Data Lineage vs Data Traceability: 7 Key Distinctions
Explore the intricate differences between data lineage and data traceability in this article.
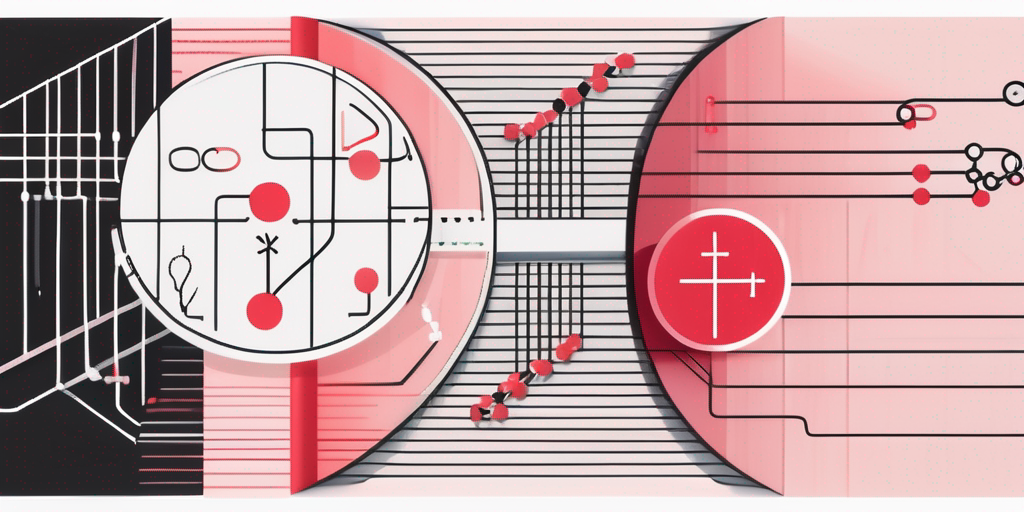
In the world of data management and analysis, two key concepts that often come up are data lineage and data traceability. While these terms may seem similar at first glance, they actually have distinct differences that are important to understand. In this article, we will unravel the basics of data lineage and data traceability, explore their significance in business, compare the two concepts, and highlight the seven key distinctions between them.
Understanding the Basics: Data Lineage
Data lineage refers to the ability to trace and understand the origin, transformation, and movement of data throughout its lifecycle. It provides a comprehensive record of how data is created, stored, and processed, allowing organizations to have a clear understanding of the data's provenance and lineage. This information is critical for various purposes, such as auditing, compliance, troubleshooting, and ensuring data quality.
When delving deeper into the concept of data lineage, it becomes apparent that it serves as a foundational pillar for data governance within organizations. By establishing a robust data lineage framework, companies can not only ensure regulatory compliance but also enhance their decision-making processes. The intricate web of connections between data sources, transformations, and destinations offers a holistic view of data flow, empowering stakeholders to make informed choices based on a thorough understanding of data origins.
Definition and Importance of Data Lineage
At its core, data lineage is a visual representation or documentation that depicts the flow of data from its source to its destination. It helps answer questions like "Where did this data come from?" and "What transformations did it undergo?". By having a clear understanding of data lineage, organizations can ensure data quality and make well-informed decisions based on accurate and reliable data.
Furthermore, data lineage serves as a crucial tool for enhancing data transparency and accountability within an organization. By establishing a transparent data lineage process, companies can build trust among stakeholders and foster a data-driven culture. This transparency also aids in identifying potential data discrepancies or anomalies, enabling swift corrective actions to maintain data integrity.
The Role of Data Lineage in Data Management
Data lineage plays a crucial role in effective data management. It allows organizations to track changes and updates made to the data, understand dependencies between different data elements, and identify potential risks or bottlenecks in data processes. With data lineage, organizations can ensure compliance with data governance standards, improve data quality, and streamline data integration and migration projects.
Moreover, data lineage acts as a guiding light for data architects and analysts, offering valuable insights into the impact of data changes on downstream systems and processes. This proactive approach to data management not only minimizes the risk of data errors but also enhances the overall efficiency of data-related initiatives. By leveraging data lineage as a strategic asset, organizations can pave the way for data-driven innovation and sustainable growth.
Unraveling Data Traceability
Data traceability is another important concept in the world of data management. It refers to the ability to track and trace data elements and their attributes throughout their lifecycle, providing transparency and visibility into the data's history. Data traceability is particularly relevant in industries with stringent regulatory requirements, such as pharmaceuticals and food production.
Understanding data traceability involves delving into the intricate web of connections that data elements create as they move through various systems and processes. Each data point carries with it a story of its origin, transformations, and interactions, forming a complex narrative that can reveal insights into how information flows within an organization.
What is Data Traceability?
Data traceability involves capturing detailed information about the origin, movement, and processing of data elements. It aims to ensure that data can be traced back to its source and that any changes or transformations made to the data are recorded and auditable. Data traceability helps organizations maintain the integrity of their data, comply with industry regulations, and provide evidence of compliance, if required.
Furthermore, data traceability extends beyond mere record-keeping; it serves as a foundational pillar for data governance frameworks. By establishing clear traceability mechanisms, organizations can enhance data quality, facilitate decision-making processes, and mitigate risks associated with data breaches or inaccuracies.
Significance of Data Traceability in Business
In business, data traceability plays a crucial role in maintaining trust and credibility. It allows organizations to demonstrate the integrity of their products and services by providing evidence of quality control processes, compliance with industry standards, and adherence to regulatory requirements. Data traceability is particularly important in sectors where consumer safety and product authenticity are paramount.
Moreover, data traceability fosters a culture of accountability within an organization. By establishing clear data lineage and audit trails, businesses can empower their teams to take ownership of data assets, promote transparency in decision-making processes, and enhance overall data stewardship practices.
Comparing Data Lineage and Data Traceability
While data lineage and data traceability share similarities in terms of capturing data history and ensuring transparency, they have distinct differences that set them apart.
Data lineage is a concept that focuses on tracking the complete journey of data from its origin to its current state. It provides a detailed record of how data has been transformed and moved throughout various systems and processes. This information is crucial for understanding the context and quality of data, making it a valuable asset for data governance and decision-making processes.
On the other hand, data traceability emphasizes the ability to trace the origins and whereabouts of specific data points. It focuses on the ability to track individual data elements across different systems and processes, enabling organizations to quickly identify the source of data discrepancies or errors. This granular level of tracking is essential for ensuring data accuracy and compliance with regulatory requirements.
Similarities Between Data Lineage and Data Traceability
- Both data lineage and data traceability aim to capture and document the history of data elements.
- They both provide transparency and visibility into data processes and transformations.
- Both concepts contribute to maintaining data integrity and compliance with industry standards.
7 Key Distinctions Between Data Lineage and Data Traceability
While data lineage and data traceability share similarities, there are seven key distinctions that set them apart:
Distinction 1: Purpose and Function
Data lineage focuses on understanding the origin and movement of data, while data traceability aims to track data elements and attributes to ensure compliance and quality control.
Distinction 2: Scope and Depth
Data lineage provides a comprehensive view of data flow across systems, applications, and processes, while data traceability focuses on specific data elements and their attributes.
Distinction 3: Tools and Techniques
Data lineage is often visualized through lineage mapping tools, data catalogs, or metadata management systems. Data traceability typically relies on unique identifiers, barcodes, or RFID tags.
Distinction 4: Impact on Data Quality
Data lineage helps identify data quality issues by tracing the data's journey and highlighting potential areas of concern. Data traceability ensures data integrity by capturing the history of data changes and ensuring compliance with quality control standards.
Distinction 5: Role in Compliance and Regulation
Data lineage provides valuable evidence for regulatory compliance by documenting data processes and transformations. Data traceability is essential for industries with strict traceability requirements, such as pharmaceuticals or food production.
Distinction 6: Influence on Decision Making
Data lineage helps decision makers understand the reliability and accuracy of data, enabling them to make informed decisions. Data traceability ensures the availability of accurate data for decision making, especially in industries where consumer safety is critical.
Distinction 7: Effect on Data Governance
Data lineage contributes to data governance by providing insights into data movement and ensuring compliance with data management policies. Data traceability helps organizations achieve regulatory compliance and maintain trust in their products and services.
While these distinctions are crucial to understanding the differences between data lineage and data traceability, it is important to delve deeper into each distinction to fully grasp their significance.
Let's explore the first distinction in more detail. Data lineage, as mentioned earlier, focuses on understanding the origin and movement of data. This means that it not only tracks where the data comes from but also how it flows through various systems, applications, and processes. By having a clear understanding of the data's journey, organizations can identify any bottlenecks or areas where data quality may be compromised.
On the other hand, data traceability aims to track specific data elements and their attributes. This is particularly important in industries where compliance and quality control are critical, such as pharmaceuticals or food production. By assigning unique identifiers, barcodes, or RFID tags to data elements, organizations can easily trace their movement and ensure that they meet regulatory requirements.
As you can see, the scope and depth of data lineage and data traceability differ significantly. While data lineage provides a holistic view of data flow, data traceability focuses on specific data elements. This distinction is essential for organizations to determine which approach best suits their needs and objectives.
Furthermore, the tools and techniques used for data lineage and data traceability also differ. Data lineage is often visualized through lineage mapping tools, data catalogs, or metadata management systems. These tools help organizations visualize the complex web of data flow and understand how different data elements are interconnected. On the other hand, data traceability relies on unique identifiers, barcodes, or RFID tags to track data elements. These technologies enable organizations to easily identify and trace specific data elements throughout their lifecycle.
As we can see, each distinction between data lineage and data traceability brings unique benefits and considerations. By understanding these distinctions in greater detail, organizations can make informed decisions about which approach to adopt and how to leverage them effectively.
You might also like
Get in Touch to Learn More
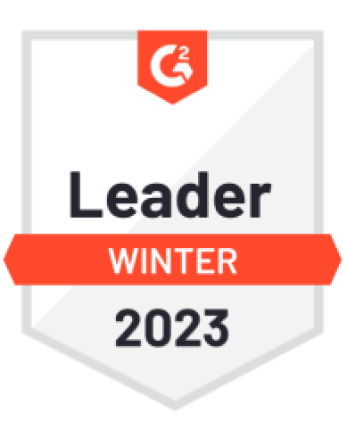
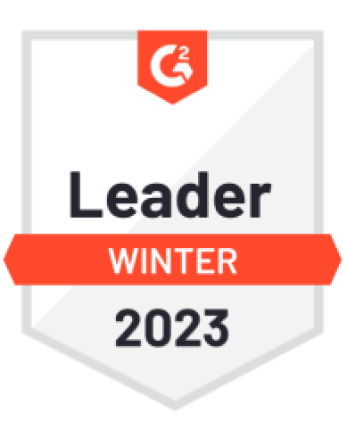

“[I like] The easy to use interface and the speed of finding the relevant assets that you're looking for in your database. I also really enjoy the score given to each table, [which] lets you prioritize the results of your queries by how often certain data is used.” - Michal P., Head of Data