Data Lakehouses: The Best of Data Lakes and Data Warehouses
Discover the ultimate fusion of data lakes and data warehouses with our in-depth article on data lakehouses.
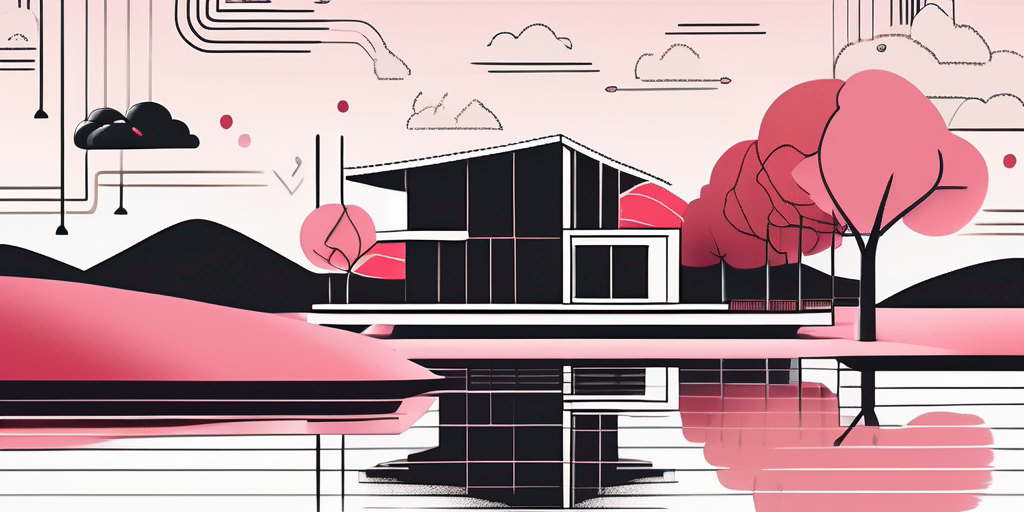
In this era of big data and analytics, organizations are constantly seeking innovative solutions to store, manage, and analyze their ever-growing volumes of data. Two popular options that have emerged in recent years are data lakes and data warehouses. While both have their strengths and weaknesses, a new approach known as data lakehouses offers a unique amalgamation, combining the best of both worlds. In this article, we will delve into the concept of data lakehouses, explore their architecture, and discuss the advantages they bring to businesses.
Understanding the Concept of Data Lakehouses
Firstly, let's define what exactly we mean by data lakehouses. A data lakehouse is a data management approach that combines the raw storage capabilities of data lakes with the structured querying capabilities of data warehouses. It aims to eliminate the trade-offs associated with traditional data storage and processing methods, offering a more holistic solution for data management. This integration of features allows organizations to seamlessly store, process, and analyze vast amounts of data without compromising on performance or flexibility.
Defining Data Lakehouses
Data lakehouses act as a single source of truth for an organization's data, consolidating both structured and unstructured data into a unified repository. Unlike the traditional approach of data warehousing, this unified data lakehouse does not require predefined schema or upfront data transformation. Instead, it allows for schema-on-read, enabling faster and more agile data exploration and analysis.
The Evolution of Data Lakehouses
The concept of data lakehouses has evolved as a response to the limitations of existing systems. Data lakes offered a powerful solution for storing large volumes of raw data, but lacked the structure necessary for efficient querying and analysis. On the other hand, data warehouses provided structure but were often inflexible and unable to handle the volume and variety of modern data. The emergence of data lakehouses sought to bridge this gap, providing organizations with a unified and versatile data management solution.
With the rise of big data and the increasing complexity of data sources, organizations faced challenges in managing and extracting value from their data. Traditional data storage and processing methods were no longer sufficient to handle the sheer volume and variety of data being generated. This led to the development of data lakehouses, which combined the best aspects of data lakes and data warehouses.
By leveraging the raw storage capabilities of data lakes, organizations could store vast amounts of data in its original format, without the need for upfront transformation or predefined schema. This flexibility allowed for the inclusion of both structured and unstructured data, enabling a more comprehensive view of the organization's data assets. Additionally, the structured querying capabilities of data warehouses provided the necessary tools for efficient analysis and reporting.
One of the key advantages of data lakehouses is their ability to support schema-on-read. This means that data can be analyzed and interpreted at the time of query, rather than requiring a predefined schema. This eliminates the need for time-consuming data transformation processes, allowing for faster and more agile data exploration. It also enables organizations to easily incorporate new data sources without disrupting existing workflows.
Furthermore, data lakehouses offer improved performance compared to traditional data storage and processing methods. By leveraging distributed computing frameworks, such as Apache Spark, organizations can parallelize data processing tasks and achieve faster query execution times. This scalability ensures that data lakehouses can handle the growing demands of big data analytics, providing timely insights to drive informed decision-making.
In conclusion, data lakehouses represent a significant advancement in data management, offering organizations a unified and versatile solution for storing, processing, and analyzing data. By combining the strengths of data lakes and data warehouses, data lakehouses provide the flexibility and performance required to effectively manage modern data challenges. As organizations continue to generate and accumulate vast amounts of data, the adoption of data lakehouses is expected to increase, empowering businesses to unlock the full potential of their data assets.
The Intersection of Data Lakes and Data Warehouses
Before diving into the advantages of data lakehouses, it is important to understand the key features of both data lakes and data warehouses.
Key Features of Data Lakes
Data lakes are designed to store vast amounts of raw and unfiltered data in its native format. With data lakes, organizations can ingest structured, semi-structured, and unstructured data without the need for predefined schema or data transformation. This flexibility allows for easy scalability and adaptability, accommodating ever-changing business needs.
One of the key benefits of data lakes is their ability to store data in its native format, preserving the original structure and content. This raw data can later be transformed and processed as needed, enabling organizations to derive valuable insights from diverse data sources.
Key Features of Data Warehouses
Data warehouses, on the other hand, are structured repositories that store processed and aggregated data optimized for reporting and analysis. They are designed to provide fast and reliable querying capabilities for business intelligence purposes. Data warehouses enforce schema and data quality constraints, ensuring consistency and accuracy in reporting.
Another important aspect of data warehouses is their support for complex queries and analytics. By structuring data in a way that is optimized for querying, data warehouses enable efficient analysis of large datasets, empowering organizations to make data-driven decisions with speed and precision.
The Superiority of Data Lakehouses
Now, let's delve deeper into the world of data lakehouses and uncover the myriad advantages they bring to the table. The seamless integration of data lakes and data warehouses into a unified infrastructure not only revolutionizes data management but also propels organizations towards unparalleled success.
Picture this: a data ecosystem where raw and refined data coexist harmoniously in a centralized location, thanks to the innovative concept of data lakehouses. By harnessing the combined power of data lakes and data warehouses, organizations bid farewell to data duplication woes and embrace a single source of truth. This centralized approach not only eliminates data silos but also streamlines data management processes, making it a breeze to govern and maintain data at scale.
Enhanced Data Management
One of the standout features of data lakehouses is their unparalleled ability to cater to both raw and refined data requirements within a unified framework. This unique approach not only simplifies data storage but also ensures data integrity and consistency across the board. By consolidating data lakes and data warehouses, organizations can bid adieu to the complexities of managing disparate data sources and embrace a more cohesive and efficient data management strategy.
Improved Data Accessibility
Unlocking a treasure trove of possibilities, data lakehouses pave the way for enhanced data accessibility like never before. By seamlessly blending exploratory analytics and structured reporting capabilities, these cutting-edge platforms cater to a diverse range of user needs. Data scientists and analysts can revel in the freedom to explore raw data using flexible query mechanisms offered by data lakes, while business users can tap into the structured querying prowess of data warehouses to derive actionable insights and reports with ease. This versatility empowers stakeholders across the organization to access and analyze data in a manner that aligns perfectly with their unique requirements and preferences.
The Architecture of Data Lakehouses
Now let's take a closer look at the architecture of data lakehouses and understand the building blocks that make them work.
The Building Blocks of Data Lakehouses
At the core of a data lakehouse architecture lies a unified storage layer that can handle both structured and unstructured data. This storage layer can leverage cloud-based storage solutions such as Amazon S3, Azure Blob Storage, or Google Cloud Storage. On top of the storage layer, organizations deploy query engines or data processing frameworks that enable efficient querying and analysis of the data.
The Role of Cloud Computing in Data Lakehouses
Cloud computing plays a pivotal role in the architecture of data lakehouses. By leveraging the scalability and flexibility offered by the cloud, organizations can seamlessly scale their data storage and processing capabilities. Additionally, the cloud provides built-in security and reliability features that ensure the integrity and availability of the data.
Implementing Data Lakehouses in Business
While the advantages of data lakehouses are clear, their successful implementation requires careful planning and preparation.
Preparing Your Business for Data Lakehouses
Before embarking on the journey towards implementing a data lakehouse, organizations must assess their data infrastructure and ensure compatibility with the new architecture. This may involve restructuring existing data pipelines, training personnel, and establishing data governance practices. Adequate planning is key to ensuring a smooth transition and maximizing the benefits of a data lakehouse.
Potential Challenges and Solutions
Like any technological transformation, implementing data lakehouses can present challenges. One common challenge is data quality and governance, as data lakes allow for the ingestion of raw and unstructured data. Organizations must carefully define and enforce data quality standards to prevent the introduction of inaccurate or incomplete data. Additionally, organizations may face integration challenges when migrating from existing data storage systems. Thorough testing and correct implementation strategies can mitigate these challenges and lead to successful adoption of data lakehouses.
In conclusion, data lakehouses provide a comprehensive and versatile solution for managing modern data requirements. By combining the strengths of data lakes and data warehouses, organizations can achieve enhanced data management, improved data accessibility, and streamlined analytics. As businesses continue to face the challenge of ever-increasing data volumes, data lakehouses present an enticing option for optimizing data storage, processing, and analysis.
You might also like
Get in Touch to Learn More
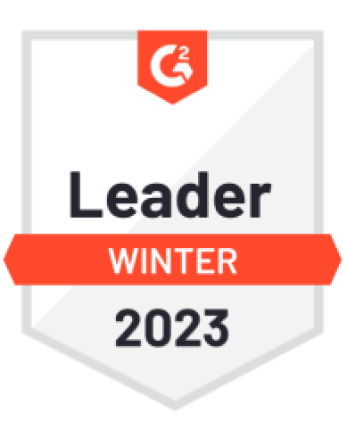
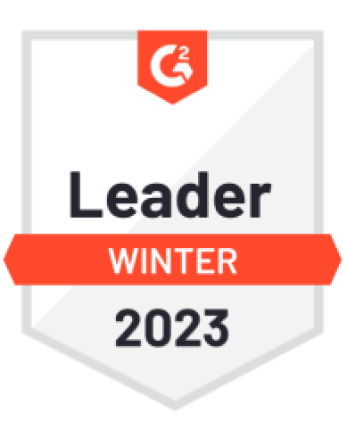

“[I like] The easy to use interface and the speed of finding the relevant assets that you're looking for in your database. I also really enjoy the score given to each table, [which] lets you prioritize the results of your queries by how often certain data is used.” - Michal P., Head of Data