Unlocking the Power of Semantic Data: The Key to Smarter Data Integration and Analysis
Learn how semantic data can revolutionize your data integration and analysis processes.
Semantic data has become increasingly vital in today's digital age. With the sheer volume of data being generated every day, it has become crucial for businesses to harness the power of semantic data to enable smarter data integration and analysis. By leveraging semantic data, businesses can enhance their data integration processes, streamline analysis, and unlock valuable insights. In this article, we will explore the importance of semantic data, its role in data integration, its impact on data analysis, and its future potential. We will also provide practical steps for implementing semantic data into your business and measuring its success.
Understanding Semantic Data
Defining Semantic Data
Semantic data refers to data that carries additional contextual meaning beyond its literal representation. It goes beyond traditional data formats and incorporates rich metadata, enabling machines to understand the relationships between different data elements. By providing context, semantic data enhances the accuracy and relevance of data analysis, leading to more actionable insights.
One key aspect of semantic data is its ability to facilitate interoperability between different systems and applications. By adhering to standardized semantic web technologies such as RDF (Resource Description Framework) and OWL (Web Ontology Language), organizations can ensure that their data is not only understandable within their own ecosystem but also easily shareable and interpretable across diverse platforms.
The Importance of Semantic Data in Today's Digital Age
In the vast landscape of data-driven decision making, semantic data plays a crucial role in extracting relevant information from vast datasets. With the exponential growth of data sources and formats, businesses face significant challenges in integrating and analyzing information effectively. Semantic data helps overcome these challenges by providing structure and meaning to raw data, making it easier to extract valuable insights and drive informed decision-making.
Furthermore, semantic data fosters the development of advanced technologies such as artificial intelligence and machine learning. These technologies heavily rely on well-structured data with clear semantics to train algorithms and make accurate predictions. By leveraging semantic data, organizations can enhance the performance of their AI systems and deliver more personalized and efficient services to their customers.
The Role of Semantic Data in Data Integration
How Semantic Data Enhances Data Integration
Data integration involves consolidating data from various sources, formats, and systems into a unified view. However, traditional data integration approaches often struggle with the heterogeneity and complexity of data. Semantic data addresses these challenges by enabling the integration of structured and unstructured data, incorporating metadata, and establishing common vocabularies. This improves data discoverability, compatibility, and interoperability, leading to more accurate and comprehensive data integration.
Moreover, semantic data plays a crucial role in enhancing data governance within organizations. By providing a semantic layer that defines the meaning and relationships between data elements, it facilitates better data management practices. This structured approach to data integration ensures data security, compliance with regulations, and effective data stewardship.
Overcoming Data Integration Challenges with Semantic Data
Data integration poses several challenges, including data inconsistency, lack of interoperability, and data quality issues. Semantic data helps overcome these challenges by providing a standardized framework for data integration. It ensures data consistency by mapping different data elements to a common ontology and resolving semantic conflicts. By enhancing data compatibility and ensuring interoperability, semantic data enables seamless integration across diverse data sources and systems.
Furthermore, the use of semantic data in data integration processes leads to improved data lineage and traceability. Organizations can track the origins and transformations of data across various stages of integration, ensuring data provenance and auditability. This transparency not only enhances data quality but also builds trust among stakeholders regarding the accuracy and reliability of integrated data.
Semantic Data and Data Analysis
Leveraging Semantic Data for Effective Data Analysis
Data analysis involves extracting insights and patterns from data to inform decision-making. Semantic data significantly enhances data analysis by providing valuable context. This context enables data scientists and analysts to understand relationships between entities, uncover hidden connections, and gain a deeper understanding of the data. By leveraging semantic data, businesses can improve the accuracy, relevance, and speed of their data analysis, leading to more precise and informed decisions.
Furthermore, semantic data can help in data integration by linking related information from different sources. This integration allows for a more comprehensive analysis, as it combines data from various domains to provide a holistic view. By incorporating semantic data into the integration process, organizations can break down data silos and create a unified data environment that supports more robust analysis and decision-making.
The Impact of Semantic Data on Predictive Analysis
Predictive analysis relies on historical data to forecast future outcomes or trends. Semantic data enriches predictive analysis by improving the quality and relevance of input data. By incorporating contextual information, semantic data enables more accurate predictions by identifying intricate patterns and relationships that might be missed using traditional data analysis approaches. This empowers businesses to make more reliable predictions and optimize their decision-making processes.
Moreover, semantic data can enhance predictive modeling by providing a deeper understanding of the underlying semantics of the data. This understanding allows data scientists to create more sophisticated models that capture complex relationships and dependencies, leading to more accurate predictions. By leveraging semantic data in predictive analysis, organizations can gain a competitive edge by anticipating market trends and customer behavior with greater precision.
The Future of Semantic Data
Emerging Trends in Semantic Data
Semantic data is evolving rapidly, with several exciting trends shaping its future. One such trend is the proliferation of linked data, where disparate datasets are interlinked to create a web of interconnected information. This web-like structure enables seamless data discovery, sharing, and integration, paving the way for smarter and more comprehensive data analysis. Additionally, advancements in natural language processing (NLP) and machine learning are making it easier to extract semantic meaning from unstructured data, further expanding the possibilities of semantic data analysis.
Another significant development in the realm of semantic data is the rise of knowledge graphs. Knowledge graphs are powerful tools that represent information in a structured way, connecting entities and their relationships. By leveraging knowledge graphs, organizations can gain deeper insights, improve data quality, and facilitate more informed decision-making processes. The interconnected nature of knowledge graphs enables a holistic view of data, enabling users to explore complex relationships and uncover valuable patterns.
The Potential of Semantic Data in AI and Machine Learning
Artificial intelligence (AI) and machine learning (ML) are increasingly reliant on semantic data to achieve higher levels of accuracy and sophistication. Semantic data enhances AI and ML algorithms by providing the necessary context to understand and interpret data more effectively. By incorporating semantic data, AI systems can improve inferences, automatic classification, and language understanding capabilities. As AI and ML continue to evolve, semantic data will play a pivotal role in unlocking their full potential and enabling more intelligent and personalized experiences.
Furthermore, the combination of semantic data with AI and ML technologies opens up new possibilities in various industries, such as healthcare, finance, and e-commerce. In healthcare, semantic data can be utilized to enhance patient care through personalized treatment plans and predictive analytics. In finance, semantic data can improve risk assessment models and fraud detection mechanisms. In e-commerce, semantic data can enable more accurate product recommendations and personalized shopping experiences. The synergy between semantic data and AI/ML is revolutionizing how organizations leverage data to drive innovation and achieve competitive advantages.
Implementing Semantic Data in Your Business
Steps to Incorporate Semantic Data
Implementing semantic data in your business requires careful planning and execution. Here are some key steps to consider:
- Define your semantic data strategy: Identify the specific goals and objectives you want to achieve with semantic data and align them with your overall business strategy.
- Design your ontology: Develop a semantic model that represents your business domain and describes the relationships between different data elements.
- Adopt semantic data standards: Use established semantic standards, such as RDF (Resource Description Framework) and OWL (Web Ontology Language), to ensure compatibility and interoperability.
- Integrate semantic data into your existing systems: Incorporate semantic data integration techniques, such as data mapping and transformation, to integrate semantic data into your existing data infrastructure.
- Train your team: Provide training and support to your team members to ensure they have the necessary skills and knowledge to work with semantic data effectively.
Measuring the Success of Semantic Data Implementation
Measuring the success of semantic data implementation involves evaluating its impact on key performance indicators (KPIs) and business outcomes. Some metrics to consider include improved data integration efficiency, enhanced data analysis capabilities, increased accuracy in predictions, and a higher return on investment (ROI) from data-driven initiatives. Regular monitoring and evaluation will help identify areas of improvement and ensure ongoing optimization of your semantic data implementation.
In conclusion, the power of semantic data cannot be understated in today's data-driven landscape. By understanding the nuances of semantic data, businesses can leverage its potential to realize smarter data integration and analysis. Whether it is improving data integration processes, enhancing data analysis capabilities, or predicting future trends, semantic data opens a world of possibilities. By adopting a strategic approach and following best practices, businesses can unlock the full potential of semantic data and gain a competitive edge in the ever-evolving digital age.
Ready to harness the transformative power of semantic data in your organization? CastorDoc is here to guide you through the journey. As the most reliable AI Agent for Analytics, CastorDoc empowers your business teams with trustworthy, instantaneous data answers, enabling self-service analytics and maximizing the ROI of your data stack. Experience the autonomy and trust to make informed decisions with CastorDoc. Try CastorDoc today and unlock the full potential of your data.
You might also like
Get in Touch to Learn More
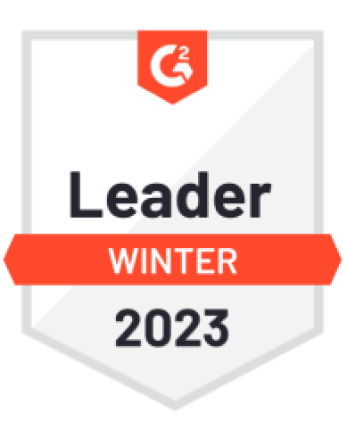
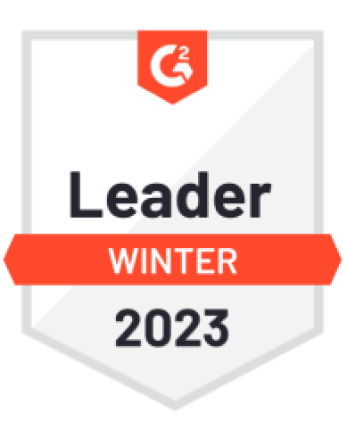

“[I like] The easy to use interface and the speed of finding the relevant assets that you're looking for in your database. I also really enjoy the score given to each table, [which] lets you prioritize the results of your queries by how often certain data is used.” - Michal P., Head of Data