Data Governance Maturity Unlocked: The 5 Essential Steps
Unlock the secrets to achieving data governance maturity with these 5 essential steps! Discover how to navigate the complexities of data governance and elevate your organization's data management practices to the next level..
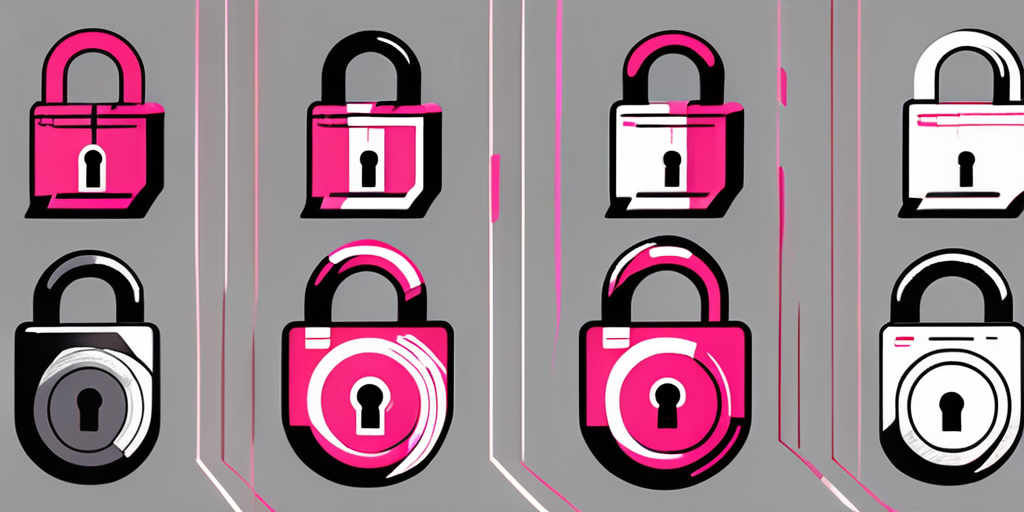
In today's data-driven world, organizations are faced with the challenge of effectively managing and governing their data assets. Data governance plays a crucial role in ensuring that data is accurate, reliable, and aligned with business objectives. To achieve a mature state of data governance, organizations must follow a structured approach. In this article, we will explore the five essential steps to unlocking data governance maturity.
Understanding Data Governance Maturity
Data governance maturity refers to the level of effectiveness and sophistication in an organization's data governance practices. It encompasses the processes, policies, controls, and structures that govern the management of data across the enterprise. Understanding data governance maturity is crucial as it allows organizations to assess their current state and identify areas for improvement.
Organizations that prioritize data governance maturity often experience improved operational efficiency and increased stakeholder trust. By establishing clear guidelines and procedures for data management, organizations can streamline processes, reduce data errors, and enhance data security. Furthermore, a mature data governance framework fosters a culture of data accountability and transparency, where employees understand the importance of data quality and compliance.
The Importance of Data Governance Maturity
Data governance maturity is vital for organizations seeking to establish a reliable and accurate data management framework. When data is properly governed, organizations can make informed decisions, achieve regulatory compliance, mitigate risks, and improve overall data quality. Data governance maturity provides a solid foundation for data-driven initiatives and enhances the organization's ability to derive value from its data assets.
Moreover, data governance maturity plays a crucial role in fostering innovation and driving competitive advantage. Organizations with mature data governance practices are better equipped to leverage data analytics, artificial intelligence, and other emerging technologies to gain valuable insights and stay ahead of market trends. By nurturing a data-driven culture through mature data governance, organizations can unlock new opportunities for growth and differentiation in a rapidly evolving business landscape.
Key Components of Data Governance Maturity
Data governance maturity comprises several key components. These include data strategy and vision, data governance team, data governance framework, data governance policies and procedures, and ongoing monitoring and continuous improvement. These components work in synergy to establish a robust data governance ecosystem, ensuring data is effectively managed and governed.
Effective data strategy and vision set the direction for data governance initiatives, aligning them with organizational goals and objectives. A dedicated data governance team ensures that responsibilities are clearly defined, and accountability is established across the organization. The data governance framework serves as the structural backbone, outlining the processes and mechanisms for data management and decision-making. Policies and procedures provide the necessary guidelines for data handling, privacy, and security, while ongoing monitoring and continuous improvement mechanisms enable organizations to adapt to changing data landscapes and evolving regulatory requirements.
Step 1: Establishing a Data Governance Vision
Before embarking on the journey to data governance maturity, organizations must define a clear vision for their data governance initiatives. This involves understanding the organization's data governance goals and aligning them with its broader business objectives.
Establishing a robust data governance vision requires a deep dive into the organization's current data landscape. This includes conducting a comprehensive assessment of existing data governance practices, identifying gaps and areas for improvement, and determining the level of data maturity within the organization. By gaining a thorough understanding of the current state of data governance, organizations can develop a vision that is realistic, achievable, and tailored to their specific needs.
Defining Your Data Governance Goals
A crucial step in establishing a data governance vision is defining specific and measurable data governance goals. These goals should address the organization's pain points and align with its strategic priorities. Examples of data governance goals may include improving data quality, ensuring data privacy and security, or enhancing data sharing and collaboration.
Furthermore, when defining data governance goals, organizations should consider industry regulations and compliance requirements that impact their data management practices. By aligning data governance goals with regulatory mandates, organizations can ensure that their data governance initiatives not only drive business value but also mitigate risks associated with non-compliance.
Aligning Your Vision with Business Objectives
To be effective, a data governance vision must be aligned with the organization's overall business objectives. This alignment ensures that data governance initiatives are supported by the organization's leadership and are integrated into its broader business strategy. By aligning the vision with business objectives, organizations can secure the necessary resources and executive sponsorship to drive data governance maturity.
In addition to aligning with business objectives, a well-defined data governance vision should also consider the evolving technological landscape. With the rapid advancements in data management technologies such as artificial intelligence and machine learning, organizations need to ensure that their data governance vision is flexible and adaptable to incorporate emerging technologies. By future-proofing their data governance vision, organizations can stay ahead of the curve and leverage cutting-edge tools to maximize the value of their data assets.
Step 2: Building a Data Governance Team
An essential element of data governance maturity is the establishment of a dedicated data governance team. This team is responsible for driving and overseeing the data governance initiatives within the organization.
Creating a data governance team involves more than just assigning titles; it requires assembling a group of individuals with diverse skill sets and expertise. These team members should possess a deep understanding of the organization's data landscape, business processes, and regulatory requirements. By bringing together individuals with different backgrounds and perspectives, the data governance team can effectively address complex data challenges and drive meaningful change.
Identifying Key Stakeholders
Building a data governance team requires identifying key stakeholders who will champion and support data governance efforts. These stakeholders may include executives, department heads, data stewards, and subject matter experts. Engaging stakeholders from various departments ensures cross-functional representation and facilitates the adoption and implementation of data governance practices.
Key stakeholders play a crucial role in advocating for data governance initiatives across the organization. They provide strategic direction, allocate resources, and communicate the importance of data governance to all employees. By securing buy-in from key stakeholders, the data governance team can gain the necessary support and resources to drive meaningful change and establish a culture of data excellence.
Roles and Responsibilities within the Team
Once the key stakeholders are identified, it is essential to define the roles and responsibilities within the data governance team. This includes appointing a data governance leader or manager who will be responsible for overseeing the overall data governance program. Additionally, roles such as data stewards, data owners, and data custodians should be clearly defined to ensure accountability and effective governance of data assets.
Each role within the data governance team carries specific responsibilities aimed at ensuring data quality, integrity, and security. Data stewards are tasked with defining data standards and policies, while data owners take ownership of specific data domains and ensure compliance with regulations. Data custodians, on the other hand, are responsible for implementing data governance policies and procedures at a technical level, safeguarding data from unauthorized access or misuse.
Step 3: Developing a Data Governance Framework
A robust data governance framework is critical to achieving data governance maturity. The framework provides a structured approach to managing data, defining roles and responsibilities, and establishing processes and controls for data governance.
Essential Elements of a Data Governance Framework
A comprehensive data governance framework should include elements such as data governance policies, data standards, data quality controls, metadata management, data privacy and security measures, and data lifecycle management. These elements collectively form the blueprint for effective data governance and ensure that data is properly managed throughout its lifecycle.
Implementing the Framework
Implementing a data governance framework requires a phased approach, starting with defining the governance objectives and scope, establishing data governance processes and controls, and implementing technology solutions to support data governance activities. Organizations should regularly assess and refine the data governance framework to adapt to evolving business needs and industry best practices.
Step 4: Executing Data Governance Policies and Procedures
Execution is a crucial step in data governance maturity. Organizations must create and enforce effective data governance policies and procedures to ensure compliance and alignment with data governance objectives.
Creating Effective Policies
Data governance policies outline the rules and guidelines for managing data and provide the necessary framework for enforcing data governance practices. Effective policies should be comprehensive, clearly communicated, and aligned with industry standards and regulatory requirements. Organizations should establish processes for policy creation, review, approval, and dissemination to ensure that data governance policies are up-to-date and applicable.
Ensuring Compliance and Enforcement
To achieve data governance maturity, organizations must ensure compliance and enforcement of data governance policies and procedures. This involves monitoring data governance activities, conducting regular audits, and implementing appropriate checks and balances to identify and rectify non-compliant behavior. By enforcing compliance, organizations can maintain data integrity and trust, supporting data-driven decision-making processes.
In conclusion, achieving data governance maturity is a journey that requires a systematic approach and commitment from the entire organization. By following the five essential steps outlined in this article, organizations can unlock data governance maturity and harness the full potential of their data assets. Data governance maturity not only enhances data quality and reliability but also enables organizations to stay competitive in the rapidly evolving data landscape.You might also like
Get in Touch to Learn More
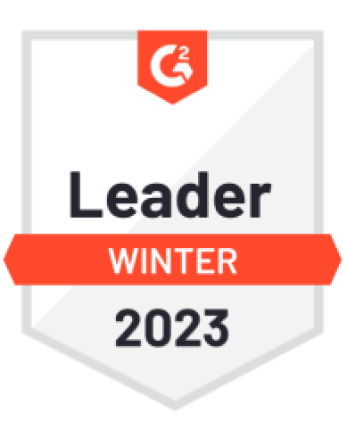
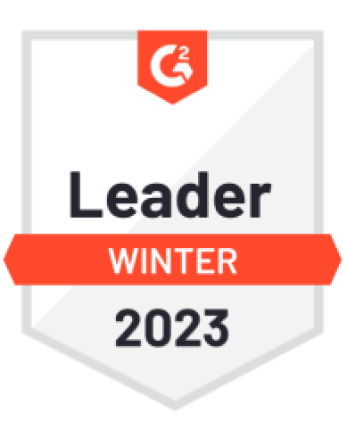

“[I like] The easy to use interface and the speed of finding the relevant assets that you're looking for in your database. I also really enjoy the score given to each table, [which] lets you prioritize the results of your queries by how often certain data is used.” - Michal P., Head of Data