Data Quality Explained: Causes, Detection, and Fixe
Learn about the importance of data quality and how to identify and address issues in your datasets.
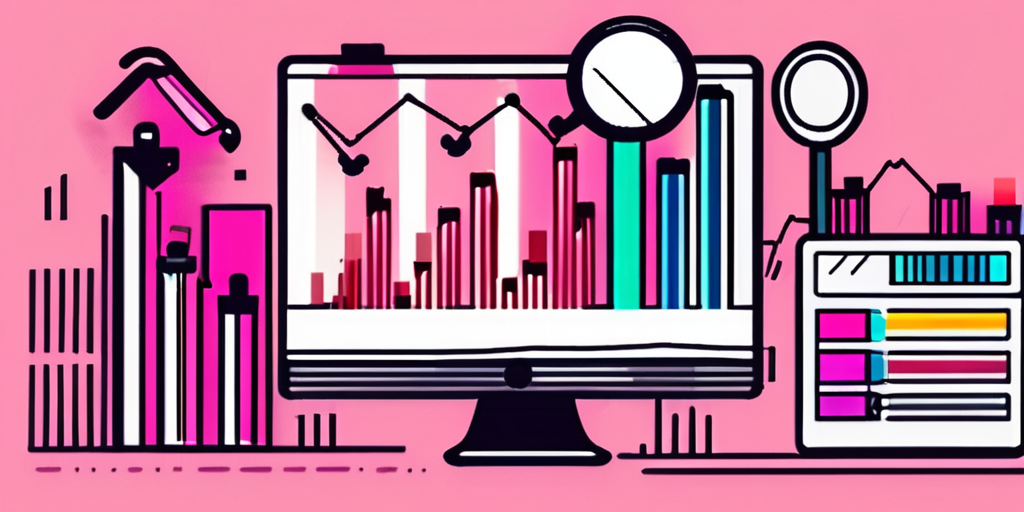
In today's data-driven world, the importance of data quality cannot be overstated. Data serves as the foundation for making critical business decisions, driving innovation, and gaining a competitive advantage. However, poor data quality can have significant repercussions on an organization. In this article, we will discuss the causes of data quality issues, the process of detecting them, and strategies for fixing them.
Understanding the Importance of Data Quality
The Role of Data in Modern Business
Data plays a central role in modern business operations. It is the lifeblood that organizations rely on to gain insights, improve operational efficiency, enhance customer experiences, and drive growth. High-quality data helps businesses make informed and accurate decisions, while poor-quality data can lead to costly mistakes and missed opportunities.
How Poor Data Quality Impacts Decision-Making
Poor data quality can have direct consequences on decision-making processes. Decision-makers heavily rely on accurate and reliable data to forecast trends, identify market opportunities, and mitigate risks. When the data is incorrect, incomplete, or inconsistent, decision-makers may make misguided choices that can have negative implications for the business.
The Impact of Data Quality on Customer Relationships
Aside from decision-making, data quality also significantly affects customer relationships. Inaccurate or outdated customer data can result in failed communication attempts, duplicate marketing efforts, and ultimately, dissatisfied customers. On the other hand, maintaining clean and reliable customer data enables businesses to personalize interactions, anticipate needs, and build long-lasting relationships based on trust and understanding.
Strategies for Ensuring Data Quality
Given the critical role of data quality in business success, organizations must implement strategies to ensure the accuracy and reliability of their data. This includes regular data cleansing processes, validation checks, data governance policies, and investing in technologies that automate data quality assurance. By prioritizing data quality, businesses can unlock the full potential of their data assets and drive sustainable growth and innovation.
Identifying the Causes of Poor Data Quality
Human Error and Data Entry
One of the primary causes of poor data quality is human error during the data entry process. Even with well-defined procedures, mistakes can occur, leading to typos, incorrect values, or missing information. These errors can propagate throughout the data lifecycle, affecting its overall quality.
System and Software Failures
Another common cause of poor data quality is system and software failures. Technical glitches, software bugs, or hardware malfunctions can corrupt or alter data, resulting in inconsistencies or inaccuracies. It is crucial for organizations to have robust systems in place to detect and resolve such failures promptly.
Inadequate Data Governance Policies
The absence or inadequacy of data governance policies can also contribute to poor data quality. Data governance defines the rules, processes, and responsibilities for managing and maintaining data integrity. When organizations lack clear data governance frameworks, data inconsistencies, redundancies, and inaccuracies are more likely to occur.
External Data Sources and Integration Challenges
Integrating data from external sources can pose challenges that impact data quality. External data sources may have different formats, structures, or quality standards, leading to issues such as data duplication or mismatched records. Organizations must establish robust data integration processes to ensure seamless data flow and consistency across various sources.
Lack of Data Quality Monitoring and Reporting
Without proper monitoring and reporting mechanisms in place, organizations may overlook data quality issues until they escalate into significant problems. Regular data quality assessments, automated alerts for anomalies, and detailed reporting on data accuracy are essential for proactively identifying and addressing data quality issues in a timely manner.
The Process of Data Quality Detection
Data Profiling Techniques
Data profiling techniques involve analyzing data sets to uncover anomalies, inconsistencies, and data quality issues. By examining data characteristics such as completeness, accuracy, and consistency, organizations can identify areas that require improvement and take corrective actions accordingly.
One common data profiling technique is outlier detection, which focuses on identifying data points that significantly deviate from the norm. Outliers can indicate errors in data entry or processing, making them crucial to address for maintaining data quality. Additionally, data profiling often involves data standardization, where different formats and representations of data are harmonized to ensure consistency and accuracy across the dataset.
Data Quality Assessment Tools
Data quality assessment tools provide automated solutions to detect, measure, and monitor data quality. These tools evaluate data against predefined quality metrics, enabling organizations to proactively identify and address data quality issues. By leveraging these tools, businesses can streamline the detection process and focus on improving data integrity.
Some advanced data quality assessment tools utilize machine learning algorithms to continuously learn from data patterns and automatically adapt quality checks. This dynamic approach allows organizations to stay ahead of evolving data quality challenges and ensure ongoing data reliability. Moreover, these tools often offer customizable dashboards and reports to provide stakeholders with real-time insights into data quality metrics and trends.
The Role of Data Stewards in Detection
Data stewards play a crucial role in data quality detection. They are responsible for ensuring the proper management, integrity, and usability of data. Data stewards collaborate with stakeholders across the organization to define data quality standards, implement data quality processes, and monitor the ongoing quality of data.
Furthermore, data stewards act as advocates for data quality within the organization, promoting best practices and fostering a culture of data governance. They work closely with data owners and data users to establish data quality KPIs and ensure that data quality initiatives align with business objectives. By engaging stakeholders at all levels, data stewards facilitate a comprehensive approach to data quality detection and maintenance.
Strategies for Fixing Data Quality Issues
Implementing Data Cleansing
Data cleansing involves the process of identifying and correcting or removing inaccurate, incomplete, or irrelevant data. Through various techniques such as deduplication, validation, and enrichment, organizations can enhance data quality and reliability. Data cleansing should be performed regularly to maintain high-quality data.
One important aspect of data cleansing is deduplication. This process involves identifying and removing duplicate records from a dataset. By eliminating duplicates, organizations can avoid confusion and ensure that their data is accurate and up-to-date. Validation is another crucial technique in data cleansing. It involves checking the accuracy and consistency of data against predefined rules or standards. This helps identify any inconsistencies or errors that may exist in the dataset. Lastly, data enrichment is a technique that involves enhancing existing data with additional information from external sources. This can include adding demographic data, geolocation data, or any other relevant information that can improve the quality and value of the dataset.
Establishing Data Governance Framework
To address data quality issues holistically, organizations should establish a robust data governance framework. This framework encompasses policies, procedures, and structures designed to ensure data integrity, accessibility, and usability. By implementing a comprehensive data governance program, organizations can promote data quality as a strategic priority.
One key component of a data governance framework is data stewardship. Data stewards are responsible for ensuring the quality and integrity of data within an organization. They play a crucial role in defining data standards, monitoring data quality, and resolving any data-related issues. Another important aspect of data governance is data documentation. This involves documenting the metadata, data lineage, and data definitions to provide a clear understanding of the data and its quality. Additionally, data governance frameworks often include data quality metrics and KPIs (Key Performance Indicators) to measure and track the effectiveness of data quality initiatives.
The Role of Data Quality Management Software
Data quality management software provides organizations with the tools and capabilities to monitor, measure, and improve data quality continuously. These software solutions offer features such as data profiling, data cleansing, data matching, and data integration. By leveraging data quality management software, organizations can automate data quality processes and maintain high standards of data integrity.
Data profiling is a key feature of data quality management software. It involves analyzing the structure, content, and quality of data to identify any anomalies or issues. This helps organizations gain insights into the overall quality of their data and prioritize areas for improvement. Data matching is another important capability of data quality management software. It involves comparing and matching data across different datasets to identify duplicates or inconsistencies. This helps organizations ensure data consistency and accuracy across various systems and databases. Additionally, data integration features in data quality management software enable organizations to consolidate and integrate data from multiple sources, ensuring that data is accurate and consistent across the entire organization.
In conclusion, data quality is crucial for organizations to make accurate decisions, gain valuable insights, and stay ahead in today's competitive landscape. The causes of poor data quality can vary, but identifying them is essential to implementing effective data quality detection and fixing strategies. With the right tools, processes, and governance frameworks in place, organizations can ensure that their data is accurate, reliable, and fit for purpose.
You might also like
Get in Touch to Learn More
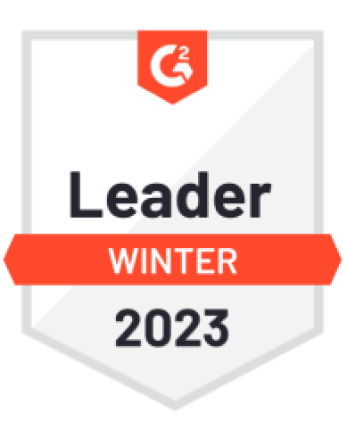
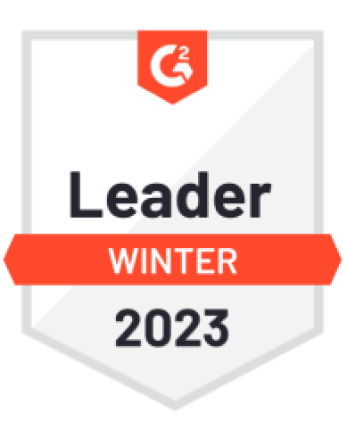

“[I like] The easy to use interface and the speed of finding the relevant assets that you're looking for in your database. I also really enjoy the score given to each table, [which] lets you prioritize the results of your queries by how often certain data is used.” - Michal P., Head of Data