Data Observability Tool Comparison: Lantern vs. Marquez
In the world of data management, ensuring the observability of data is crucial for maintaining the integrity and reliability of data pipelines. Two popular tools that facilitate data observability are Lantern and Marquez. In this article, we will dive into the features, pros, and cons of these tools, and ultimately compare and contrast their capabilities. By the end, you will have a clear understanding of which tool is best suited for your specific data observability needs.
Understanding Data Observability
Data observability refers to the ability to gain insights and monitor various aspects of data within a system. It encompasses three critical components: data quality, data freshness, and data lineage. By having a robust data observability tool, organizations can ensure the accuracy and timeliness of data, track its origin and transformations, and identify and resolve any issues that may arise.
The Importance of Data Observability
Data lies at the heart of every organization, and ensuring its observability is paramount. Data observability enables companies to make informed decisions, identify and resolve issues quickly, and maintain trust in the data-driven insights they generate. Without proper observability, data pipelines can become riddled with inaccuracies, leading to flawed analyses, inefficient operations, and potentially severe consequences for the business.
Key Components of Data Observability
When evaluating data observability tools like Lantern and Marquez, it is essential to consider the key components that make up a comprehensive solution. These components include:
- Data Quality Monitoring: A reliable tool should provide visibility into the quality of data by monitoring metrics such as completeness, accuracy, consistency, and validity.
- Data Freshness: Tracking the timeliness of data is of utmost importance. Tools should allow monitoring of data latency, ensuring that data is up-to-date.
- Data Lineage: The ability to track data lineage is crucial for understanding the origin, flow, and transformations that data undergoes within a system.
Let's delve deeper into each of these components to understand their significance:
Data Quality Monitoring: Ensuring the quality of data is essential for making reliable business decisions. Data quality monitoring tools enable organizations to identify and rectify any issues that may impact the accuracy and completeness of their data. By monitoring metrics such as completeness, accuracy, consistency, and validity, organizations can maintain data integrity and trust in their insights.
Data Freshness: The timeliness of data is crucial for organizations to stay agile and responsive in today's fast-paced business environment. Data freshness refers to the ability to track the latency of data, ensuring that it is up-to-date. By monitoring data freshness, organizations can identify any delays in data processing and take corrective actions to ensure real-time insights and decision-making.
Data Lineage: Understanding the journey of data from its origin to its current state is vital for data-driven organizations. Data lineage tools provide visibility into the flow and transformations that data undergoes within a system. This information helps organizations trace back any issues or discrepancies in their data, ensuring data accuracy and compliance.
By leveraging comprehensive data observability tools that encompass these key components, organizations can establish a solid foundation for their data-driven operations. These tools enable them to gain valuable insights, maintain data integrity, and make informed decisions that drive business growth and success.
Introduction to Lantern
Lantern is a popular data observability tool that offers a range of features aimed at ensuring the integrity and reliability of data pipelines. Let's explore some of its core features below.
Lantern is designed to provide users with a comprehensive solution for monitoring and optimizing data pipelines. By offering a suite of features that focus on data quality, freshness, and lineage, Lantern empowers organizations to make informed decisions based on reliable and up-to-date data.
Core Features of Lantern
Lantern provides comprehensive data observability by offering the following features:
- Data Quality Monitoring: Lantern enables users to define and track custom data quality metrics, ensuring the accuracy and completeness of data.
- Data Freshness Monitoring: The tool allows users to monitor data latency, ensuring that data is up-to-date and meets desired timeliness requirements.
- Data Lineage Visualization: Lantern visualizes data lineage, enabling users to understand the flow and transformations of data within a system.
Moreover, Lantern goes beyond basic monitoring by providing proactive alerts and notifications to users when data quality or freshness thresholds are not met. This real-time feedback loop allows teams to address issues promptly and maintain the reliability of their data pipelines.
Pros and Cons of Using Lantern
Like any tool, Lantern has its advantages and disadvantages. Let's explore these in more detail:
- Pros:
- Easy to set up and use, even for non-technical users.
- Provides a comprehensive set of features for data observability.
- Offers intuitive visualizations for better understanding of data lineage.
- Cons:
- May have limitations in terms of scalability for very large datasets.
- Does not offer advanced data anomaly detection capabilities.
Introduction to Marquez
Marquez is another data observability tool that aims to provide visibility and control over data pipelines. Let's delve deeper into the world of Marquez to uncover its intricacies and functionalities.
Founded on the principles of enhancing data observability, Marquez stands out as a robust platform designed to empower users with insights into their data ecosystem. By offering a comprehensive suite of features, Marquez enables organizations to optimize their data workflows and drive informed decision-making processes.
Core Features of Marquez
Marquez offers a rich tapestry of features that elevate the data observability experience:
- Data Quality Monitoring: Marquez empowers users to establish and track data quality metrics, ensuring the integrity and trustworthiness of their data assets.
- Data Freshness Monitoring: With Marquez, users can meticulously monitor data latency, guaranteeing that their data remains current and relevant for analytical purposes.
- Data Lineage Visualization: Through intuitive visualizations, Marquez illuminates the intricate web of data lineage, enabling users to trace data origins, transformations, and destinations with ease.
- Metadata Management: Marquez goes beyond lineage tracking by offering robust metadata management capabilities, allowing users to enrich their data assets with contextual information for improved understanding and governance.
Pros and Cons of Using Marquez
Let's weigh the advantages and limitations of incorporating Marquez into your data observability toolkit:
- Pros:
- Boasts a user-friendly interface that simplifies adoption and usage for data professionals across various skill levels.
- Delivers comprehensive data lineage tracking, empowering users with a holistic view of data flow and dependencies within their pipelines.
- Supports multiple programming languages, enhancing flexibility and interoperability for diverse data pipeline environments.
- Cons:
- May lack some advanced features present in more mature data observability tools, potentially limiting the depth of analysis and monitoring capabilities.
- As a relatively newer entrant in the market, Marquez may have a smaller user base compared to established industry players, which could impact community support and resources.
In-depth Comparison
Now, let's delve even deeper into the comparison between Lantern and Marquez, exploring various facets of their functionality to provide a comprehensive analysis.
One crucial aspect to consider when evaluating these two tools is their integration capabilities. Lantern excels in seamless integration with a wide range of data sources, making it ideal for organizations dealing with diverse data formats. On the other hand, Marquez boasts robust integration features that enable users to connect with various data storage systems effortlessly, ensuring smooth data flow across different platforms.
User Interface Comparison
Both Lantern and Marquez offer user-friendly interfaces that facilitate intuitive interactions with the tools. While Lantern's interface is celebrated for its simplicity and user-friendliness, catering to users of all technical backgrounds, Marquez takes it a step further by providing a highly customizable interface. This customization empowers users to personalize their workspace according to their unique preferences and workflow, enhancing overall user experience.
Performance and Speed Comparison
When evaluating performance and speed, Lantern shines in handling substantial data volumes efficiently, ensuring swift processing and analysis. However, it is essential to note that when dealing with exceptionally large datasets, Lantern may encounter scalability challenges. In contrast, Marquez is engineered with scalability in mind, capable of seamlessly managing massive data volumes without compromising on performance or speed, making it a reliable choice for demanding data processing tasks.
Scalability Comparison
Both Lantern and Marquez offer scalable solutions to accommodate varying data processing needs. Nevertheless, Marquez stands out in handling intricate and dynamic data pipelines with ease, making it a preferred option for organizations navigating complex data environments that evolve rapidly over time. Its scalability features ensure that organizations can efficiently scale their data operations as their needs grow, maintaining optimal performance and reliability.
Pricing Structure
Understanding the pricing details of Lantern and Marquez is essential to make an informed decision.
When it comes to Lantern's pricing structure, they offer a subscription-based model that caters to a wide range of users. The tiers are designed to accommodate varying sizes and complexities of data pipelines, ensuring that users can choose a plan that aligns with their specific needs. Starting at $X per month for smaller-scale usage, Lantern's pricing scales up gradually to cater to enterprise-grade deployments that require more robust features and support.
On the other hand, Marquez adopts a usage-based pricing approach that provides flexibility for users with diverse data processing requirements. With Marquez, users are billed based on the number of data pipelines they utilize and the volume of data processed through these pipelines. This pay-as-you-go model allows for cost efficiency, as users only pay for the resources they consume. The pricing for Marquez starts at $X per pipeline, with incremental increases based on the amount of data processed.
Lantern Pricing Details
Lantern offers a subscription-based pricing model with different tiers based on the size and complexity of the data pipelines. The pricing starts at $X per month for small-scale usage and can go up to $X per month for enterprise-grade deployments.
Marquez Pricing Details
Marquez follows a usage-based pricing model. Users are billed based on the number of data pipelines and the volume of data processed. The pricing starts at $X per pipeline and increases based on the volume of data processed.
In conclusion, both Lantern and Marquez offer robust solutions for data observability. Choosing between the two depends on specific requirements such as ease of use, scalability, performance, and pricing considerations. By understanding the features, pros, and cons of both tools, you can make an informed decision that best aligns with your organization's data observability needs.
While Lantern and Marquez offer valuable features for data observability, it's essential to explore solutions that integrate advanced governance with ease of use. CastorDoc stands out by combining a robust data catalog with an AI assistant, streamlining self-service analytics for both data teams and business users. With CastorDoc, you gain comprehensive control over data governance, cataloging, lineage, and quality assurance, all through a user-friendly conversational interface. Whether you're managing data compliance or seeking to empower business decisions, CastorDoc's intuitive platform is designed to elevate your data management capabilities. To discover how CastorDoc compares to other tools and how it can transform your data governance and analytics, check out more tools comparisons here.
You might also like
Get in Touch to Learn More
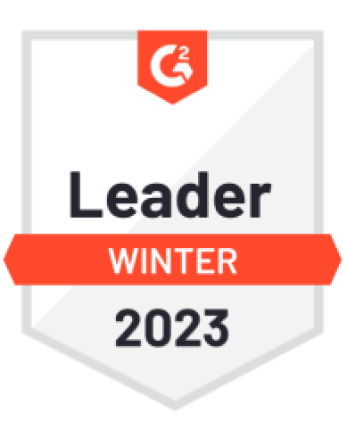
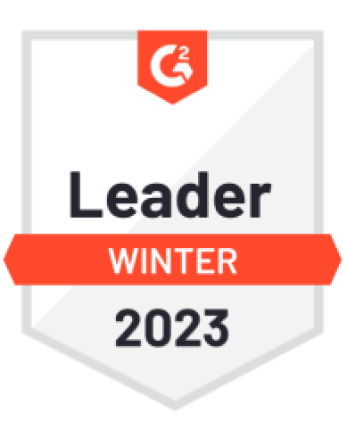

“[I like] The easy to use interface and the speed of finding the relevant assets that you're looking for in your database. I also really enjoy the score given to each table, [which] lets you prioritize the results of your queries by how often certain data is used.” - Michal P., Head of Data