Building Powerful Semantic Models: 7 Secrets Most Analytics Teams Miss
Unlock the hidden potential of your analytics team with these 7 often-overlooked secrets to building powerful semantic models.

In today's data-driven world, building powerful semantic models is essential for effective analytics. Semantic models provide a structured framework for understanding data relationships, leading to better decision-making. However, many analytics teams overlook crucial aspects of developing these models, leading to missed opportunities and inaccurate insights. This article explores the significance of semantic models, common mistakes teams make, and seven secrets to mastering their development.
Understanding the Importance of Semantic Models in Analytics
Semantic models are critical in transforming raw data into actionable insights. By defining the relationships between different data sets, these models enable analytics teams to interpret complex information in a meaningful way. This facilitates a better understanding of customer behavior, market trends, and operational efficiency. In today's data-driven landscape, organizations that harness the power of semantic models can gain a competitive edge, as they can quickly adapt to changes and make informed decisions based on real-time data analysis.
The role of semantics in data interpretation cannot be overstated. A well-constructed semantic model enhances data usability, ensuring that all stakeholders can engage with analytics without needing deep technical expertise. Consequently, decision-makers at all levels can leverage insights derived from data, leading to a more agile and responsive organization. Furthermore, as businesses increasingly rely on data to guide their strategies, the ability to communicate insights clearly becomes paramount. Semantic models serve as a bridge, translating complex data into understandable formats that resonate with diverse audiences, from marketing teams to executive leadership.
The Role of Semantic Models in Data Interpretation
Semantic models connect disparate data points into a coherent narrative. They provide a common language for data analysis, which is essential in organizations with vast amounts of diverse data. By using standardized terminologies and structures, analytics teams can ensure that all interpretations and conclusions from data are consistent and aligned with organizational goals. This consistency not only improves the quality of insights but also fosters trust among stakeholders, as they can rely on a shared understanding of the data being analyzed.
This structured approach is particularly beneficial in scenarios where data is continuously changing. Semantic models enable teams to adjust their analyses dynamically as new data comes in, ensuring that insights remain relevant and accurate. Additionally, by implementing semantic models, organizations can better manage the complexities of big data, allowing for more sophisticated analyses that take into account the nuances of relationships between data points. This adaptability is crucial in fast-paced environments where timely decision-making can significantly impact business outcomes.
Why Your Analytics Team Needs to Prioritize Semantic Models
Analytics teams often focus on technological advancements and algorithmic improvements, neglecting the foundational importance of semantic models. Prioritizing these models can lead to significant improvements in data quality, interpretation, and stakeholder engagement. The clarity that semantic models provide fosters collaboration between technical and non-technical team members, bridging the gap in understanding across different departments. This collaborative environment encourages innovation, as diverse perspectives can come together to analyze data from various angles, leading to richer insights.
Moreover, focusing on semantic models enhances the longevity of analytics initiatives. As the organization grows and diversifies, having a robust semantic foundation allows for seamless integration of new data sources and analytics tools. In contrast, ignoring semantic structures can lead to redundant work and confusion, undermining the overall effectiveness of data initiatives. By establishing a strong semantic framework, organizations can future-proof their analytics strategies, ensuring that they remain relevant and impactful as the data landscape evolves. This proactive approach not only saves time and resources but also empowers teams to explore new opportunities for growth and efficiency in their operations.
The Common Mistakes Analytics Teams Make with Semantic Models
Despite their importance, many analytics teams make critical mistakes when developing semantic models. Recognizing these pitfalls is the first step toward creating more effective models that drive better insights.
Overlooking the Complexity of Semantic Models
One of the most significant errors teams make is underestimating the complexity involved in developing semantic models. These models require careful consideration of various elements, including data relationships, context, and the different use cases for the data. A lack of thoroughness in these areas can result in models that are overly simplistic or misaligned with the actual data.
This oversight can lead to scenarios where data misinterpretations become commonplace, ultimately eroding trust in analytics outputs. Teams must acknowledge the intricate nature of these models and invest the necessary time and resources into their development. Furthermore, the integration of multiple data sources can add layers of complexity that are often overlooked. For instance, when combining structured data from databases with unstructured data from social media or customer feedback, it is essential to establish clear relationships and hierarchies to ensure that the semantic model accurately reflects the nuances of the data. This holistic approach not only enhances the model's reliability but also enriches the insights derived from it.
Misunderstanding the Purpose of Semantic Models
Another common mistake is misunderstanding the purpose of semantic models. Some analytics teams view these models solely as passive repositories of information rather than as dynamic tools for enhancing data analytics. This narrow perspective limits the potential of semantic models to drive insights and improve decision-making.
To avoid this trap, it is crucial for teams to consistently engage with and revise their semantic models. This ensures that the models evolve alongside changes in the data landscape and organizational priorities, thereby maximizing their utility. Additionally, fostering a culture of collaboration between data engineers, analysts, and business stakeholders can lead to a more comprehensive understanding of the model’s purpose. By involving diverse perspectives in the development process, teams can create semantic models that not only serve current analytical needs but also anticipate future requirements, making them more resilient and adaptable in a rapidly changing business environment. This proactive approach can significantly enhance the strategic value of data analytics within the organization.
The 7 Secrets to Building Powerful Semantic Models
To build effective semantic models, analytics teams should adopt the following seven secrets. Each secret brings essential considerations that can significantly enhance the effectiveness of model development.
Secret 1: Embracing the Complexity of Semantic Models
Embracing complexity begins with recognizing that semantic models are not one-size-fits-all solutions. Teams should be prepared to explore the intricate details of their data sets, including nuances in terminology, relationships, and context. This understanding enables the creation of models that genuinely reflect the underlying data dynamics. Additionally, it is crucial to engage with domain experts who can provide insights into the specific language and context of the data, ensuring that the model captures the subtleties that may otherwise be overlooked. By doing so, teams can create a more nuanced representation of the data that enhances both interpretability and usability.
Secret 2: Prioritizing Accuracy in Semantic Models
Accuracy is paramount. Every element of a semantic model must be carefully validated to ensure it aligns with true data relationships. Regular audits and quality checks will help maintain high levels of accuracy, yielding trustworthy insights. Furthermore, employing automated validation tools can streamline this process, allowing teams to quickly identify discrepancies and rectify them before they propagate through the model. By integrating continuous monitoring mechanisms, organizations can maintain a high standard of accuracy over time, ensuring that the insights derived from the models remain relevant and actionable.
Secret 3: Ensuring Consistency in Semantic Models
Consistency across models is vital for effective communication within an analytics team. To achieve this, teams should implement standard conventions for terminology and data representation, ensuring that all members speak the same 'data language.' This consistency not only aids in collaboration but also simplifies the onboarding process for new team members, who can more easily grasp the established conventions. Additionally, creating a centralized repository of definitions and standards can serve as a reference point, promoting uniformity and reducing the likelihood of misinterpretation across various projects.
Secret 4: Focusing on Scalability of Semantic Models
As organizations grow, their data requirements evolve. Consequently, semantic models must be constructed with scalability in mind. This involves designing models that can accommodate increasing volumes of data and additional dimensions without a complete redesign. It is also beneficial to adopt modular design principles that allow for the easy addition or modification of components as new data sources emerge. By anticipating future needs and building flexibility into the architecture, teams can ensure that their models remain relevant and effective as the organization’s data landscape changes.
Secret 5: Incorporating Flexibility in Semantic Models
Flexibility is essential in a rapidly changing data landscape. Developing models that can quickly adapt to new data sources, organizational needs, and analytical methodologies will position teams to remain effective in fluid environments. This adaptability can be further enhanced by leveraging technologies such as machine learning and artificial intelligence, which can help automate the integration of new data types and formats. By fostering a culture of innovation and experimentation, teams can stay ahead of the curve and continuously refine their models to meet evolving analytical demands.
Secret 6: Maintaining Transparency in Semantic Models
Transparency fosters trust in the analytics process. Stakeholders should be able to understand how models are constructed and the logic behind them. Documenting model development processes and decisions ensures clarity and allows for easier troubleshooting and revisions when necessary. Moreover, creating visual representations of the model architecture can aid in demystifying complex structures, making it easier for non-technical stakeholders to grasp the underlying concepts. Engaging in open discussions about model assumptions and limitations can also promote a culture of accountability, where all team members feel empowered to contribute to the model's integrity.
Secret 7: Encouraging Collaboration in Semantic Model Development
Finally, encouraging collaboration among team members promotes diverse perspectives that enhance model quality. Cross-functional teamwork can reveal insights and considerations that might be overlooked in siloed environments, ultimately leading to more robust semantic models. Establishing regular brainstorming sessions and workshops can facilitate knowledge sharing and foster a sense of community within the team. Additionally, utilizing collaborative tools and platforms can streamline communication, making it easier for team members to share ideas and feedback in real-time. By cultivating an environment that values collaboration, teams can harness the collective expertise of their members to drive innovation and improve the overall quality of their semantic models.
Implementing These Secrets into Your Analytics Team
Transforming these secrets into actionable strategies involves a series of deliberate steps and cultural shifts within the analytics team.
Steps to Incorporate These Secrets into Your Team's Workflow
Begin by engaging the team in workshops to raise awareness about the significance of semantic models. Provide training on best practices and common pitfalls associated with model development. From there, establish guidelines that incorporate the seven secrets into regular workflows, ensuring they become an integral part of the team’s operations.
Overcoming Challenges in Semantic Model Development
Implementing these strategies may present challenges, such as resistance to change or existing workflows that are deeply ingrained. Address these challenges head-on through open discussions that highlight the benefits of adopting semantic models and integrate their principles gradually. Encourage feedback and be prepared to iterate on processes based on team experiences.
Ultimately, developing powerful semantic models is not just about the models themselves; it's about cultivating a culture of data literacy, collaboration, and continuous improvement within analytics teams.
Ready to elevate your analytics team's capabilities and harness the full potential of your data? CastorDoc is here to transform the way you approach semantic models and data governance. With advanced cataloging, lineage capabilities, and a user-friendly AI assistant, CastorDoc enables self-service analytics that empower both data professionals and business users alike. Take control of your data governance lifecycle, ensure compliance, and enhance data quality with ease. For business users, CastorDoc's natural language interactions make data accessible and understandable, allowing you to confidently drive strategic decisions. Don't miss out on the opportunity to revolutionize your organization's data management. Try CastorDoc today and unlock the secrets to building powerful semantic models that most analytics teams miss.
You might also like
Get in Touch to Learn More
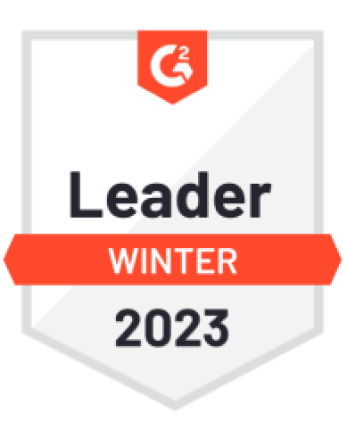
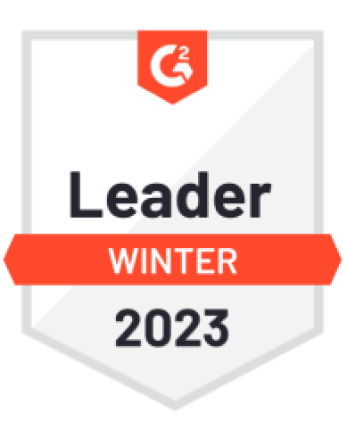

“[I like] The easy to use interface and the speed of finding the relevant assets that you're looking for in your database. I also really enjoy the score given to each table, [which] lets you prioritize the results of your queries by how often certain data is used.” - Michal P., Head of Data