Data Quality Problems? 5 Ways to Fix Them in 2024!
Discover 5 innovative strategies to tackle data quality issues in 2024! Uncover solutions to fix data quality problems and ensure accurate, reliable data for your business..

The importance of data quality cannot be overstated. Businesses rely on accurate, reliable data to make informed decisions, drive growth, and gain a competitive edge. However, data quality problems continue to plague organizations, leading to lost opportunities and costly mistakes. In this article, we will delve into the significance of data quality and explore five effective ways to fix data quality problems in the year 2024.
Understanding the Importance of Data Quality
As data has become the lifeblood of modern business, ensuring its quality is paramount. When data is accurate, complete, and up-to-date, organizations can derive insights, make reliable predictions, and develop effective strategies. Conversely, poor data quality can have severe repercussions, hampering decision-making processes and impeding business growth.
Ensuring data quality involves a combination of processes, including data cleansing, validation, and normalization. Data cleansing involves detecting and correcting errors or inconsistencies in the data, ensuring its accuracy and reliability. Validation ensures that data meets specific criteria or standards, preventing the entry of incorrect or incomplete information. Normalization involves organizing data in a consistent format, making it easier to analyze and interpret.
The Role of Data in Modern Business
Data is no longer just a byproduct but a strategic asset that drives business success. With the advent of big data and analytics, organizations can harness data to gain valuable insights into customer behavior, market trends, and operational efficiency. High-quality data enables businesses to optimize processes, enhance customer experiences, and stay ahead of the competition.
Furthermore, data quality plays a crucial role in regulatory compliance and risk management. In industries such as finance, healthcare, and telecommunications, accurate and reliable data is essential for meeting regulatory requirements and ensuring data security. Poor data quality can lead to compliance violations, legal issues, and reputational damage, highlighting the importance of maintaining data integrity.
The Consequences of Poor Data Quality
On the other hand, poor data quality can lead to detrimental effects on various business aspects. Inaccurate or incomplete data can result in flawed analyses, false assumptions, and poor decision-making. This can lead to missed opportunities, dissatisfied customers, and financial losses. It is essential to address data quality problems to mitigate these risks.
Moreover, poor data quality can also impact data-driven initiatives such as machine learning and artificial intelligence. These technologies rely on high-quality data to train algorithms and make accurate predictions. Low-quality data can introduce biases, errors, and inaccuracies into the models, compromising their effectiveness and reliability. Addressing data quality issues is crucial for ensuring the success and scalability of AI and machine learning applications.
Identifying Common Data Quality Problems
To fix data quality problems effectively, it is crucial to identify and understand the common challenges organizations face. Here are three common data quality problems:
Incomplete or Missing Data
One of the most prevalent data quality issues is incomplete or missing data. When certain data points are missing or incomplete, it can impair the accuracy and reliability of analyses and decisions based on that data. Poor data entry processes, system limitations, and human error are often the culprits behind this problem.
Addressing incomplete or missing data requires implementing robust data validation processes and establishing clear data capture guidelines. Organizations can also leverage data profiling tools to identify gaps in their datasets and develop strategies to fill those gaps effectively. By ensuring comprehensive data collection practices, businesses can enhance the quality and usability of their data assets.
Inaccurate Data
Data inaccuracies can arise from various sources, such as data entry errors, outdated information, or technical glitches. Inaccurate data undermines the credibility of analyses, reports, and business insights derived from it. Detecting and rectifying inaccurate data is crucial for maintaining data integrity and ensuring reliable decision-making.
To combat inaccurate data, organizations can implement data cleansing procedures that involve regular data quality checks, deduplication processes, and anomaly detection algorithms. Employing data validation rules and establishing data quality metrics can help in identifying and correcting inaccuracies proactively. Additionally, fostering a data-driven culture within the organization can promote accountability and encourage stakeholders to prioritize data accuracy in their day-to-day operations.
Outdated Data
In the rapidly evolving digital landscape, data becomes outdated at an alarming rate. Outdated data can mislead decision-makers and hinder their ability to address current market dynamics. Regularly updating data and implementing effective data governance practices are essential for maintaining data relevance and ensuring business agility.
Organizations can mitigate the risks associated with outdated data by establishing data stewardship roles responsible for monitoring data currency and relevance. Implementing data lifecycle management strategies can help in systematically archiving obsolete data and updating existing datasets with the most recent information. Leveraging data synchronization tools and integrating real-time data feeds can also aid in keeping data up-to-date and aligned with the dynamic business environment.
Implementing Data Quality Management
Now that we have identified common data quality problems, let us explore how organizations can implement effective data quality management strategies. It is crucial for businesses to prioritize data quality management to ensure that their decision-making processes are based on accurate and reliable information.
Implementing data quality management involves a multi-faceted approach that requires collaboration across different departments within an organization. By establishing a robust data quality management framework, companies can enhance their operational efficiency, reduce risks associated with poor data quality, and ultimately improve customer satisfaction.
The Basics of Data Quality Management
Data quality management involves a systematic approach to maintain and improve data quality across an organization. It encompasses processes, tools, and methodologies to ensure data accuracy, completeness, consistency, and timeliness. Data quality management should be a collaborative effort involving business stakeholders, data professionals, and IT teams. By fostering a data-driven culture within the organization, companies can empower employees to make informed decisions based on high-quality data.
Furthermore, data quality management is not a one-time project but an ongoing initiative that requires continuous monitoring and refinement. Organizations must invest in training programs to educate employees on the importance of data quality and provide them with the necessary skills to contribute to data governance and data quality improvement efforts.
Key Components of Data Quality Management
Successful data quality management strategies should include the following key components:
- Data Governance: Establishing clear policies, procedures, and responsibilities for managing data quality.
- Data Profiling: Analyzing data to identify data quality issues and anomalies.
- Data Cleansing: Removing or correcting inaccurate, incomplete, or inconsistent data.
- Data Integration: Ensuring seamless integration of data from various sources while maintaining data quality.
- Data Quality Monitoring: Regularly monitoring data quality and implementing corrective measures when necessary.
Each of these components plays a critical role in ensuring that data is accurate, reliable, and actionable. Data governance sets the foundation for data quality management by defining roles and responsibilities, while data profiling and cleansing help organizations identify and address data quality issues proactively. Data integration and quality monitoring, on the other hand, ensure that data remains consistent and up-to-date across all systems and processes.
Five Effective Solutions for Data Quality Problems
To fix data quality problems, organizations should adopt a multi-faceted approach. Here are five effective solutions:
Data Cleansing Techniques
Data cleansing involves identifying and correcting data inaccuracies, inconsistencies, and redundancies. Techniques such as deduplication, standardization, and validation can help improve data quality. Automated data cleansing tools can streamline the process and ensure accuracy and efficiency.
For instance, deduplication is a powerful technique that eliminates duplicate records from a dataset. By identifying and removing redundant entries, organizations can ensure that their data remains clean and free from unnecessary clutter. Standardization, on the other hand, involves transforming data into a consistent format, making it easier to analyze and compare. Validation techniques, such as data type validation and range validation, help ensure that data entered into a system meets specific criteria, reducing the risk of errors and inconsistencies.
Data Governance Strategies
Implementing robust data governance strategies is vital for maintaining data quality. This involves establishing clear data quality standards, defining roles and responsibilities, and ensuring compliance with data management policies. Data governance frameworks and practices can instill data quality as a cultural norm within an organization.
Effective data governance goes beyond just setting rules and regulations. It involves creating a data-driven culture where every employee understands the importance of data quality and takes responsibility for maintaining it. By fostering a culture of data governance, organizations can ensure that data quality becomes ingrained in their day-to-day operations, leading to better decision-making and improved business outcomes.
Data Integration Solutions
Data integration plays a crucial role in maintaining data quality, especially when organizations deal with data from multiple sources. Implementing data integration solutions that automate and streamline data pipelines can ensure consistent, accurate, and up-to-date data across different systems.
With the increasing volume and variety of data, organizations often face challenges in consolidating and integrating data from various sources. Data integration solutions, such as Extract, Transform, Load (ETL) processes and data integration platforms, provide a systematic approach to combining data from disparate sources. These solutions not only enable organizations to merge data seamlessly but also ensure that data quality is maintained throughout the integration process.
Data Quality Tools and Software
Investing in data quality tools and software can significantly enhance data quality management efforts. These tools can automate data profiling, cleansing, and monitoring processes, allowing organizations to identify and address data quality issues efficiently. From data quality dashboards to data cleansing algorithms, a wide range of tools is available to suit different organizational needs.
For example, data profiling tools enable organizations to gain insights into the quality of their data by analyzing its structure, completeness, and consistency. These tools can automatically detect anomalies and outliers, helping organizations identify potential data quality issues. Data cleansing tools, on the other hand, provide a systematic way to clean and correct data inaccuracies, ensuring that data remains accurate and reliable. By leveraging data quality tools and software, organizations can streamline their data quality management efforts and focus on deriving meaningful insights from their data.
Regular Data Audits
Conducting regular data audits is essential to maintain data quality. Data audits involve evaluating data against predefined quality standards, identifying discrepancies, and implementing corrective actions. By conducting periodic data audits, organizations can proactively address data quality problems and prevent them from escalating.
Data audits can take various forms, such as manual inspections, automated checks, and statistical analysis. These audits help organizations identify data quality issues, such as missing values, inconsistent formats, and outliers. By addressing these issues promptly, organizations can ensure that their data remains accurate, reliable, and fit for purpose. Regular data audits also provide an opportunity for organizations to assess the effectiveness of their data quality management strategies and make necessary improvements.
In conclusion, data quality problems can have far-reaching consequences for businesses. However, by understanding the importance of data quality, identifying common data quality problems, implementing data quality management strategies, and adopting effective solutions, organizations can fix data quality problems and harness the full potential of their data assets. By investing in data cleansing techniques, data governance strategies, data integration solutions, data quality tools and software, and regular data audits, organizations can ensure that their data remains accurate, reliable, and valuable. With data quality as a top priority, businesses can make informed decisions, fuel innovation, and succeed in a data-driven world.
You might also like
Get in Touch to Learn More
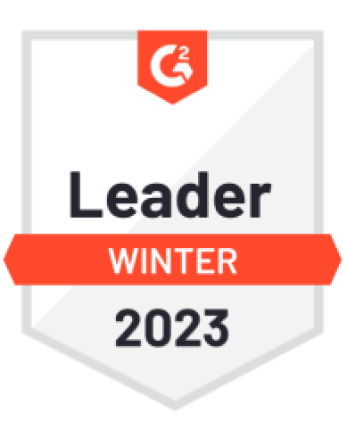
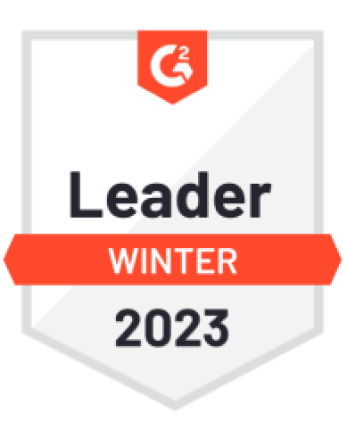

“[I like] The easy to use interface and the speed of finding the relevant assets that you're looking for in your database. I also really enjoy the score given to each table, [which] lets you prioritize the results of your queries by how often certain data is used.” - Michal P., Head of Data