Databricks Metadata Management: FAQs, Tools, Getting Started
Learn everything you need to know about Databricks metadata management in this comprehensive article.

Metadata management is a crucial part of working with data in Databricks. In this article, we'll explore what Databricks Metadata Management is all about, why it's important, and how you can get started. We'll also cover some common questions, useful tools, and even look at what's coming up in the future of metadata management. Let's dive in!
Understanding Databricks Metadata Management
Metadata management is like keeping a detailed catalog of all your data. In Databricks, it's about organizing information about your data assets, such as tables, files, and workflows. By automating metadata management processes, businesses can achieve significant cost and time savings.
Defining Databricks Metadata Management
Databricks Metadata Management is the process of creating and maintaining a central repository of information about your data. It's like builing a library catalog for your data, keeping track of what you have, where it came from, and how it's changed over time. This ensures data governance policies are consistently enforced, improving data lineage and discovery.
Importance of Metadata Management in Databricks
Good metadata management in Databricks is crucial for several reasons:
- It helps you find the data you need quickly.
- It ensures your data is reliable and high-quality.
- It keeps you compliant with data regulations.
- It makes collaboration easier and allows for data reuse.
- It improves data security by tracking who's accessing and modifying data.
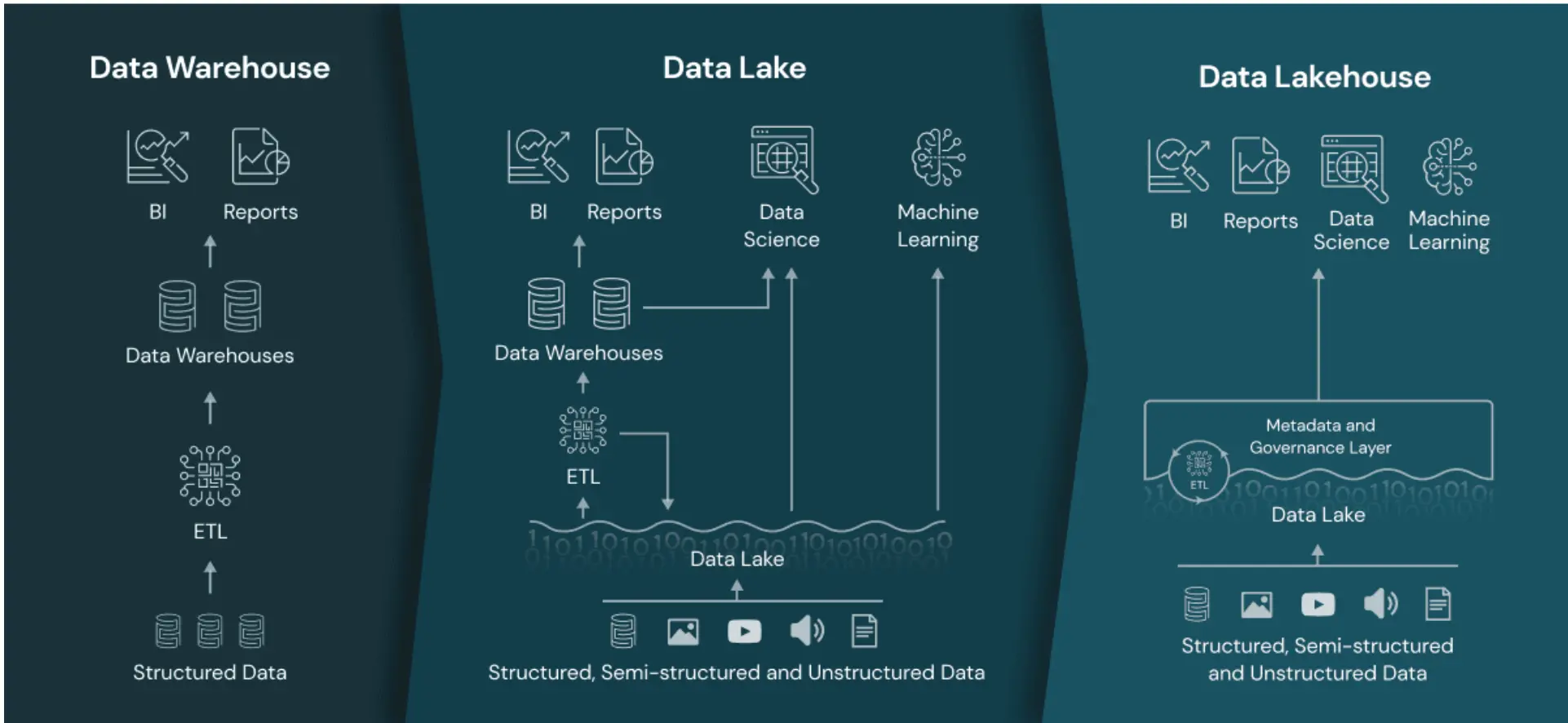
Frequently Asked Questions about Databricks Metadata Management
What is Databricks Metadata Management?
Databricks Metadata Management is about creating a map of your data landscape. It involves tracking where your data comes from, how it's structured, and how it's used. This helps you understand and manage your data more effectively.
Why is Metadata Management Important in Databricks?
Metadata management is like having a well-organized toolbox for your data. It helps you find what you need quickly, ensures your data is in good shape, and helps you follow data governance rules. It's particularly important if you're dealing with sensitive data or working in heavily regulated industries. With regulations like GDPR and CCPA becoming increasingly stringent, automation aids businesses in staying compliant.
How Does Databricks Handle Metadata Management?
Databricks comes with built-in features for metadata management. It has a workspace for organizing your assets, and a catalog for keeping track of your datasets and tables. It also integrates with external metadata tools, allowing you to extend your metadata management capabilities.
Exploring Tools for Databricks Metadata Management
Overview of Tools for Metadata Management
Databricks offers several tools to help with metadata management:
- Databricks Workspace: This is your central hub for organizing projects and collaborating with your team.
- Databricks Delta: This is an advanced data management system that helps keep your data organized and up-to-date.
- Databricks catalog: This serves as your data directory, helping you find and manage your datasets and tables.

Selecting the Right Tools for Your Needs
Choosing the right tools depends on your specific requirements. For basic needs, the Workspace and catalog might be sufficient. For more complex data environments, you might want to incorporate Delta. Consider your data volume, complexity, and integration needs when making your choice.
Getting Started with Databricks Metadata Management
Preparing for Metadata Management
Before diving in, it's good to do some groundwork:
- Define your metadata management goals.
- Identify key stakeholders and their roles.
- Determine the scope of your metadata management initiative.
- Establish data governance policies.
- Define the metadata attributes you want to capture.
- Ensure you have the necessary infrastructure and resources.
Implementing Databricks Metadata Management
Once you're ready to start:
- Configure your Databricks catalog.
- Use tags and labels to enhance data discoverability.
- Leverage data lineage capabilities to track data flow.
- Regularly update and maintain your metadata repository.
Best Practices for Databricks Metadata Management
Here are some tips for successful metadata management:
- Establish clear naming conventions.
- Regularly review and update metadata attributes.
- Implement access controls and permissions.
- Encourage collaboration and knowledge sharing.
- Monitor and track metadata usage.
Advanced Topics in Databricks Metadata Management
Optimizing Your Metadata Management Strategy
As you become more proficient, look for ways to fine-tune your approach. Consider automating tasks, using advanced analytics for insights, and measuring the impact of your metadata management efforts.
Troubleshooting Common Issues in Metadata Management
You might encounter challenges like inconsistent metadata or data duplication. Stay proactive by assigning data stewardship roles, implementing data quality checks, and regularly auditing and cleaning up your data.
Future Trends in Databricks Metadata Management
Keep an eye on emerging trends like AI-powered metadata management, integration with data governance tools, and metadata-driven analytics platforms. Staying informed about these developments can help you make the most of your metadata management efforts.
Effective metadata management is key to unlocking the full potential of your data in Databricks. By understanding what it is, why it matters, and how to implement it effectively, you can ensure your data is high-quality, well-governed, and easy to use.
Conclusion
Now that you understand the importance of metadata management in Databricks, take your data strategy to the next level with CastorDoc. Our AI assistant is powered by a robust Data Catalog, leveraging the very metadata principles we've discussed to provide accurate and nuanced answers to your data questions. CastorDoc seamlessly integrates advanced governance, cataloging, and lineage capabilities - crucial aspects of effective metadata management - with a user-friendly data assistant. This combination creates a powerful tool for enabling self-service analytics, making it easier than ever to find, understand, and use your data assets. Don't just manage your metadata - put it to work for you.
Transform your Databricks metadata into actionable insights and business decisions with CastorDoc. Ready to unlock the full potential of your data? Try CastorDoc today and experience the power of intelligent metadata management.
You might also like
Get in Touch to Learn More
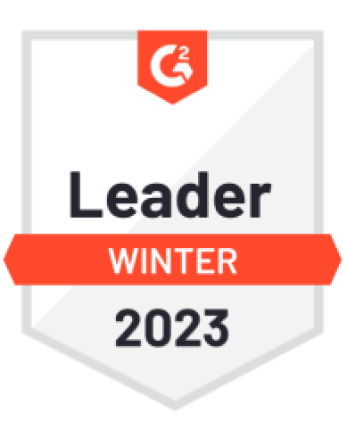
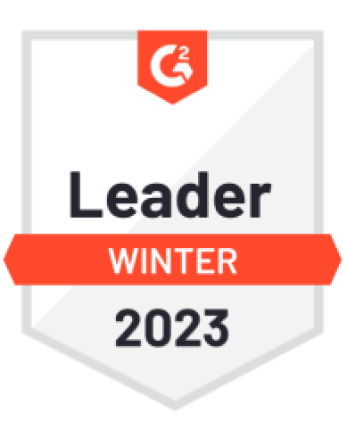

“[I like] The easy to use interface and the speed of finding the relevant assets that you're looking for in your database. I also really enjoy the score given to each table, [which] lets you prioritize the results of your queries by how often certain data is used.” - Michal P., Head of Data