Right Tools and Technologies
Overcoming the technical challenges requires leveraging the right tools and technologies. Implementing robust data lineage and observability solutions can automate the extraction of lineage information and provide real-time monitoring capabilities. This allows organizations to have a comprehensive understanding of how data flows through their systems, enabling them to identify bottlenecks, pinpoint issues, and optimize their data pipelines.
Adopting technologies such as metadata management platforms, data quality tools, and monitoring systems can streamline the implementation process and ensure scalability. Metadata management platforms provide a centralized repository for storing and managing metadata, making it easier to track and trace data lineage. Data quality tools help organizations maintain the integrity and accuracy of their data, ensuring that the lineage information is reliable and trustworthy. Monitoring systems, on the other hand, provide real-time visibility into the health and performance of data pipelines, allowing organizations to proactively detect and address any issues that may arise.
Building a Data-Centric Culture
Instituting a data-centric culture is instrumental in overcoming organizational challenges. Establishing clear data governance policies, communication channels, and training programs can foster awareness and collaboration. By creating a shared understanding of the importance of data lineage and data observability, organizations can break down silos and encourage cross-functional collaboration.
Training programs play a crucial role in equipping employees with the necessary skills and knowledge to effectively leverage data lineage and observability tools. By providing comprehensive training, organizations can empower their workforce to make data-driven decisions and take ownership of data quality. This not only enhances the overall data management capabilities of the organization but also promotes a culture of continuous learning and improvement.
In conclusion, data lineage and data observability are crucial components of an effective data management strategy. Understanding the concepts and their interconnection, along with their importance in ensuring data quality, facilitating regulatory compliance, and enhancing decision-making processes, is vital for organizations striving to derive value from their data. Though challenges exist, leveraging the right tools and technologies while fostering a data-centric culture can enable organizations to fully embrace data lineage and data observability, paving the way for data-driven success.
You might also like
Get in Touch to Learn More
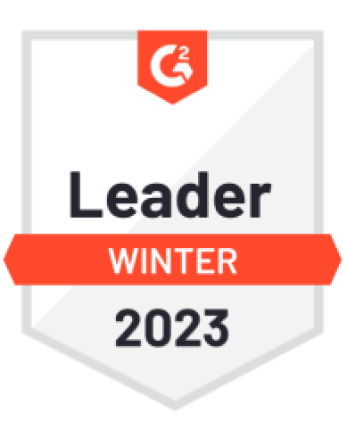
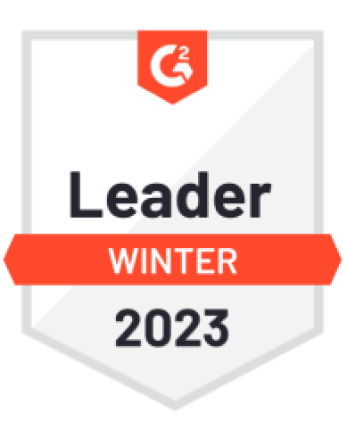

“[I like] The easy to use interface and the speed of finding the relevant assets that you're looking for in your database. I also really enjoy the score given to each table, [which] lets you prioritize the results of your queries by how often certain data is used.” - Michal P., Head of Data